Taming the Wild West of Data Governance
Bringing Order to the Chaos
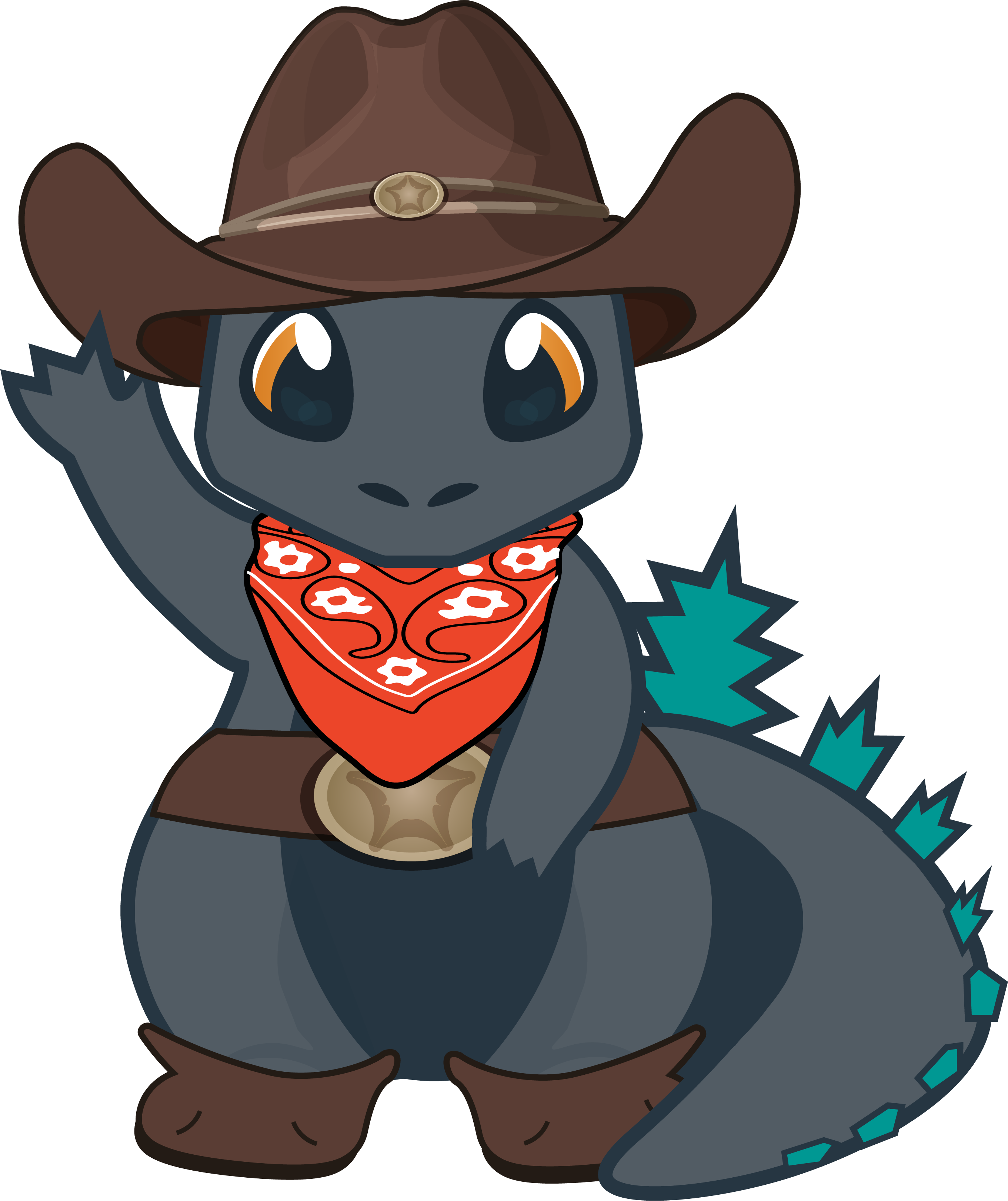
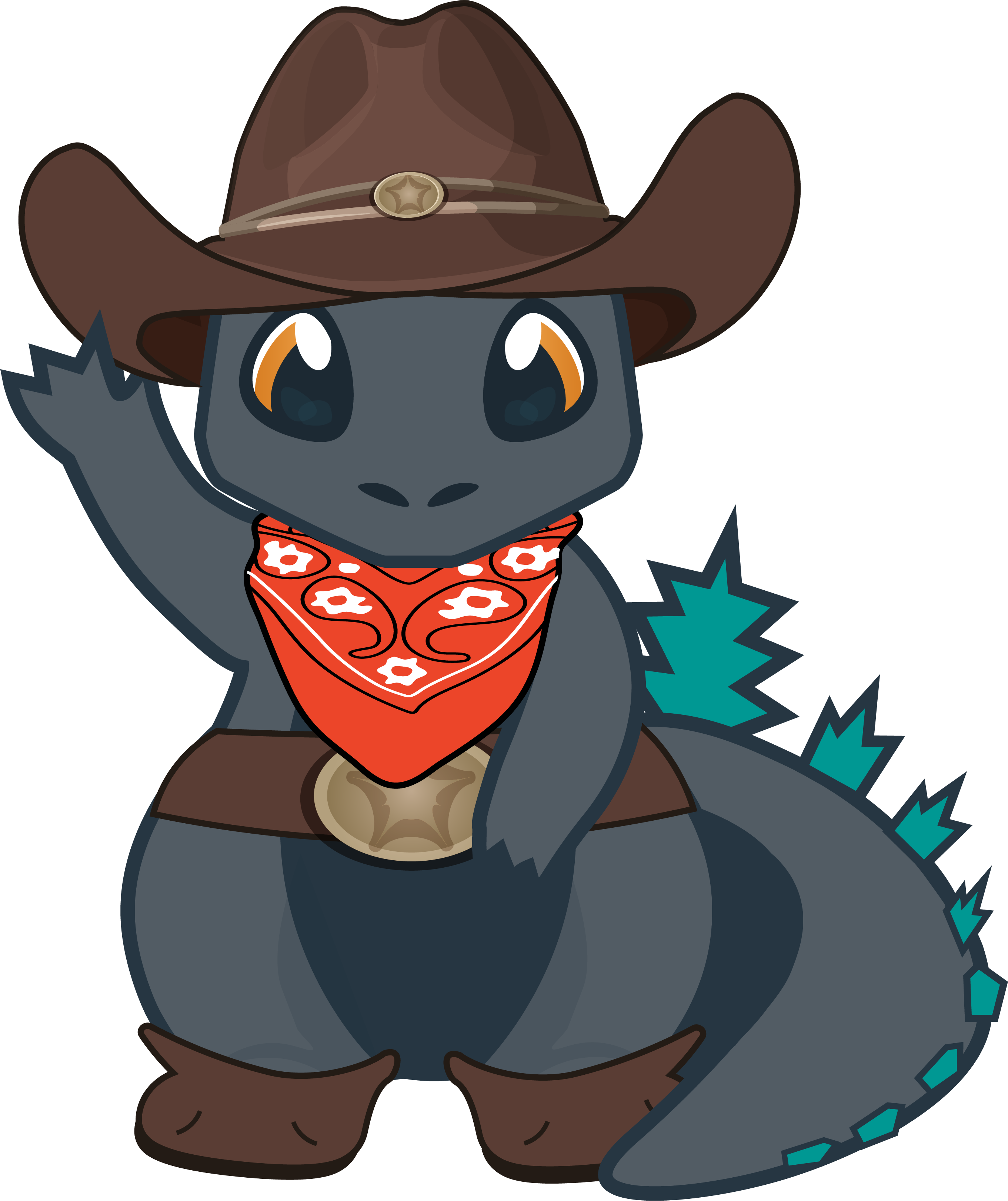
Taming the Wild West of Data Governance
Bringing Order to the Chaos
– Frank Ricotta, CEO & Founder, BurstIQ
In the days of yore, the Wild West was a lawless land – a chaotic mix of prospectors, bandits, and dreamers all vying for a piece of the gold rush. Today’s data landscape feels eerily similar. Data flows like a digital river, surging through countless disconnected systems, departments, and partners. It’s a land of:
In the days of yore, the Wild West was a lawless land – a chaotic mix of prospectors, bandits, and dreamers all vying for a piece of the gold rush. Today’s data landscape feels eerily similar. Data flows like a digital river, surging through countless disconnected systems, departments, and partners. It’s a land of:
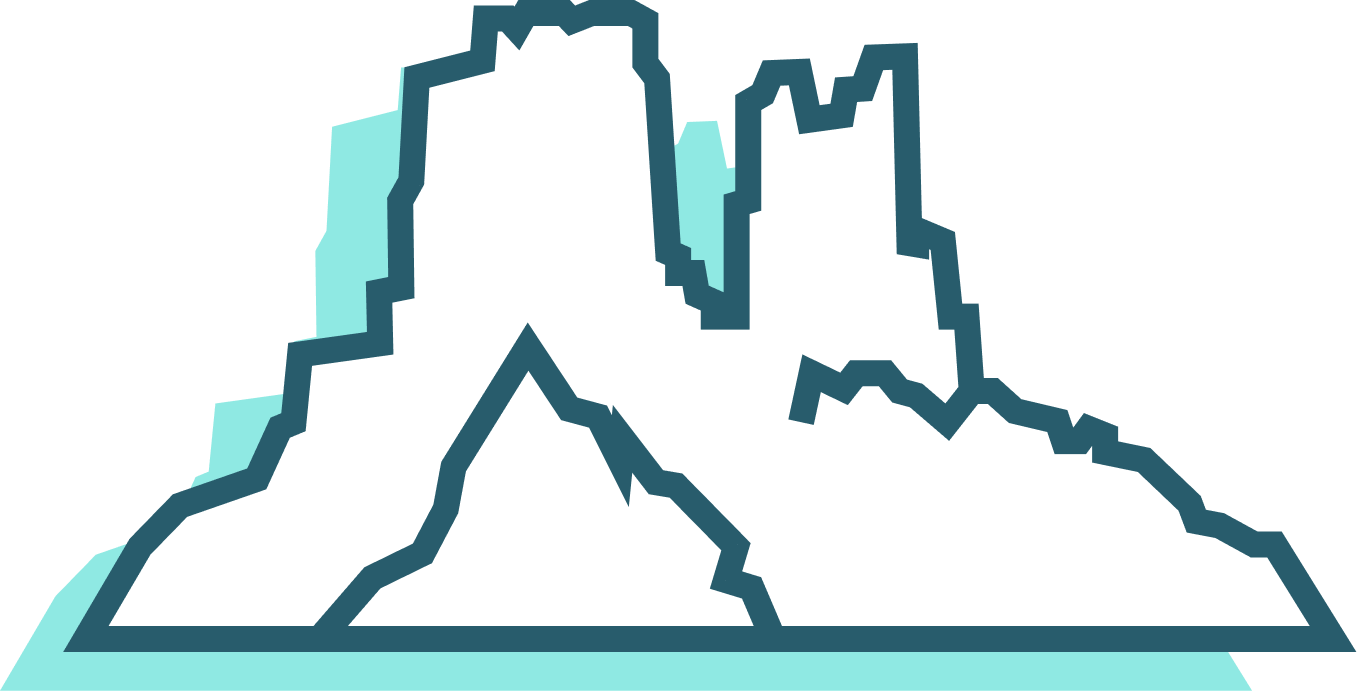
Silos as Far as the Eye Can See:
Hundreds of data sources sprawl, each holding a nugget of valuable information but lacking a central registry.
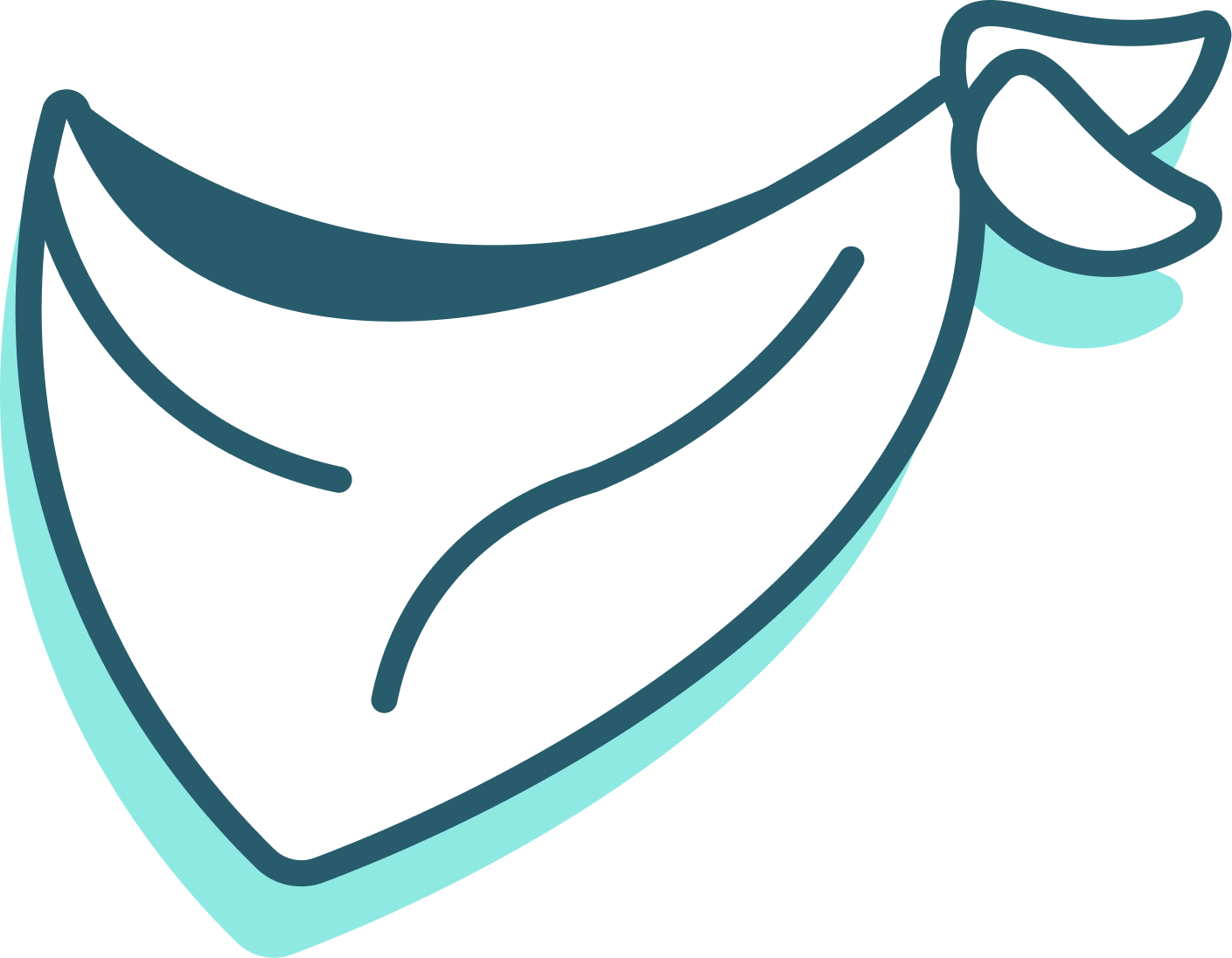
Data Definition Outlaws:
Every team interprets data differently, leading to inconsistencies and confusion.
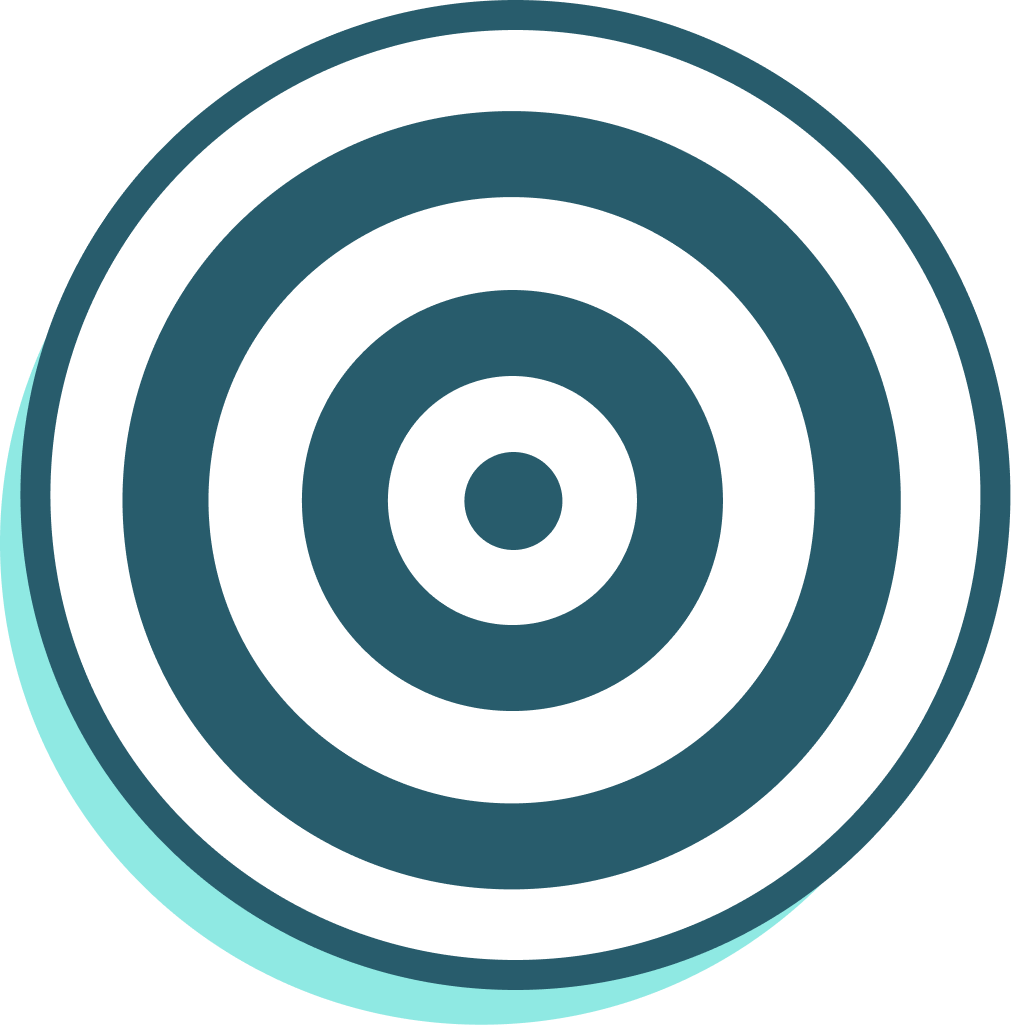
Security Shootouts:
Vulnerabilities between systems create conditions ripe for data breaches.
Just like the Wild West needed a sheriff to bring order, organizations need a new approach to data governance. At BurstIQ, we’re wrangling this digital frontier with innovative technology to calm the chaos.
However, providing some context and definition for data governance is appropriate to set the stage for this discussion.
Data governance establishes who manages and protects data assets across their lifecycle. This includes setting policies for data security, storage, retention, privacy, quality, and usage. IT and business teams have key strategic planning, implementation, and oversight roles.
Formal data governance helps reduce risks related to compliance, decision-making with poor-quality data, and unauthorized access or usage. It also enables tapping into data’s total value by discovering, understanding, and connecting relevant data across silos.
Data governance aims to establish a solid foundation for data management, enabling organizations to make informed decisions based on reliable and trusted data.
Overall, this is a noble goal. However, most organizations fall short due to various factors.
Challenges of Data Governance in Traditional Enterprise Data Solutions
Typical enterprise IT landscapes have hundreds of disconnected systems storing data in siloed departments. The problem continues to grow exponentially; the more data an organization generate, the more data an organization needs, the more silos emerge. In many ways, building barriers around a silo and controlling that data is viewed as a means of power and influence.
During a recent call with an industry analyst, they conveyed that most established and even successful businesses have realized the need to modernize their legacy data infrastructures but need a clear path forward without totally disrupting the business.
As a result, it becomes difficult to have a unified view of data across the organization, limiting the exponential impact on the business and hiding a hidden store of value, leading to significant challenges:
- Data definitions, rules, and quality metrics are inconsistent across systems
- Manual processes to request access, report issues, or make changes
- Security gaps from porous borders between systems
- There is no way to get organization-wide visibility or reuse data
- Costly data integration and master data management projects using legacy tools provide limited relief
Data quality issues can arise without proper governance, leading to inconsistencies and inaccuracies. We will explore data quality in future Knowledge Bursts. There is an underlying debate about quality vs. quantity. The former pushes for standards when ingesting into a larger data repository, while the latter promotes quality as a byproduct of computational integrity and system intelligence.
New Frontiers on the Horizon:
The Challenges of Web3, Regulations, & Partner Ecosystems
The advent of web3, partner ecosystems, government privacy regulations, and other challenges add complexity to data governance:
- Web3 & Decentralization: Decentralized technologies like blockchain introduce new governance challenges, such as managing distributed data and ensuring privacy while maintaining transparency.
- Partner Ecosystems: Collaborating with partners and leveraging third-party data sources increases the need for effective governance across organizational boundaries.
- Privacy Regulations: Government regulations like the General Data Protection Regulation (GDPR) and the California Consumer Privacy Act (CCPA) require organizations to implement robust data governance practices to protect individual privacy rights.
- Emerging Challenges: Rapid advancements in technologies like Artificial Intelligence (AI) and the Internet of Things (IoT) bring new data governance challenges related to bias, ethics, and accountability.
These require rethinking data governance to work effectively beyond the firewall.
Active Metadata:
The Gunslinger of Data Governance
Active metadata plays a crucial role in enhancing data governance practices. Active metadata is dynamic and interactive metadata, providing more than just descriptive information about data but also actively participates in the management, organization, and processing of that data. Unlike traditional static metadata, which typically remains unchanged once created, active metadata can be modified, updated, or enriched in real time based on various factors or events.
- Automated Data Lineage: Active metadata tracks data lineage throughout its lifecycle, providing insights into data origin, transformations, and usage.
- Embedded Policies: Metadata can include embedded data quality, security, and compliance policies, ensuring that data adheres to governance standards.
- Real-time Alerts: Active metadata can trigger real-time alerts when data violates governance policies, allowing for proactive remediation.
- AI-driven Recommendations: With active metadata, AI algorithms can recommend adding or updating metadata based on data usage patterns and context.
Active metadata empowers organizations to have a more comprehensive and automated approach to data governance. This “self-describing” data approach federates governance to improve agility, reduce risk, and ease regulatory needs.
Taming the Data Trail:
Data Fabrics & Knowledge Graphs Upgrade Data Infrastructures
Data fabrics and knowledge graphs offer innovative approaches to upgrade existing data infrastructures without needing a disruptive overhaul and promise to transform enterprise data estates. I’ve written extensively about both in previous blogs (How a Web3 Data Fabric Can Help You Leapfrog the Market, Knowledge Graphs: Access, Manage, Share, and Derive Insights From Data Like Never Before)
To summarize:
- Data Fabrics: Data fabrics enable the integration and optimization of data assets by providing a unified and virtualized layer that connects disparate data sources. Organizations can access and govern data more efficiently without complex data integration projects.
- Knowledge Graphs: Knowledge graphs capture relationships between data entities, enabling more contextual data discovery, intelligent querying, and recommendations. They enhance data governance by providing a holistic view of data relationships and dependencies.
Data fabrics and knowledge graphs can improve data accessibility, governance, and analytics capabilities, leading to better insights and decision-making. These can overlay governance, semantics, context, and linkages onto existing infrastructure to unlock more value. Global active metadata models can enforce policies and quality across federated sources.
Wrangling Data with AI, GenAI, & LLMs
AI and ML are instrumental in automating governance and introducing new challenges. Responsible governance applies to AI systems as well:
- Natural language interfaces (LLMs) enable more conventional data searches
- Automated profiling of data sets for quality, risk, and bias indicators
- Recommenders for appropriate data access and usage authorization
- Automated metadata recommendations and life cycle management
But models need transparency, ethical oversight, and bias detection as well. Emerging generative AI shows promise for more complex data-related tasks, such as automatic knowledge graph creation and augmenting data scenarios.
Eureka! A Civilized Data Landscape
When implementing a new data governance solution, it is crucial to measure key return on investment (ROI) metrics, such as:
- Time-to-Value: Accelerated onboarding of new data sources and reduced time spent on manual governance tasks.
- Data Accessibility & Reuse: Increased availability and usability of data across the organization, leading to improved efficiency and productivity.
- Data Quality & Trust: Measurable data quality improvements result in more reliable insights and decision-making.
- Compliance & Risk Mitigation: Demonstrable adherence to privacy regulations and reduced data breaches or non-compliance risks.
- Innovation Acceleration: The ability to leverage data as a strategic asset to drive innovation and gain a competitive edge.
These ROI measurement points help organizations assess the effectiveness and impact of their data governance initiatives.
From Data Droughts to the Data Rush:
Staking Your Claim in the New Data Territory
The transition from data scarcity to data abundance has reshaped the data landscape:
- Data Scarcity Era: In the past, organizations struggled to gather enough data to make informed decisions. Data was often scarce, controlled, and owned by individual entities.
- Data Abundance Era: With the proliferation of digital technologies and the rise of interconnected ecosystems, there is now an abundance of data available from various sources, including partners and customers. Data can be easily accessed and integrated, enabling organizations to tap into new insights and opportunities.
This shift necessitates a new approach to data governance that embraces openness, interoperability, and automation. Organizations must rethink their governance strategies to effectively manage and leverage the vast data available in the plug-and-play data ecosystem.
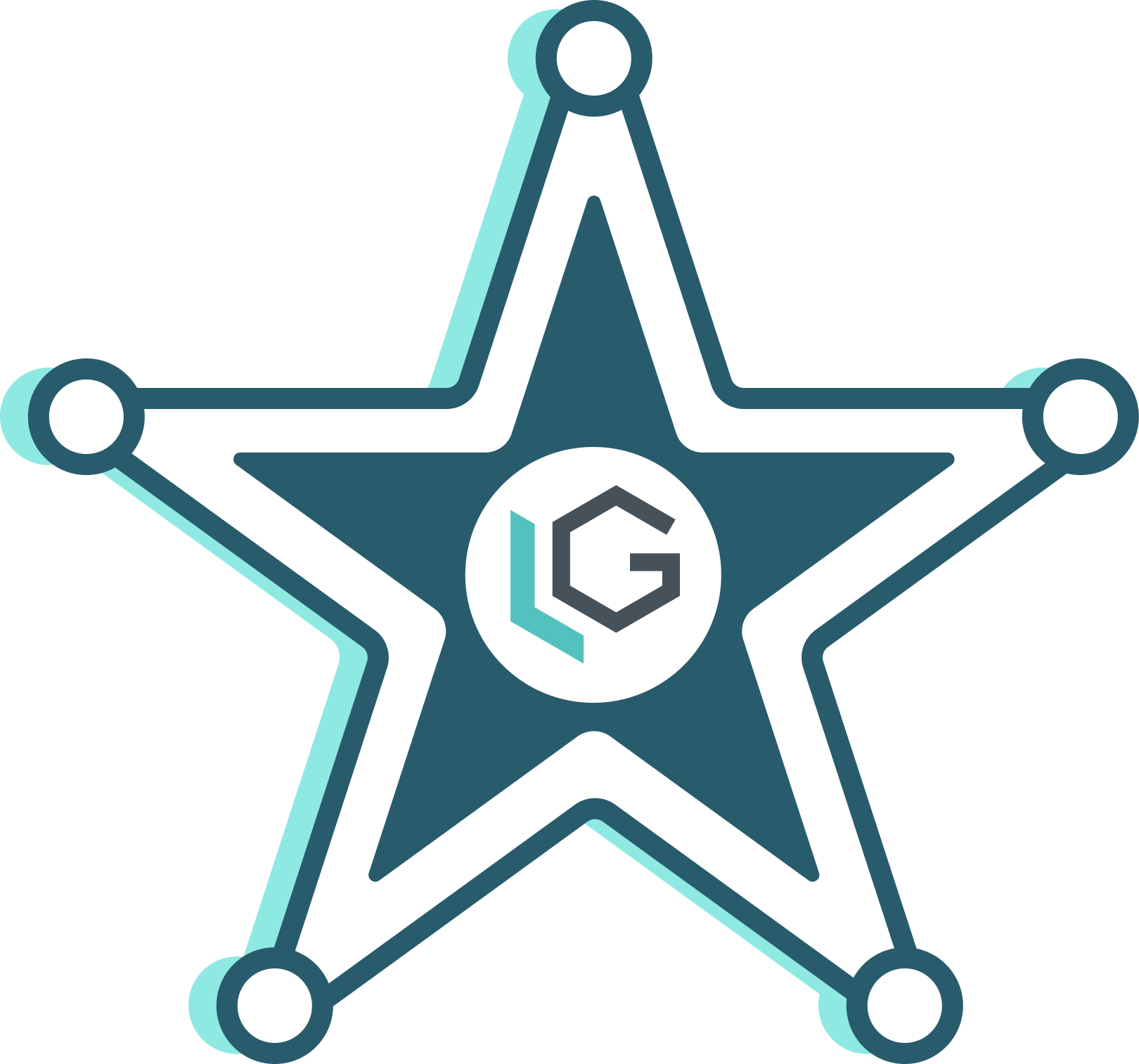
LifeGraph:
The New Sheriff in Town
BurstIQ’s LifeGraph® Platform corrals a unique blend of technologies not found in legacy data management solutions. With API-powered data integration, active metadata, privacy-enhancing technology, smart contracts, and knowledge graphs, the platform is truly a one-stop shop for holistic and dynamic data governance. LifeGraph seamlessly integrates with your current data infrastructure, instilling order amidst your existing data landscape while accommodating the daily influx of new data.
Conclusion
In conclusion, data governance’s wild wild west presents challenges and hidden opportunities. By understanding these challenges, adopting new technologies, and implementing effective data governance practices, organizations can navigate this ever-evolving landscape and unlock the full potential of their data assets.
Are you interested in taming data governance at your organization?
Let’s connect to discuss the possibilities.
About BurstIQ:
BurstIQ’s LifeGraph is an advanced data management platform that enables secure, transparent, and efficient data management for businesses across various industries. By harnessing the power of blockchain technology, BurstIQ empowers organizations to quickly integrate data from any source so they can create a trustworthy business ecosystem. The platform applies knowledge graphs to make data more accessible and to deliver insights that optimize processes, reduce risk, and personalize customer solutions across their ecosystems. With a strong focus on innovation and customer-centricity, BurstIQ continues revolutionizing industries and setting new standards in enterprise blockchain-powered Web3 solutions.
Safeguarding
Intellectual Property
in an AI-Powered World
– Frank Ricotta, CEO & Founder, BurstIQ
As artificial intelligence continues its relentless march into nearly every industry and business function, crucial questions arise regarding protecting proprietary innovations, data, and other intellectual capital in an AI-driven landscape. If AI uses another person’s or company’s data to generate a new innovation, who owns the innovation – the owner of the source data, the creator of the AI, or the AI itself? With AI poised to become businesses’ most valuable asset and competitive differentiator, implementing robust IP protections is more critical than ever.
What does IP Protection entail with AI?
Confidential Data Protection
First and foremost, organizations must keep focus on implementing stringent policies, protocols, and security measures to prevent the leakage of sensitive internal data like customer information, financial records, strategic plans, research, and other trade secrets that power AI systems. As organizations become highly data-centric, vigilant data governance and traceable access controls are essential to lock down intellectual capital and maintain competitive edges or to track the source of a breach or leak in order to fortify and protect against future attacks.
Assigning Ownership Rights to AI Output
Second, with creative AI now composing music, generating art, designing products, writing software, and even inventing novel solutions, questions about legal rights and ownership of AI’s autonomous output arise. Who owns the IP rights if AI systems create songs, products, or processes with little to no human involvement? Should the AI platform itself enjoy legal protections? Does IP belong to the creators of the underlying algorithms or to the data owner or the AI user who prompts the output? Resolving these issues will grow in importance as AI’s creative prowess expands.
Tracking Attribution in Collaborative Efforts
Finally, collaborative human-AI projects introduce additional complexity in properly assigning ownership and quantifying individual contributions when breakthroughs emerge. Implementing immutable data provenance trails and contribution-tracking systems to follow IP lineage across these entangled partnerships is necessary. Doing so will help untangle knotty attribution questions when valuable innovations result.
Thankfully, there are promising methods to balance IP protections and responsible innovation. One such method is federated learning. Federated learning enables the use of sensitive data to train models without moving data off-site, averting confidentiality pitfalls.
- Encryption, access controls, and data masking preserve data security and privacy when leveraging cloud services. Privacy-preserving computation methods like homomorphic encryption, secure multi-party computation, and trusted execution environments allow sensitive data to be analyzed without exposing raw datasets.
- Powerful anonymization, differential privacy, and synthetic data generation techniques derive valuable insights from data while preventing reconstruction of sensitive personal information.
- Compartmentalization walls off proprietary algorithms in development, only combining components at the last stage before deployment to prevent IP leakage.
- Granular access policies on model usage, controls on external hosting, and model watermarking – essentially injecting data tracking into the data itself – help maintain control even once models are deployed.
- Formal verification methods mathematically prove code and model properties, preventing extraction of proprietary logic while still allowing external validation.
- Blockchain-based data provenance platforms create immutable asset ownership records and contributor identities across collaborations. Detailed lineage tracking on model development data, code, testing, and deployment provides audit trails demonstrating IP ownership.
- Stringent data governance policies, finely tuned access controls, and data usage auditing help regulate internal datasets, allowing AI to maintain confidentiality while permitting controlled analytics usage.
The LifeGraph® platform from BurstIQ provides a Web3/blockchain based secure and scalable data fabric supporting AI adoption, ensuring data integrity, governance, and security while maintaining regulatory compliance. Its distributed data structure safeguards IP, fostering trust and transparency in data transactions. Active metadata builds data confidence by offering insights into data’s origin, quality, and usage, promoting informed decisions. Ownership and consent controls, powered by smart contracts, protect IP in both data and data models, ensuring data usage is governed by explicit consent. Anonymization and tokenization techniques safeguard sensitive information, reducing the risk of IP theft. Additionally, LifeGraph incentivizes data sharing and monetization, helping companies expand their ecosystem and generate revenue while maintaining control over their intellectual property. It’s everything you need to protect your organization and accelerate your AI journey in a single platform.
If you’re ready to unlock AI’s immense innovation potential, it’s important to do so with care and foresight so you can rigorously safeguard your most valuable IP assets and data. As AI capabilities grow more profound, developing comprehensive IP protection policies, advanced security protocols, and attribution tracking systems will only become more critical.
Struggling with Where to Start with AI?
We’d love to talk to you about your company’s entry into the AI arena and schedule a collaboration session to share our expertise and ensure you’re set up for success from the very beginning. Our customers find this complimentary strategy session extremely useful for plotting their course for AI success. If you’re interested, connect with us to schedule your session.
About BurstIQ:
LifeGraph® by BurstIQ redefines the potential of organizational data. This next-generation data platform integrates advanced data management, privacy-enhancing technology, and knowledge graphs, transforming data into your organization’s ultimate superpower. Eliminate silos with a single, secure source of truth. LifeGraph reveals hidden connections within complex data sets, aligning with human and machine thinking for easier and more insightful analysis and powerful collaboration.
Organizations use LifeGraph to elevate legacy data lakes and warehouses into dynamic, secure, and person-centric data ecosystems that deliver value to everyone involved. With LifeGraph you can quickly address today’s problems and business initiatives, and ignite the spark of innovation to help your organization not only keep pace but set the tempo for the future.
To learn more about how LifeGraph can help you make data your superpower, please contact us here.