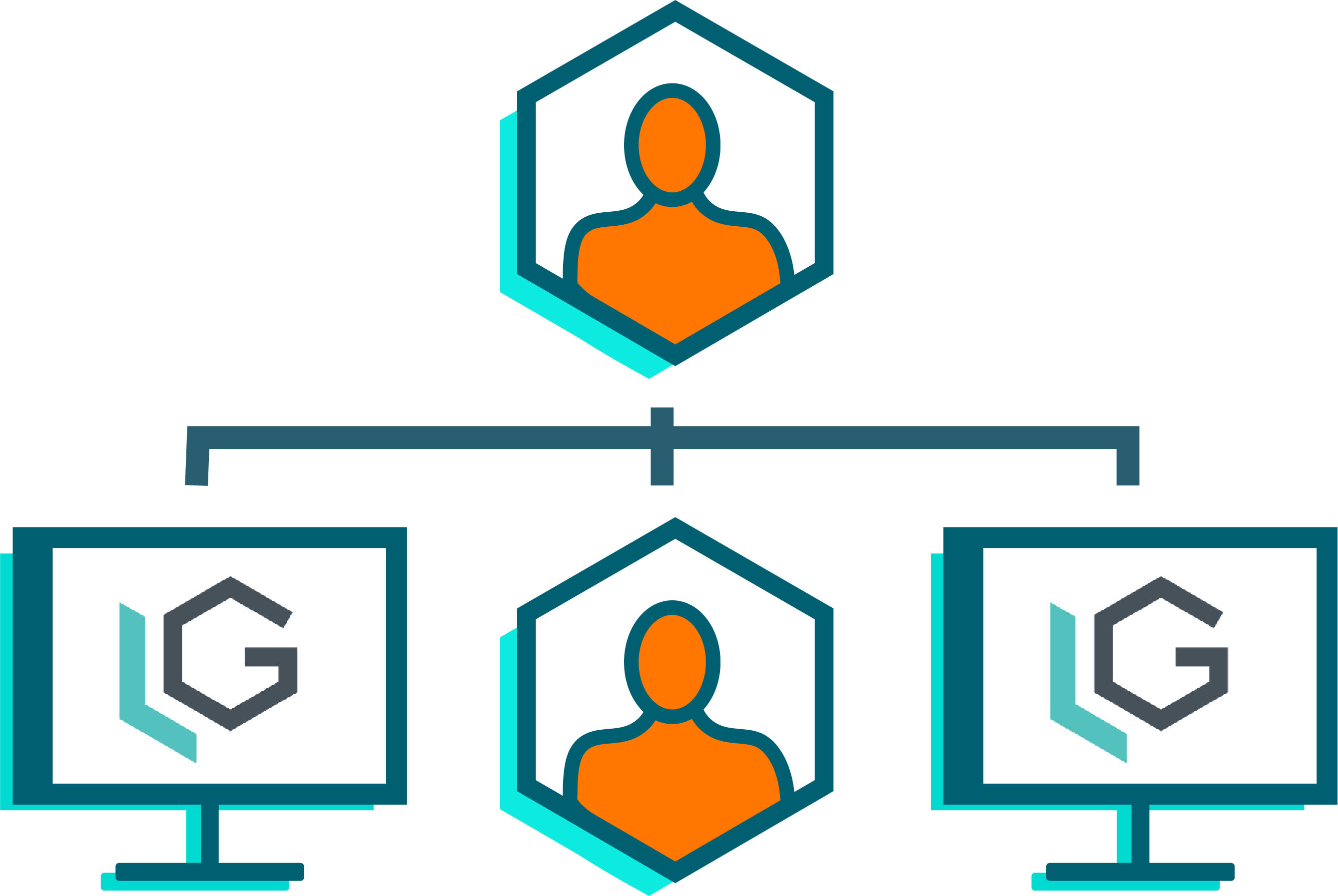
How Humans Learn, How Machines Learn, and How We Learn Together
By Frank Ricotta, CEO & Founder BurstIQ
During my freshman year in college, I was carrying a hefty course load and getting behind in several classes. One short visit home, my mom asked how things were going. When I told her about my struggles, she sat me down and taught me a new way to take notes.
She introduced me to mind mapping, which is a visual note-taking technique that begins with a central idea and expands outward to explore and connect related themes and subtopics. Mind mapping involves the use of keywords, images, and colors to create a non-linear, intuitive map of thoughts. This makes it perfect for brainstorming, organizing complex information, and improving memory and learning. Mind maps allow for flexible thinking and uncovering connections between ideas, which is different from traditional linear notes. Looking back, I realize this was my first exposure to graphs and graph models.
One thing you should know about my mom is that she has a doctorate in education and has long been considered one of the foremost experts on early childhood development and whole-brain learning. While mind mapping isn’t for everyone, my mom said that I am predominantly a visual learner, and taking notes this way aligns with how I think and learn. That short tutorial has stuck with me throughout my life. I still use this technique today when brainstorming new product ideas, trying to solve a problem, and just embarking on a self-learning journey for something new. Because of this, it shouldn’t be a big surprise that graph theory is fundamental to our product at BurstIQ.
As we strive to comprehend the learning process, we often compare the two most advanced learning systems we know: the human brain and artificial intelligence (AI). Although they are both remarkable, they function and learn in fundamentally disparate ways. With the world rapidly adopting more AI, it becomes imperative that leaders understand these differences and create a trustworthy culture where people and AI can learn together.
How Humans Learn
Humans are born learners. From our first breath, a complex interplay of genetics, environment, and experience shapes our learning journey. Wouldn’t it be nice if we could all learn as fast as a three-year-old? Human learning is a rich, multi-faceted process influenced by an individual’s sensory experiences, emotions, and cognitive abilities.
The primary learning styles include:
Beyond these, humans can be abstract thinkers – pondering concepts, ideas, and problems that aren’t physically present. This abstract capability allows for creative problem-solving, innovation, and the development of complex languages and cultures.
I bet as you were reading the list above, people you know started popping up in your head. People you work with, your boss, family, and friends.
How Machines Learn
Enter the realm of AI, where ‘learning’ takes on a different hue. AI, particularly in forms like generative models (GPT-3 for text or DALL-E for images), neural networks, and deep learning systems, ‘learn’ through data processing and pattern recognition. They often can mimic human learning styles, but they do so from a much different foundation. These systems analyze vast datasets, identify patterns, and make decisions based on their programming.
The primary AI learning methods include:
Unlike humans, AI currently lacks the ability to think abstractly or emotionally. Its learning is confined to the data it’s fed and the parameters set by its human creators. Data can significantly skew these models depending on the quality of the data, how good or bad it may be, and inherent biases contained in the data. Corrupting data will be one primary attack vector for bad actors to disrupt AI-based solutions.
Current generative AIs have demonstrated the ability to create new things based on the data they have consumed and the parameters we set when interacting with them. This is only going to get better and better. The question is, when will machines cross over and become truly aware? Will they ever cross that boundary? Given their ability to mimic, will we know? At this point, these models have a broader command of language than most individuals. When we think of language, we also have to consider computer language – i.e., software.
Comparing Human and Computer Learning
When we compare human learning to computer learning, the differences are quite noticeable. Humans learn by experiencing a wide range of emotions and contexts, driven by abstract thinking and creativity. We make connections based on our overall understanding and experience, rather than just a specific context. On the other hand, AI is highly efficient in processing and analyzing large datasets, which is beyond human capability. However, AI lacks the nuances of human thought, emotion, abstract thinking, and creativity. Although AI can make quick and efficient decisions by identifying patterns, these decisions are limited to the context provided and are only as good as the data and algorithms that drive them.
Learning Together – The Synergy of Human and AI Learning
The future, however, lies in the synergy between human and AI learning. AI can augment human learning by providing personalized educational experiences, solving complex problems, and handling data-driven tasks. Conversely, human oversight ensures that AI operates within ethical bounds and complements human capabilities rather than replacing them. Together, they offer a powerful tool for advancement in various fields.
A Continuously Learning Ecosystem Powered by LifeGraph®
At BurstIQ, we see the collaboration between humans and machines, facilitated by LifeGraph, as a way to drive innovation. LifeGraph integrates large datasets, providing a comprehensive view of data. All data in LifeGraph has context and trust built-in, and smart contracts manage the ownership and sharing of data. LifeGraph organizes data in a way that is intuitive for both humans and machines using knowledge graphs. Knowledge graphs provide a visual representation of relationships between ideas, making it easier for humans to understand complex concepts and for machine learning models to make smarter decisions.
As new information gets added to LifeGraph, it automatically establishes connections with existing nodes and relationships. Humans interacting with the graph provide input that refines the LifeGraph’s accuracy and expands its understanding of the world, and your LifeGraph ecosystem becomes more intelligent and valuable over time. It’s a testament to the power of interconnectedness and the potential of collective intelligence to create a truly enriching learning experience for everyone.
Conclusion
The journey through human and AI learning landscapes reveals distinct paths marked by remarkable capabilities and limitations. As technology evolves, understanding these differences becomes crucial, not just for developing more advanced AI but for bettering our educational strategies and appreciating the unique aspects of human cognition.
About BurstIQ:
BurstIQ’s LifeGraph is an advanced data management platform that enables secure, transparent, and efficient data management for businesses across various industries. By harnessing the power of blockchain technology, BurstIQ empowers organizations to quickly integrate data from any source so they can create a trustworthy business ecosystem. The platform applies knowledge graphs to make data more accessible and to deliver insights that optimize processes, reduce risk, and personalize customer solutions across their ecosystems. With a strong focus on innovation and customer-centricity, BurstIQ continues revolutionizing industries and setting new standards in enterprise blockchain-powered Web3 solutions.
To learn more about how LifeGraph can help you extend your capabilities to build trustworthy, AI-ready exchanges, and robust data analytics from a single, powerful platform, please contact us here.