Knowledge Burst Series:
Conquering Data Chaos
to Win in an AI World
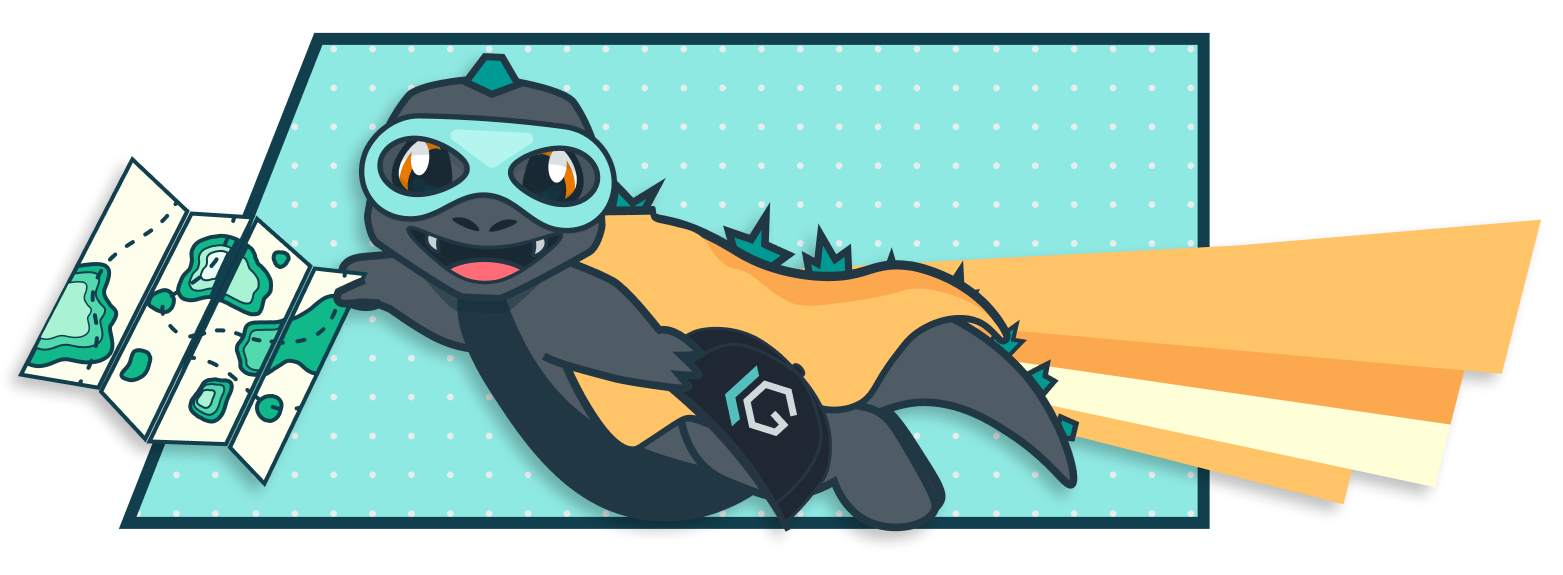
In a world increasingly driven by data, the landscape can often feel chaotic and overwhelming. The urgency to implement AI both successfully and ethically has never been greater. Join us in this Knowledge Graph Series as we embark on an exhilarating adventure with our hero, Blast. Equipped with his powerful tool, LifeGraph®—the next generation of data management—Blast tackles the chaotic landscape of data. Prepare to explore the intricacies of data-driven innovation and discover how to harness its true potential!
At BurstIQ, we make data your superpower.
We’ve found our customers identify with the following data personas:
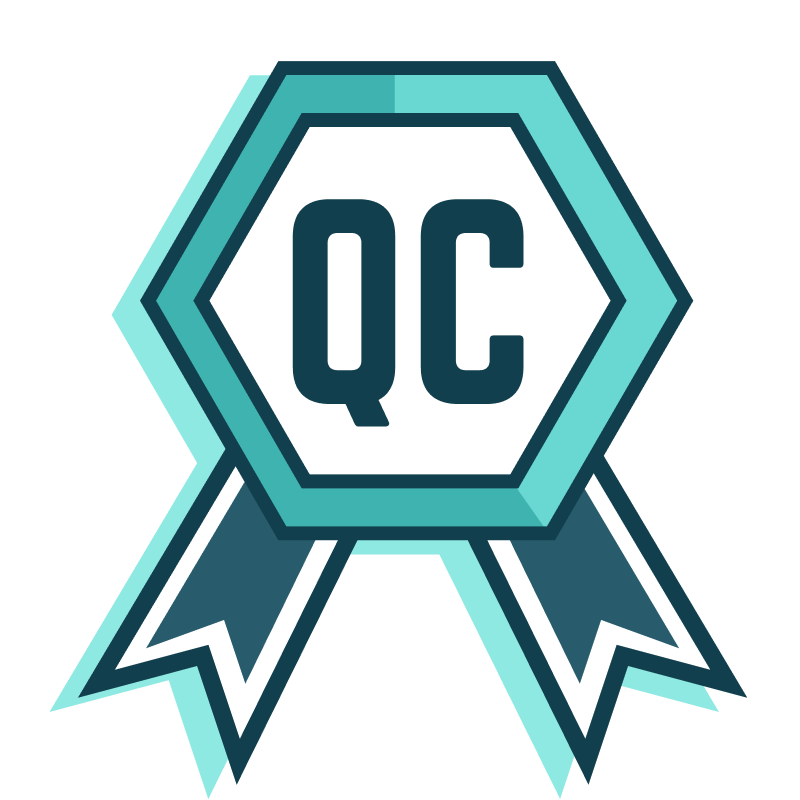
Quality Commander:
The relentless champion of data integrity, tirelessly working to ensure that information is not just abundant but also accurate and actionable.
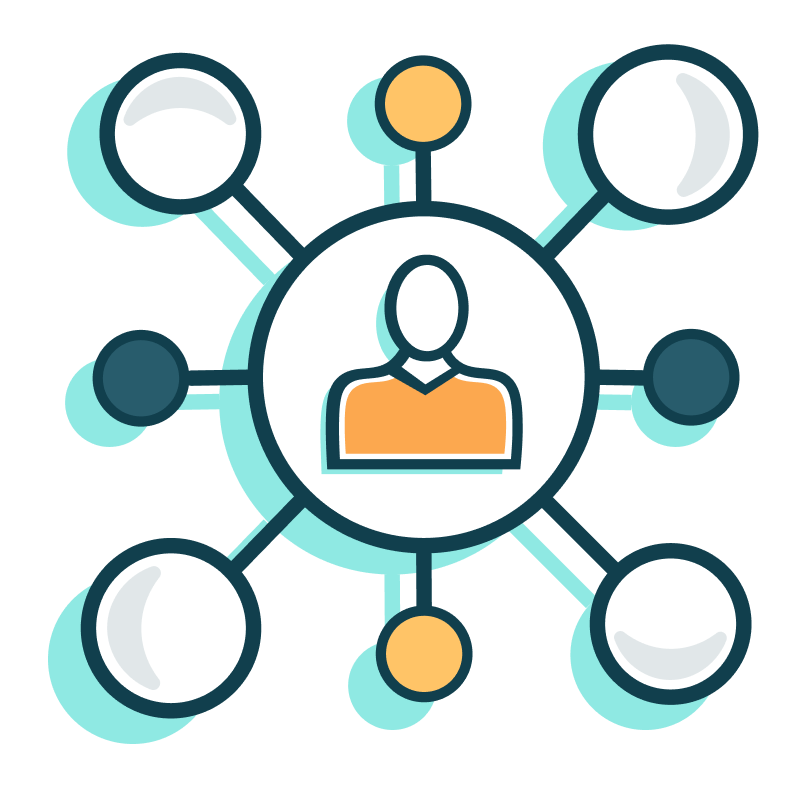
THE GREAT UNIFIER:
The collaborative force that bridges gaps between silos, systems, departments, and disciplines, fostering a culture of data-sharing and teamwork.
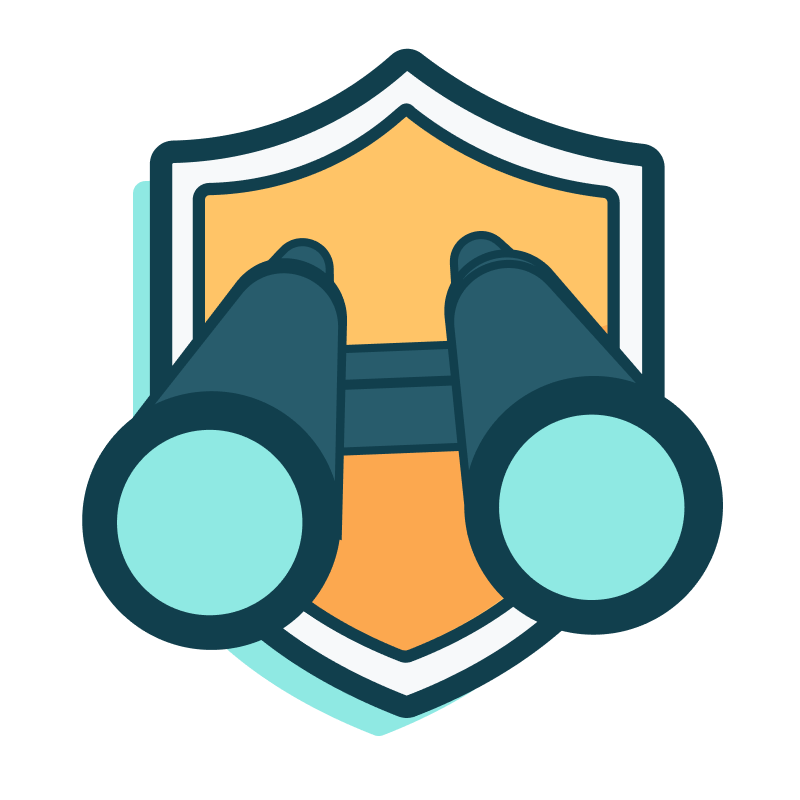
GUARDIAN OF PRIVACY:
The vigilant protector of personal information, committed to safeguarding privacy while navigating the complexities of data collection and usage.
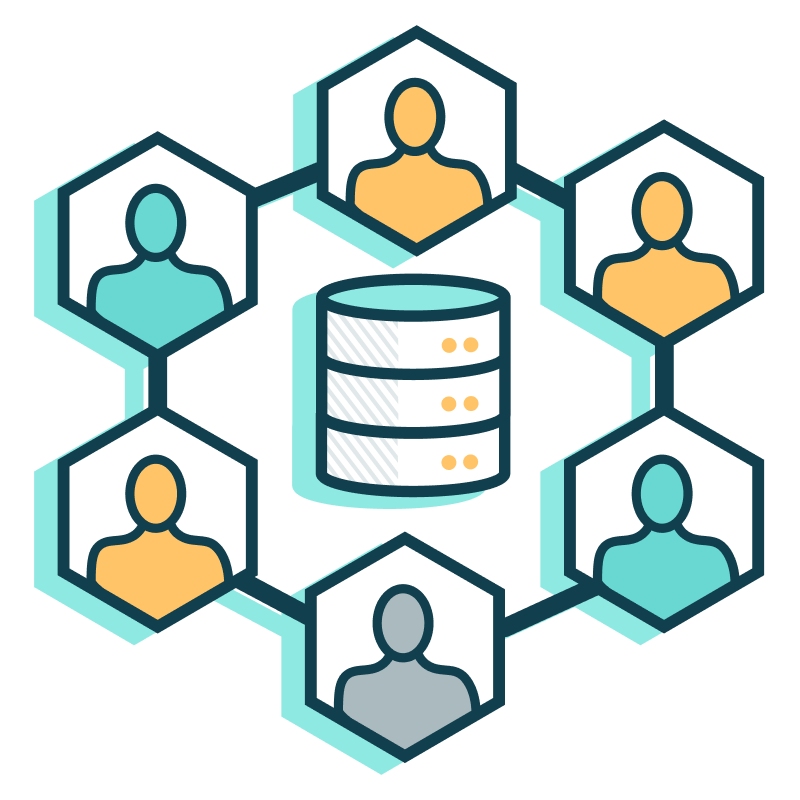
DATA DEMOCRATIZER:
The passionate advocate for accessibility, striving to empower everyone in their business ecosystem with the knowledge and tools to leverage data for their benefit.
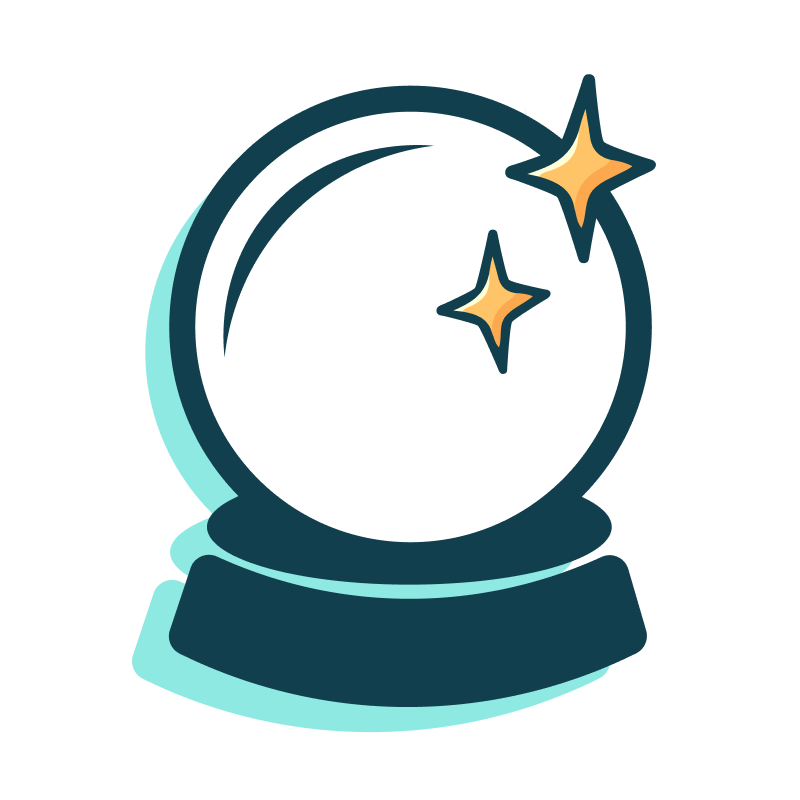
AI Activator:
The visionary architect of intelligent systems, harnessing the power of artificial intelligence, machine learning, and generative AI as a force multiplier to enhance team productivity, personalize engagements, and accelerate the pace of innovation.
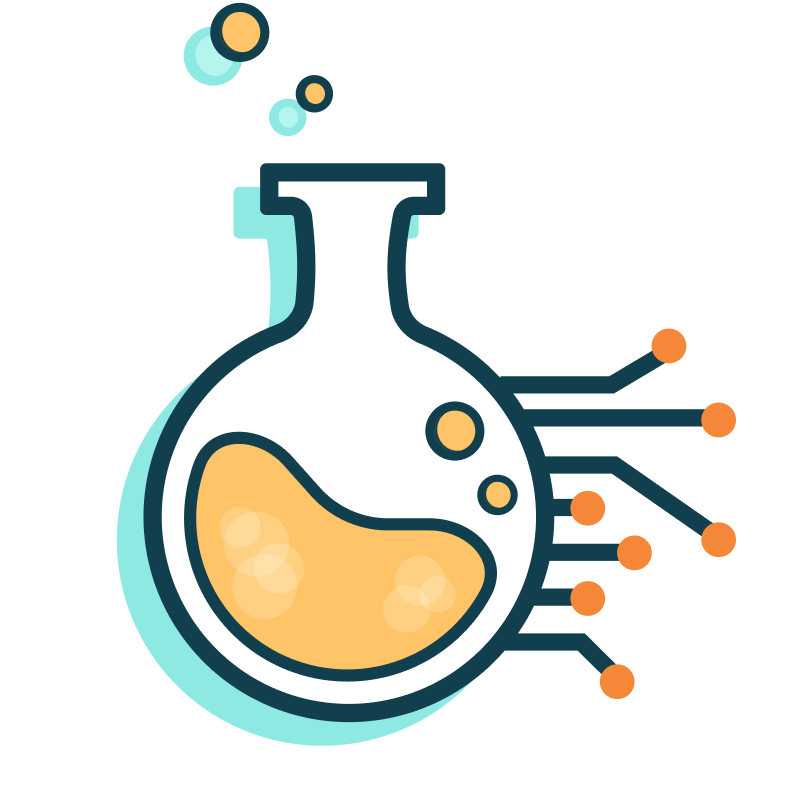
AI ALCHEMIST:
A master of transforming raw data into golden opportunities, using AI to create innovative solutions for market differentiation and business growth.
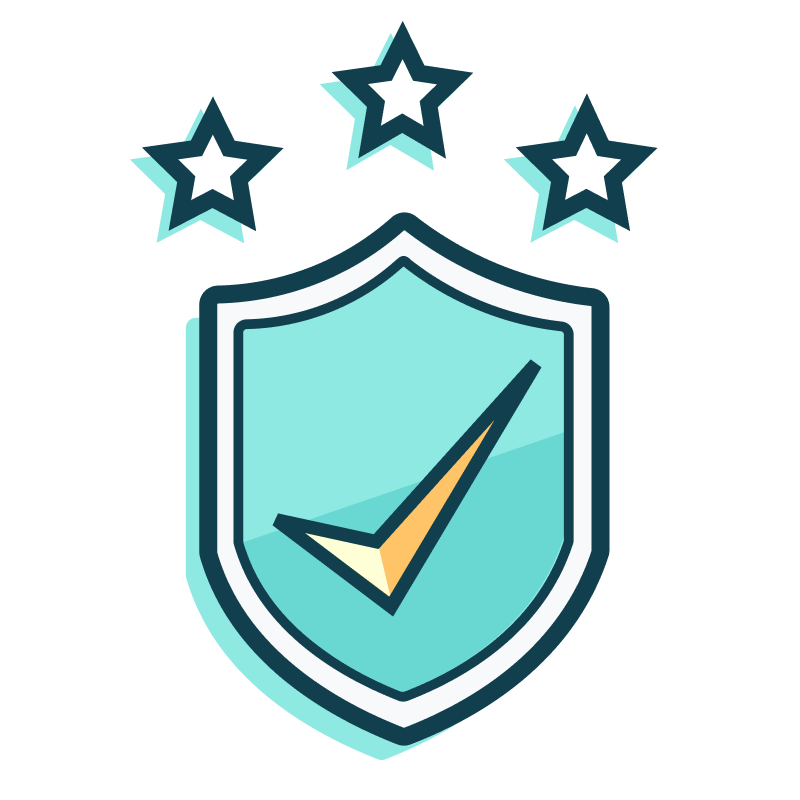
COMPLIANCE CRUSADER:
The unwavering enforcer of regulations and ethical standards, ensuring that data practices align with laws and best practices to protect both organizations and consumers.
Throughout this Knowledge Graph Series, we will dive deep into each persona, celebrating their unique missions and the challenges they face in the ever-evolving data landscape. You’ll learn how LifeGraph helps you tackle some of the biggest obstacles in data management.
Chapter 1:
Quality Commander
blast’s quality
commander saga
Passionate about making sure that every piece of data collected is spot-on and reliable, Blast knows the implications of poor quality on downstream operations—especially AI. With a keen eye for detail, Blast strives to root out errors and maintain high standards, creating a culture where teams can trust their data to make smart, informed decisions.
The Pursuit of AI-Ready Data
The first step is understanding what AI-ready data really means. AI-ready data isn’t just about being clean or structured; it’s about being representative, comprehensive, and aligned with specific AI use cases. Data should encapsulate all relevant patterns, errors, and outliers that an AI model might encounter in real-world applications.
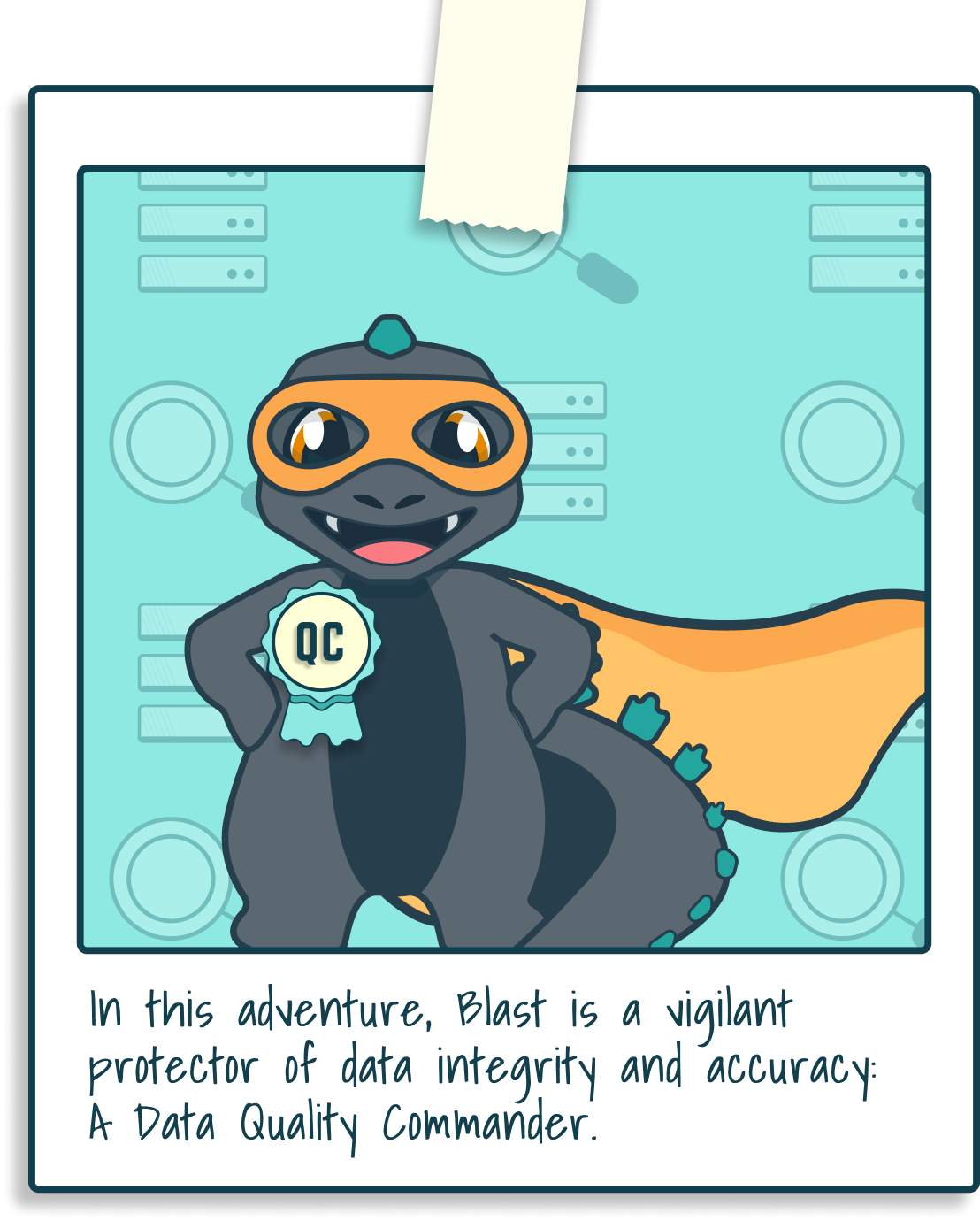
AI-ready data is optimized for use in AI models and applications, defined by its cleanliness, organization, and relevance. The quality of data input into AI systems is directly linked to the accuracy and reliability of the results produced. As AI technology evolves, implementing strong data strategies becomes essential. AI-ready data requires greater variability and alignment with the AI’s learning objectives and often necessitates real-time or near-real-time quality.
The pursuit of AI-ready data must transcend the notion of mere data value. Data has to transform into a secure and reliable asset enriched with context (origin, lineage, changes over time, etc.) and trust (ownership, privacy, and security). This involves integrating active metadata rather than relying solely on static metadata, encompassing essential features such as longitudinal data and chain-of-custody attributes.
By taking these steps, organizations can develop AI-ready data and address fundamental data quality issues, such as incompleteness, duplication, inconsistent formats, and poor labeling, that have long been challenges in data management.
how LifeGraph helps:
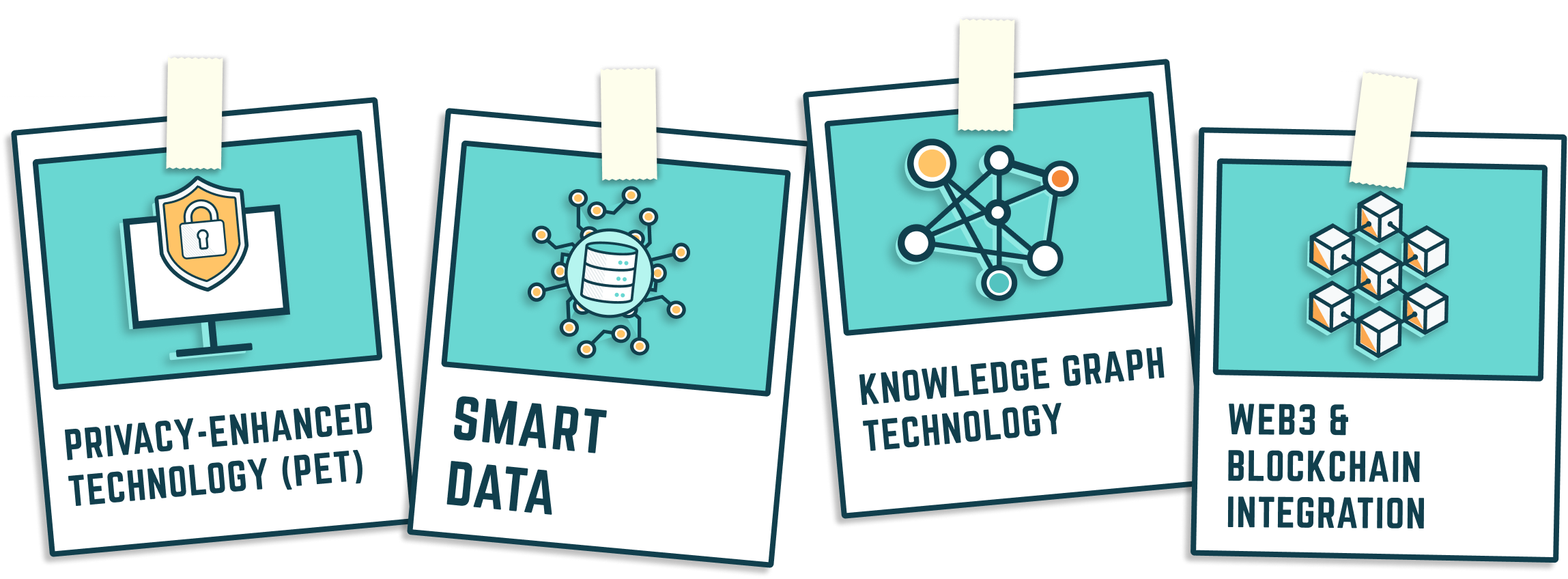
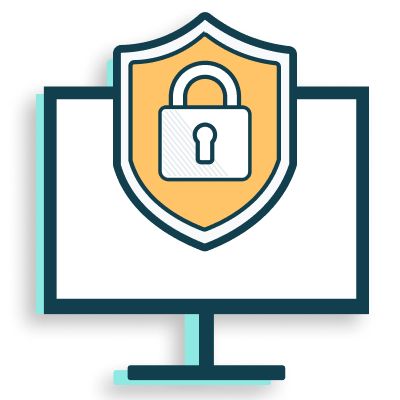
Privacy-Enhanced Technology (PET):
LifeGraph’s privacy-enhancing technology protects personal data in untrusted environments. This essential capability addresses evolving privacy laws and growing consumer concerns. By using various privacy protection techniques, LifeGraph enables valuable data insights while ensuring compliance with regulations.
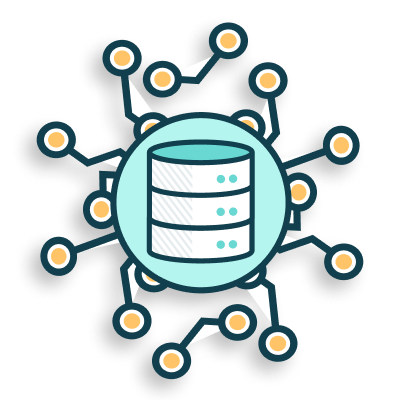
Smart Data:
LifeGraph connects data and transforms it into Smart Data. Smart Data is privacy-enhanced data enriched with trust (privacy, security, and ownership) and context (origin, lineage, changes over time, etc.), making data inherently more valuable for AI applications.
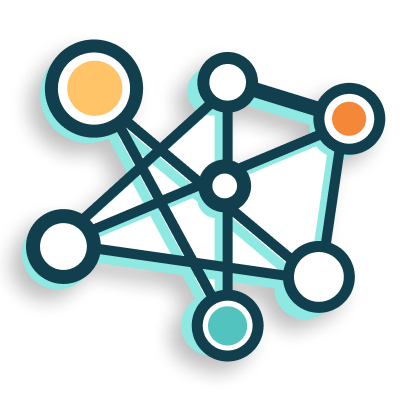
Knowledge graph technology:
LifeGraph uses knowledge graphs to store data and the relationships between data points, enhancing data quality by ensuring relevance and accuracy. This relational understanding is pivotal for AI, especially LLMs, which thrive on interconnected data for better contextual understanding.
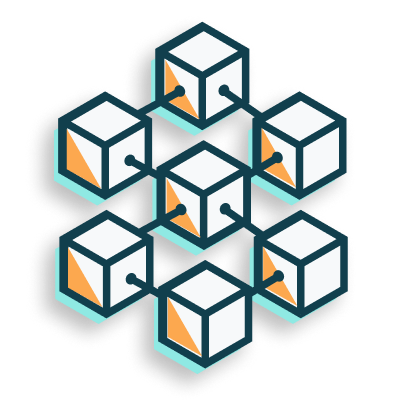
WEB3 & BLOCKCHAIN INTEGRATION:
By leveraging blockchain, LifeGraph ensures data provenance, security, and granular ownership controls which is foundational for trust in data. This integration also supports privacy-enhancing techniques crucial for sensitive data like healthcare or personal information. You can learn more in our previous Knowledge Burst: How a Web3 Data Fabric Can Help You Leapfrog the Market.
Data Governance
Data governance is critical to maintaining data quality. The framework must include policies for data quality, stewardship roles, intellectual property protection, and compliance with regulations. This framework should ensure that data quality is managed throughout its lifecycle and the entire data ecosystem. The only approach to managing this aspect of data quality is to implement a distributed governance mechanism that can be enforced in real-time or near real-time. That means governance rules must be embedded with the data transactional and access mechanisms.
Data governance must go beyond an administration function and extend to data lineage and provenance. The data ecosystem must be able to implement systems for tracking data lineage to understand where data comes from, how it’s transformed, and its quality throughout its journey. This is crucial for AI models, as understanding data origins can affect model reliability.
how LifeGraph helps:
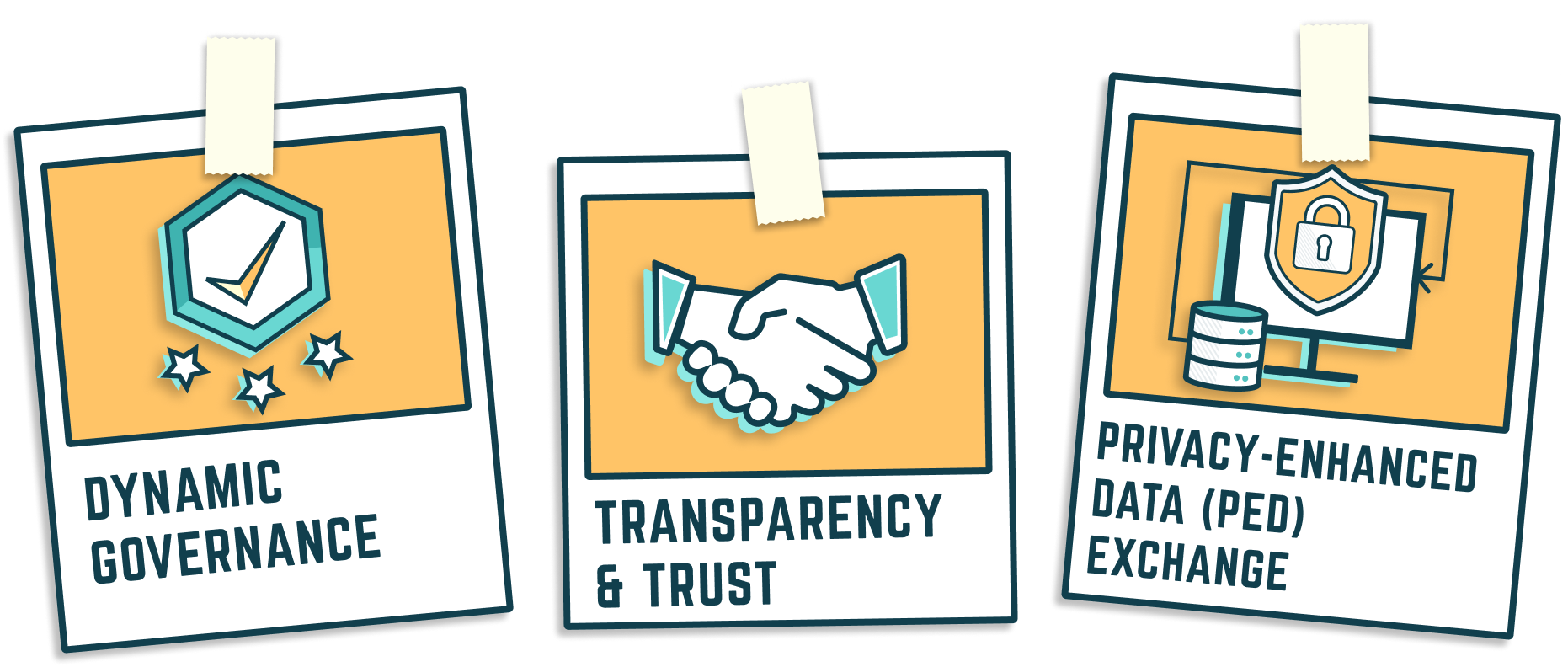
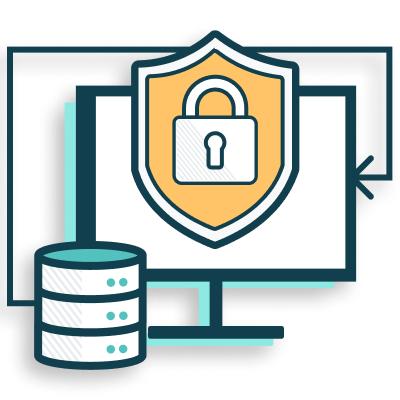
privacy-enhanced data (ped) exchange:
LifeGraph’s privacy-preserving infrastructure leverages blockchain services to empower organizations to securely share data across partners while maintaining control over who accesses what information. Ownership is assigned to each data asset using consent contracts, ensuring data is only shared if the owner consents.

dynamic governance:
LifeGraph employs blockchain technology to orchestrate how data moves throughout your organization. Consent Contracts ensure data can only be shared if the owner consents. Smart Contracts are self-executing agreements with the terms directly written into code and recorded on the blockchain. This automation ensures that data governance policies are enforced consistently without the need for intermediaries. For instance, they can automatically execute actions based on predefined conditions, ensuring compliance with regulations and organizational policies.
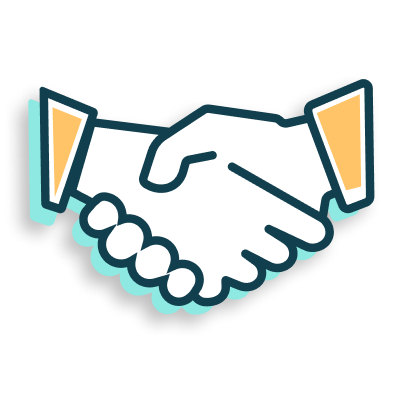
transparency & trust:
LifeGraph uses a decentralized ledger system to record all data transactions transparently and immutably, creating a reliable audit trail that stakeholders can trust. Moreover, Knowledge Graphs allow for visualizing data relationships, enhancing transparency and fostering better data governance. This solid foundation is crucial for ensuring high data quality in AI applications.
Continuous Data Quality Monitoring
Given AI’s need for up-to-date data, the Quality Commander must strive to implement continuous monitoring systems that alert when data quality drops below acceptable thresholds, affecting AI model performance. These mechanisms must be implemented at least at two critical points within the broader data ecosystem.
The first is at the ingestion point. At this point, organizations can establish a set of rules and parameters based on the targeted quality level.
It is at this point where AI-capable data pipelines and APIs are very advantageous, and given the monotonous detail required to perform this task, it is a perfect place for automated AI agents.
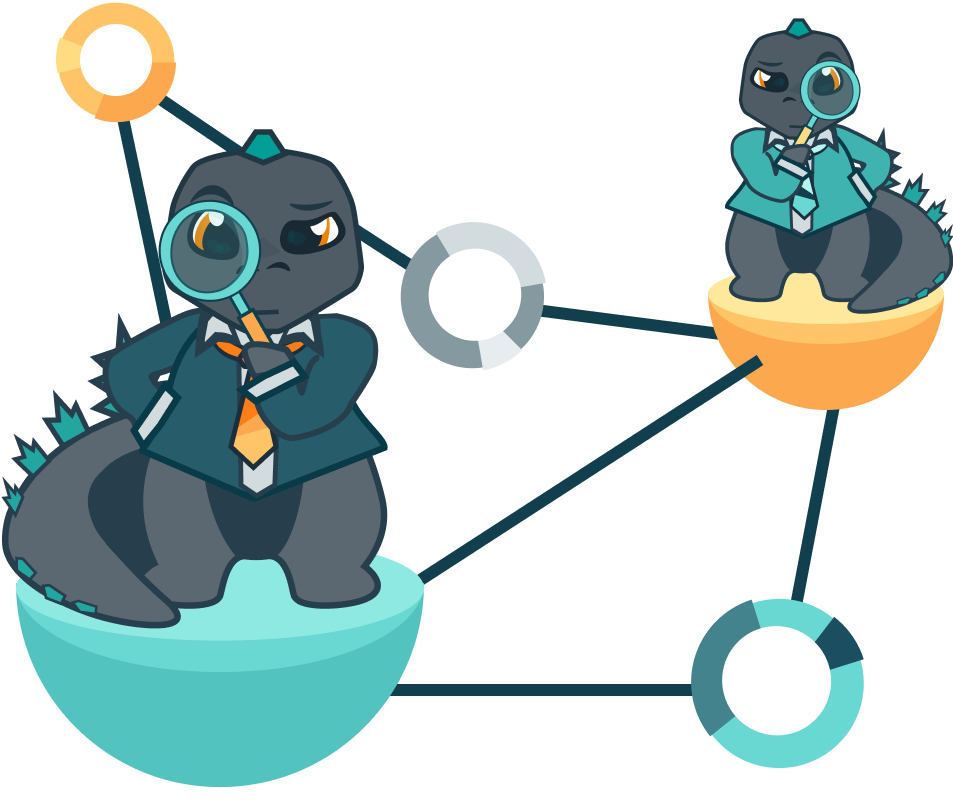
The second would be at the point of data consumption, where organizations can establish mechanisms to capture AI models’ performance feedback to inform data quality processes, creating a loop where data quality improvements directly enhance AI outcomes.
how LifeGraph helps:
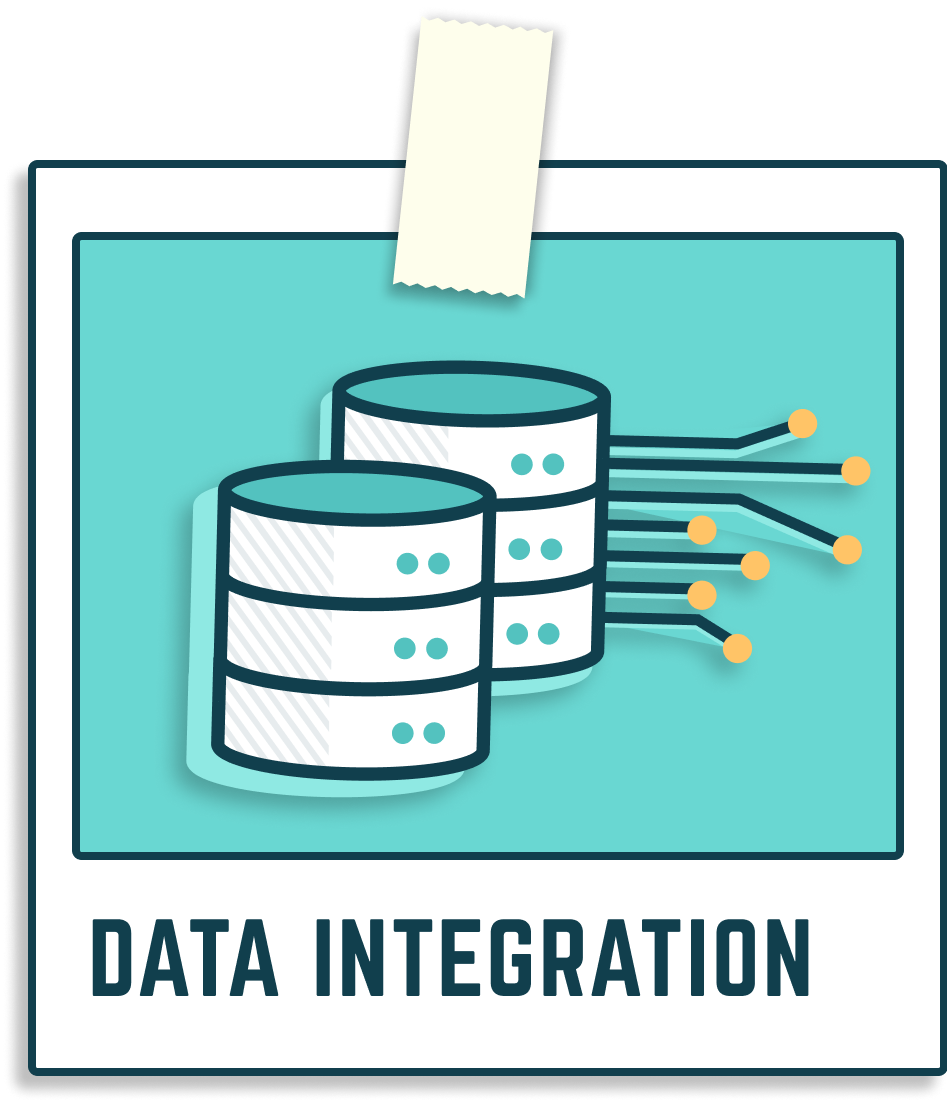
DATA integration:
LifeGraph connects all your systems and data sources through REST APIs and ingests data through adaptive data pipelines that include built-in data validation and cleansing steps, which ensures that only high-quality data is ingested and processed.
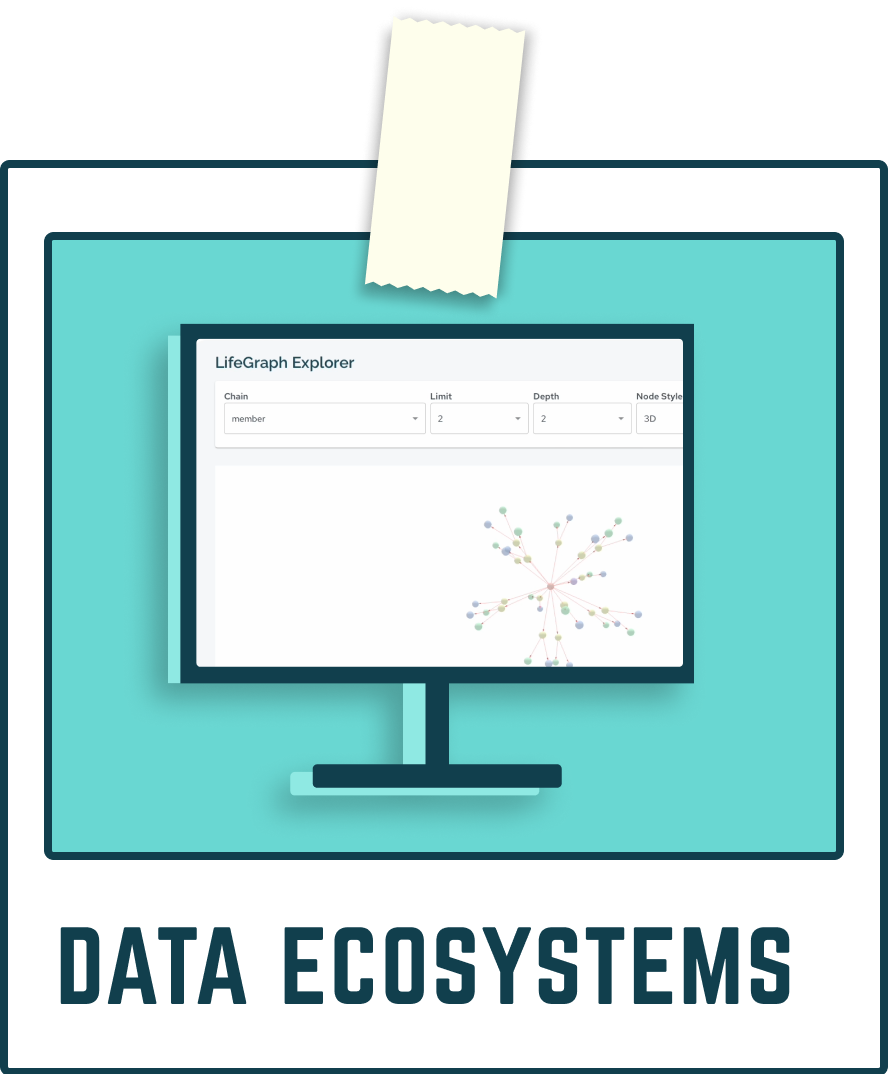
DATA ECOSYSTEMS:
With LifeGraph, data quality isn’t static. As new data from across your business ecosystem is integrated, the graph evolves, automatically updating relationships and ensuring ongoing data quality.
Data Quality as Part of AI Model Development
A Quality Commander knows quality needs to be an integral part of AI model development. The risks associated with poor-quality data in AI models are substantial, affecting everything from accuracy and bias to costs and overall effectiveness. Ensuring high-quality data is crucial for the successful deployment of AI technologies.
It is important for data teams to incorporate data quality checks as part of the training pipeline. This could mean filtering out low-quality data or weighting it based on quality. Additionally, when assessing the AI model’s effectiveness, teams need to understand the sensitivity of the AI model to data quality issues. Some models might perform poorly with slight data degradation, requiring higher data quality standards. This includes when data is looped back on itself or when the model is only trained on synthetic data with no mechanism to adjust when exposed to real-world data assets.
how LifeGraph helps:
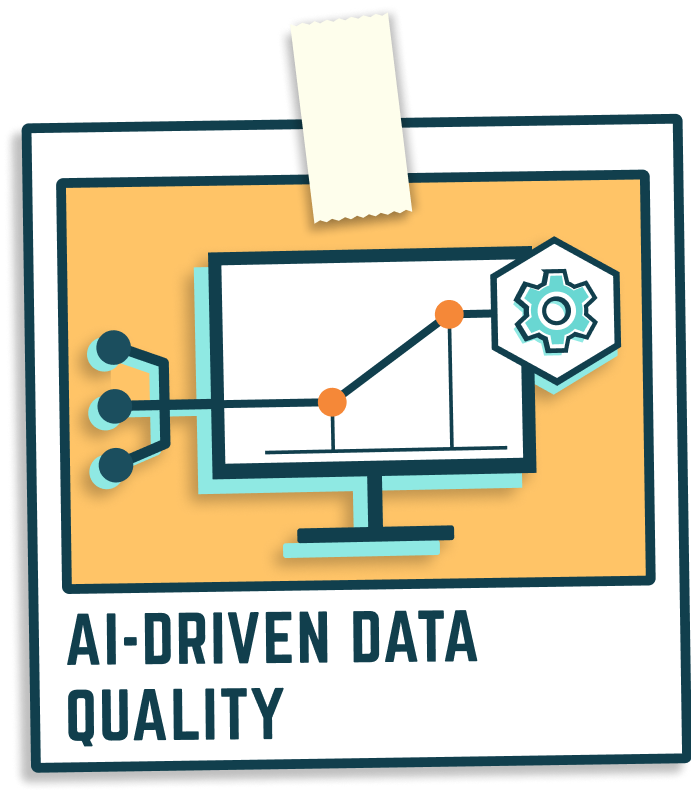
ai-driven data quality:
LifeGraph enables AI models to understand and infer from data in ways that traditional databases cannot, leading to more nuanced data quality checks based on AI’s understanding of data patterns and anomalies.
Cultural Shift & Training
Leadership is vital to a long-term sustainable data quality initiative. They must promote a culture of data literacy where all employees understand the importance of data quality for AI. There needs to be training on handling data, reporting quality issues, and understanding the impact of their data contributions.
They must also encourage a bottom-up approach where data users at all levels can contribute to data quality improvements through reporting mechanisms or direct data curation.
Data quality governance needs to be pushed to the edge and function more as a distributed autonomous organization vs a centralized bureaucracy.
how LifeGraph helps:
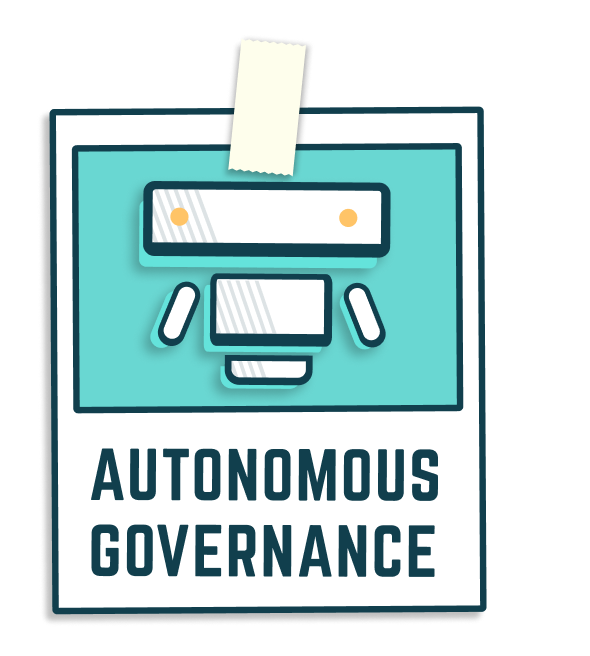
AUTONOMOUS GOVERNANCE:
LifeGraph leverages Consent Contracts to establish governance rules, significantly minimizing manual oversight. This ensures seamless and consistent governance maintenance.
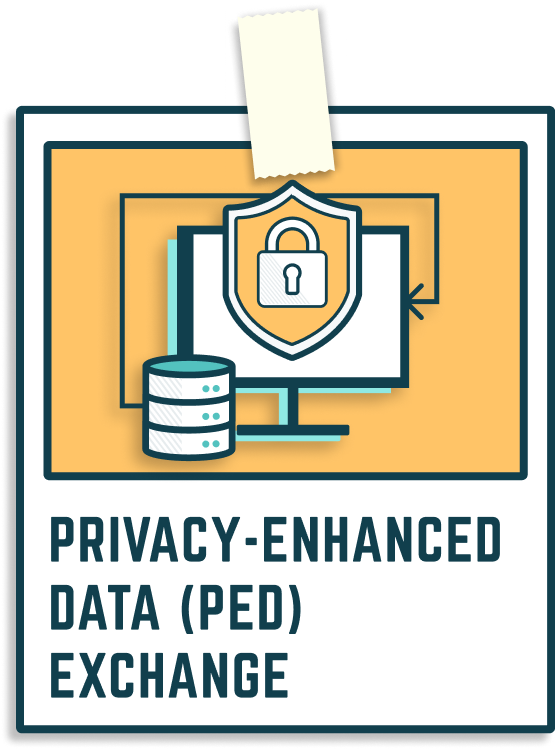
privacy-enhanced data (ped) exchange:
LifeGraph’s privacy-preserving infrastructure leverages blockchain services to empower organizations to securely share data across partners while maintaining control over who accesses what information. Ownership is assigned to each data asset using consent contracts, ensuring data is only shared if the owner consents.
Scalability & Adaptability
Data quality initiatives must scale with the enterprise’s data growth. That is why data quality metrics, standards, and enforcement must be part of the data flow and not implemented as an external solution.
As AI technologies evolve, so will the requirements for data. The initiative should be adaptable and ready to incorporate new data quality metrics, data structures, or standards that future AI models might demand. The only way to do this is by creating data assets vs data stores. We recently wrote about how to make this happen in this Knowledge Burst: The Next Wave: Data Stores to Data Ecosystems & Data Flows.
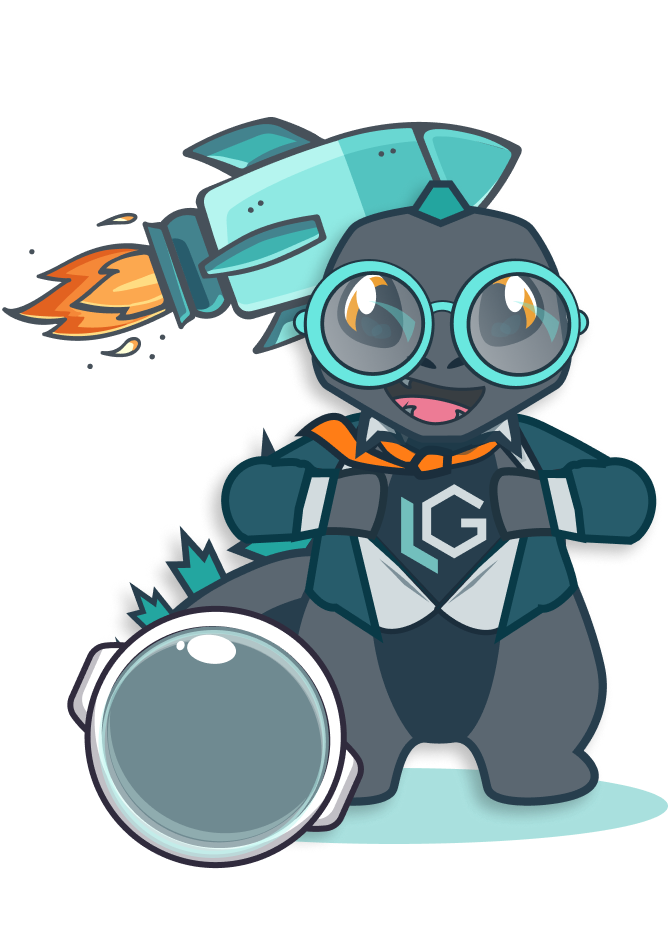
how LifeGraph helps:
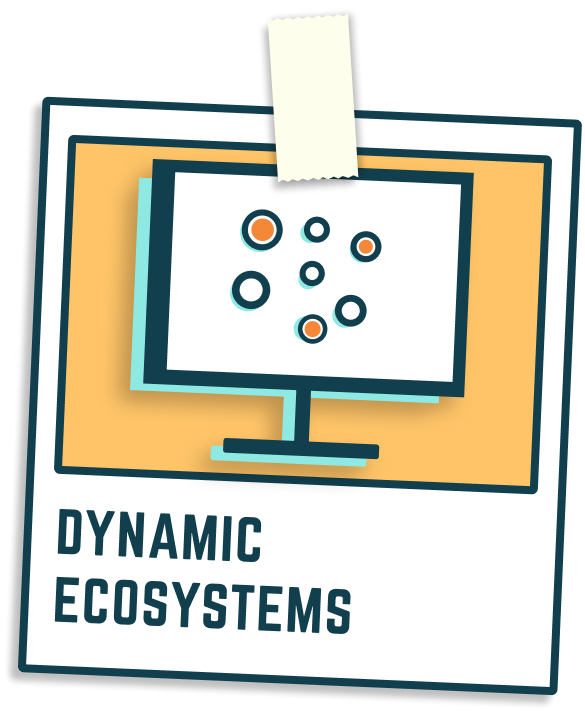
dynamic ecosystems:
LifeGraph empowers dynamic ecosystems capable of incorporating new data sources and evolving as organizational needs change. No need to write new data dictionaries and redeploy.
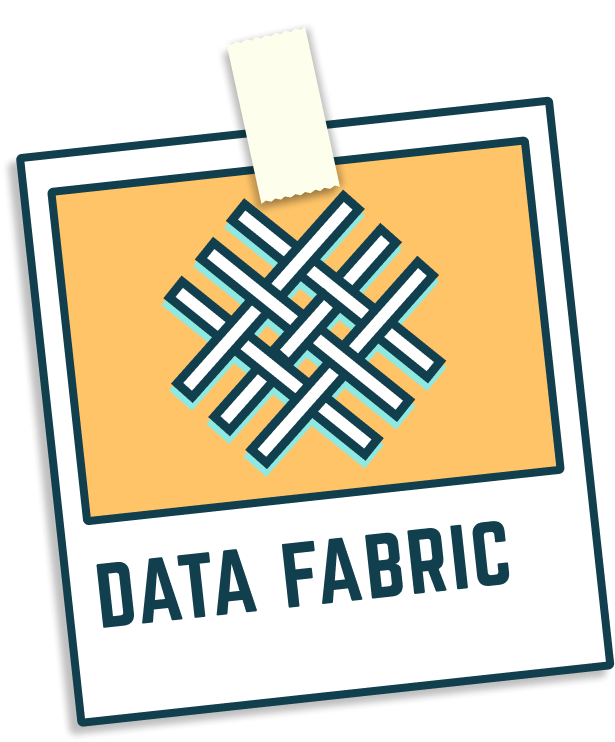
data fabric:
LifeGraph delivers a data fabric, providing a unified view of data across an organization’s ecosystem and ensuring uniform data quality management.
A Successful Quality Adventure
The evolution of data quality management, driven by adaptive APIs and platforms like BurstIQ’s LifeGraph, sets the stage for a new era of data-driven AI. By leveraging Smart Data, LifeGraph enhances data quality and builds trust and context into data assets, making them indispensable for organizations aiming to thrive in the AI-driven future. As we progress, data quality assurance will be a competitive advantage and a necessity for staying relevant in an increasingly data-centric world.
Are you a quality commander?
We’d love to connect with you to discuss how you’re defending data quality at your organization.
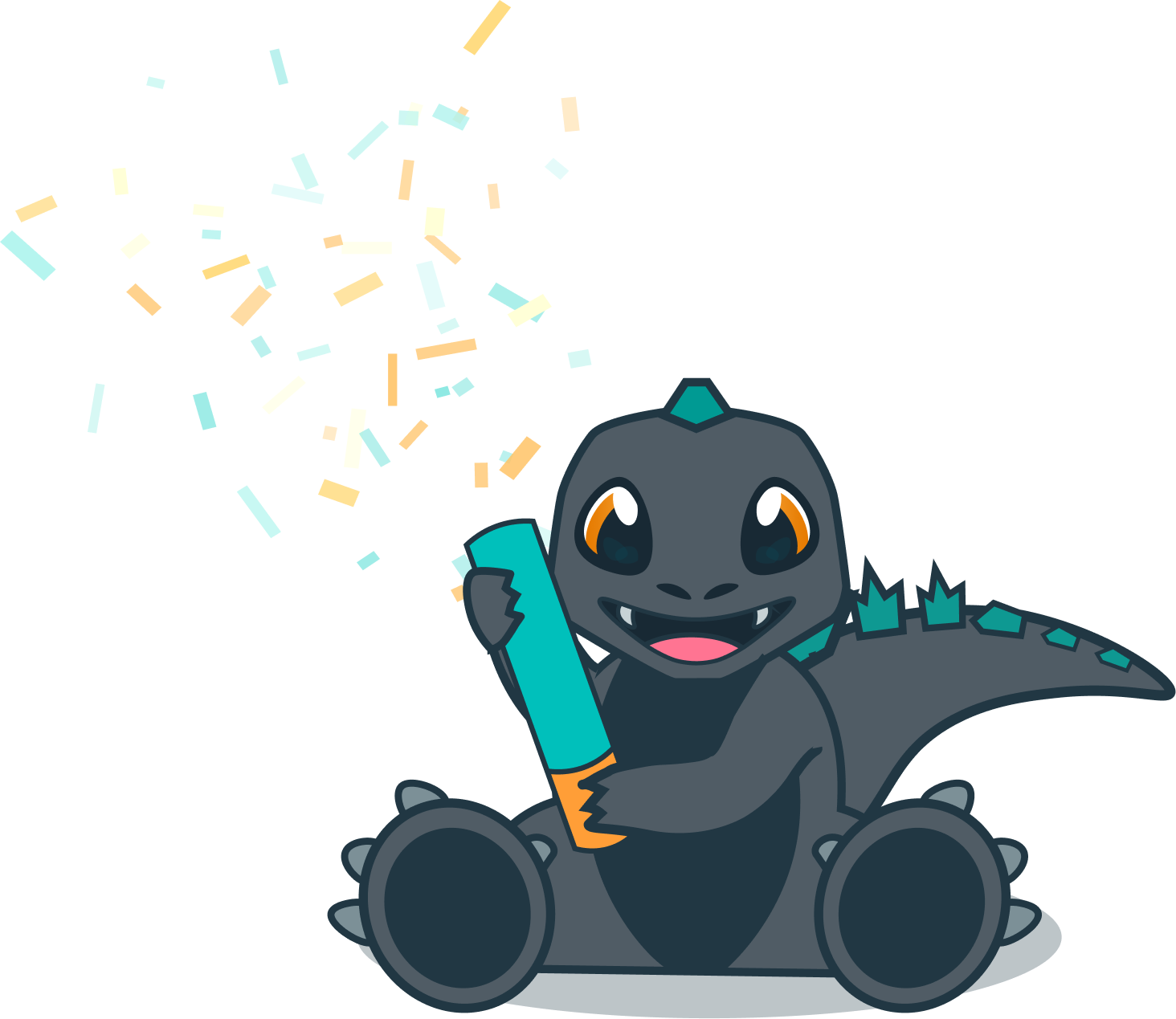
Knowledge Burst Series:
Conquering Data Chaos
to Win in an AI World
In a world increasingly driven by data, the landscape can often feel chaotic and overwhelming. The urgency to implement AI both successfully and ethically has never been greater. Join us in this Knowledge Graph Series as we embark on an exhilarating adventure with our hero, Blast. Equipped with his powerful tool, LifeGraph®—the next generation of data management—Blast tackles the chaotic landscape of data. Prepare to explore the intricacies of data-driven innovation and discover how to harness its true potential!
At BurstIQ, we make data your superpower.
We’ve found our customers identify with the following data personas:
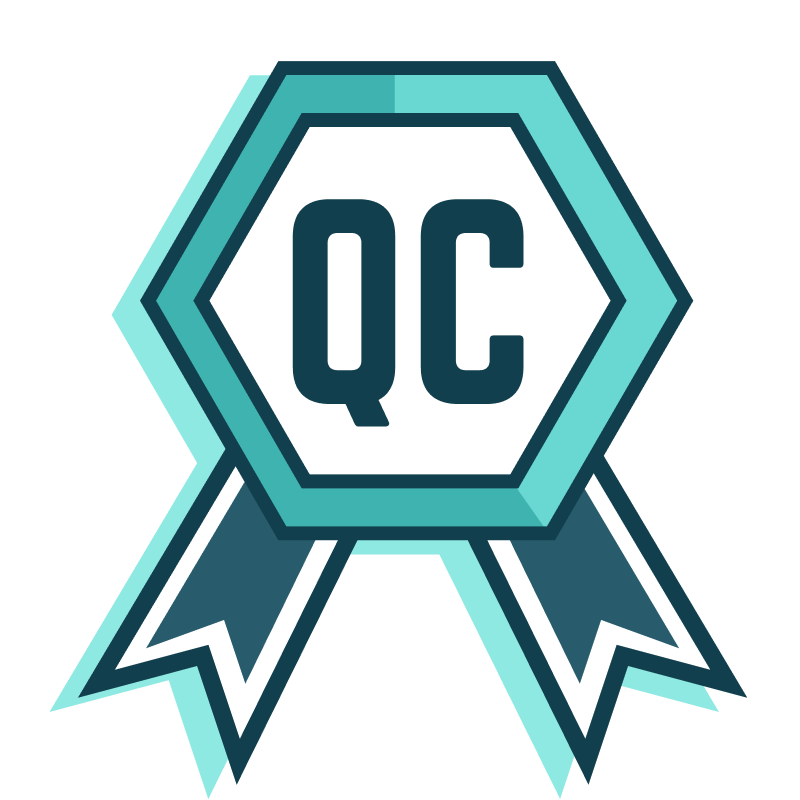
Quality Commander:
The relentless champion of data integrity, tirelessly working to ensure that information is not just abundant but also accurate and actionable.
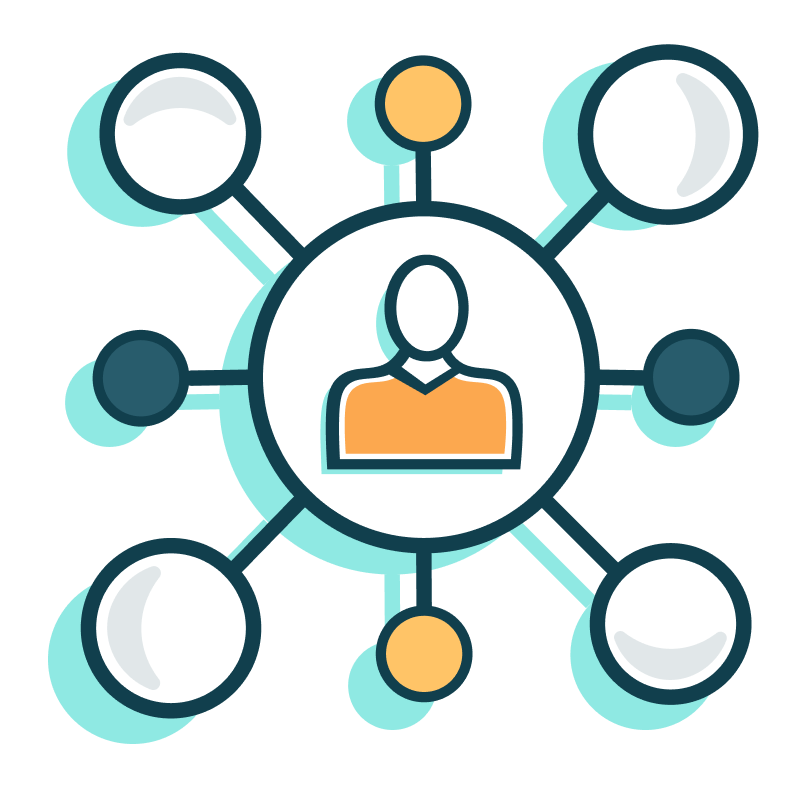
THE GREAT UNIFIER:
The collaborative force that bridges gaps between silos, systems, departments, and disciplines, fostering a culture of data-sharing and teamwork.
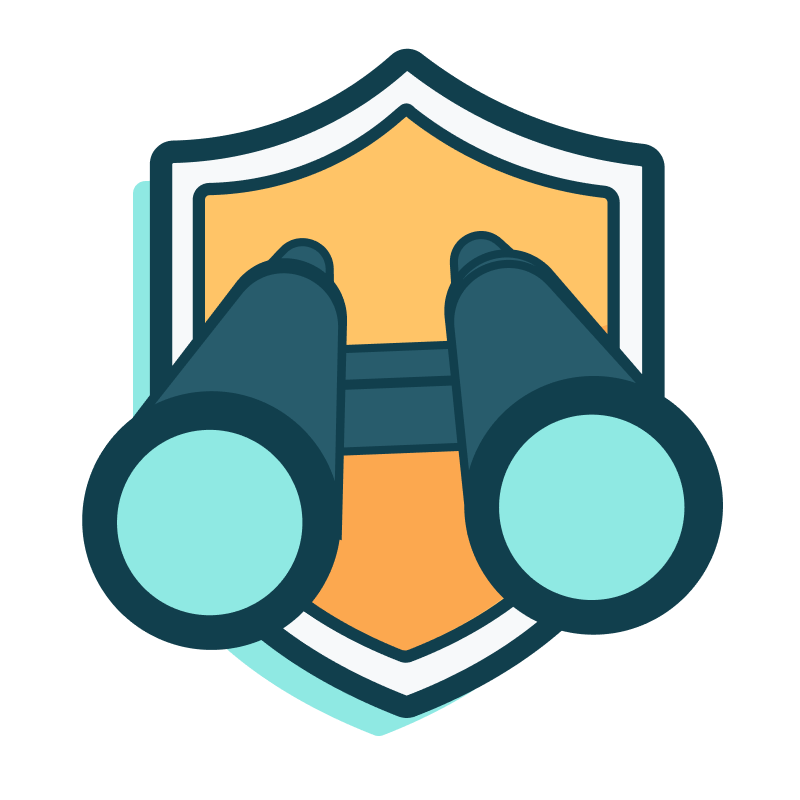
GUARDIAN OF PRIVACY:
The vigilant protector of personal information, committed to safeguarding privacy while navigating the complexities of data collection and usage.
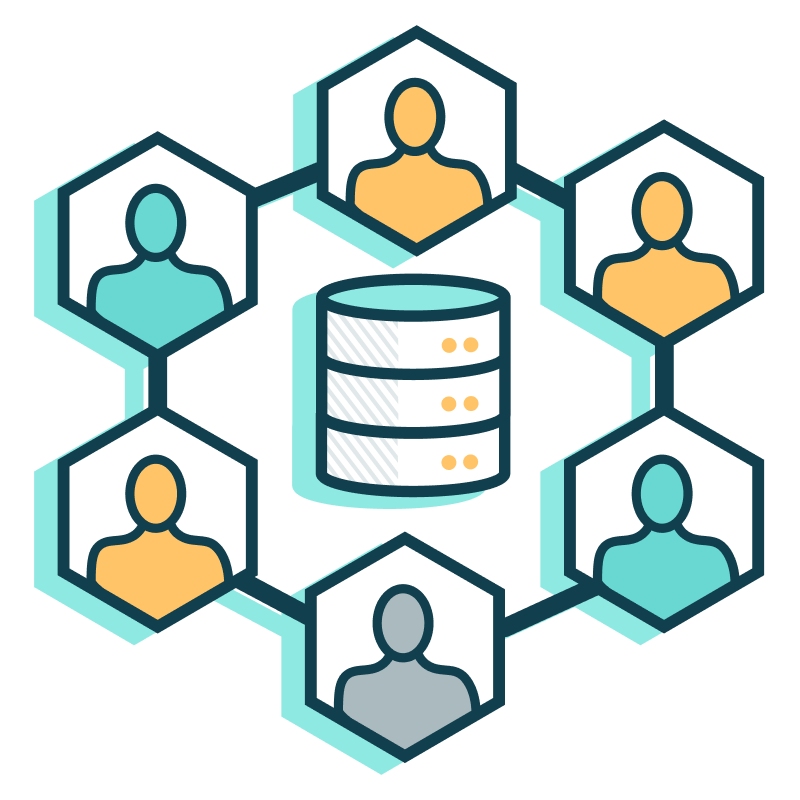
DATA DEMOCRATIZER:
The passionate advocate for accessibility, striving to empower everyone in their business ecosystem with the knowledge and tools to leverage data for their benefit.
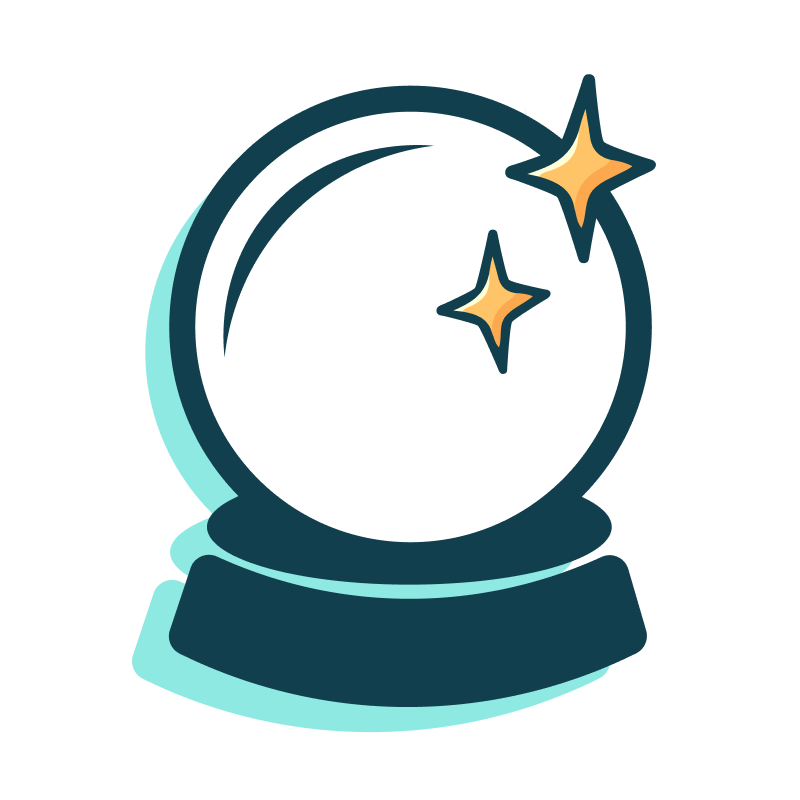
AI Activator:
The visionary architect of intelligent systems, harnessing the power of artificial intelligence, machine learning, and generative AI as a force multiplier to enhance team productivity, personalize engagements, and accelerate the pace of innovation.
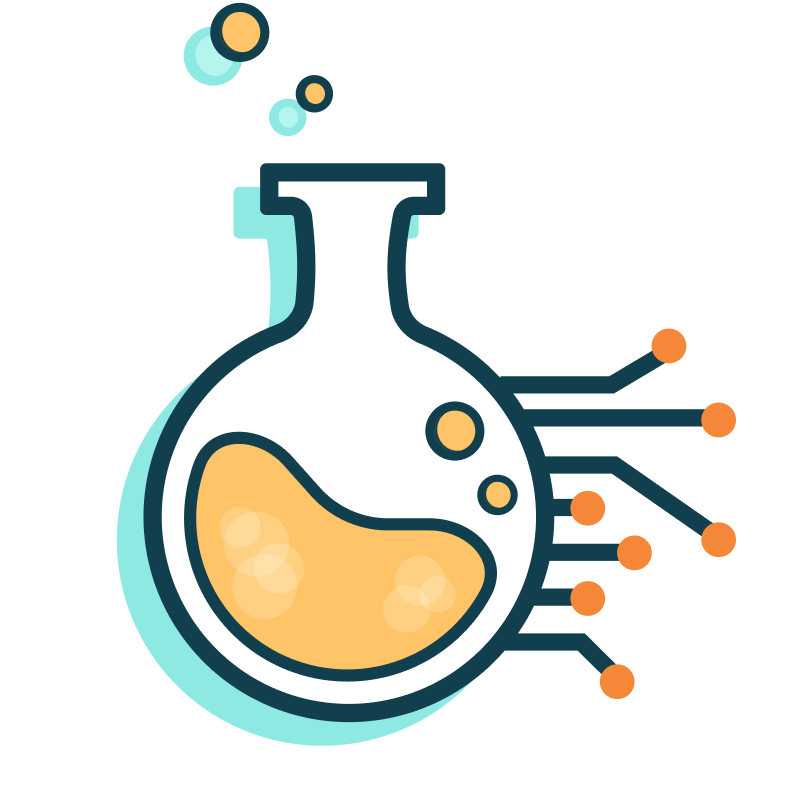
AI ALCHEMIST:
A master of transforming raw data into golden opportunities, using AI to create innovative solutions for market differentiation and business growth.
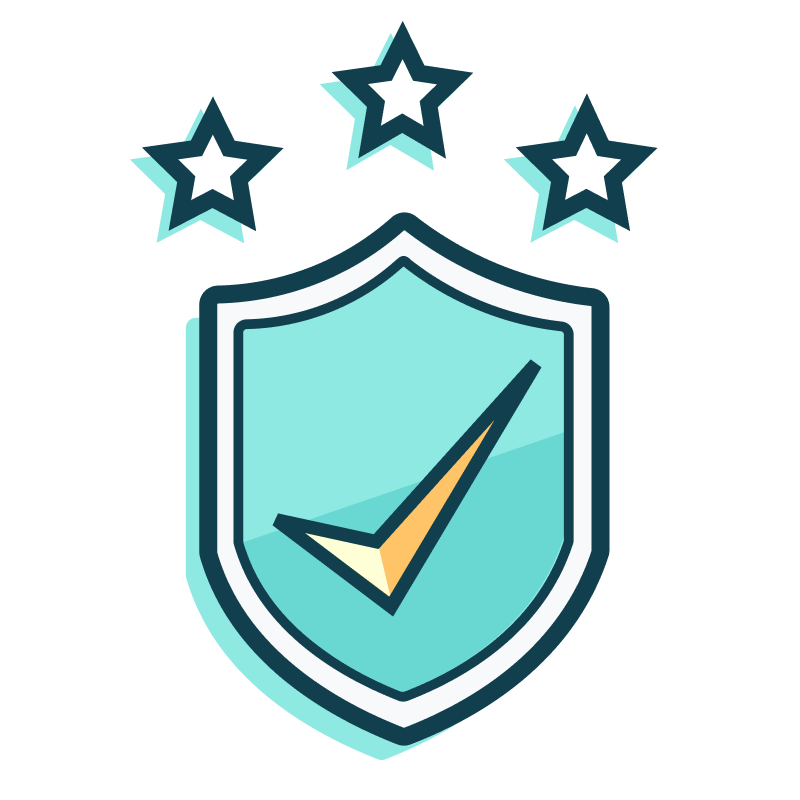
COMPLIANCE CRUSADER:
The unwavering enforcer of regulations and ethical standards, ensuring that data practices align with laws and best practices to protect both organizations and consumers.
Throughout this Knowledge Graph Series, we will dive deep into each persona, celebrating their unique missions and the challenges they face in the ever-evolving data landscape. You’ll learn how LifeGraph helps you tackle some of the biggest obstacles in data management.
Chapter 1:
Quality Commander
blast’s quality
commander saga
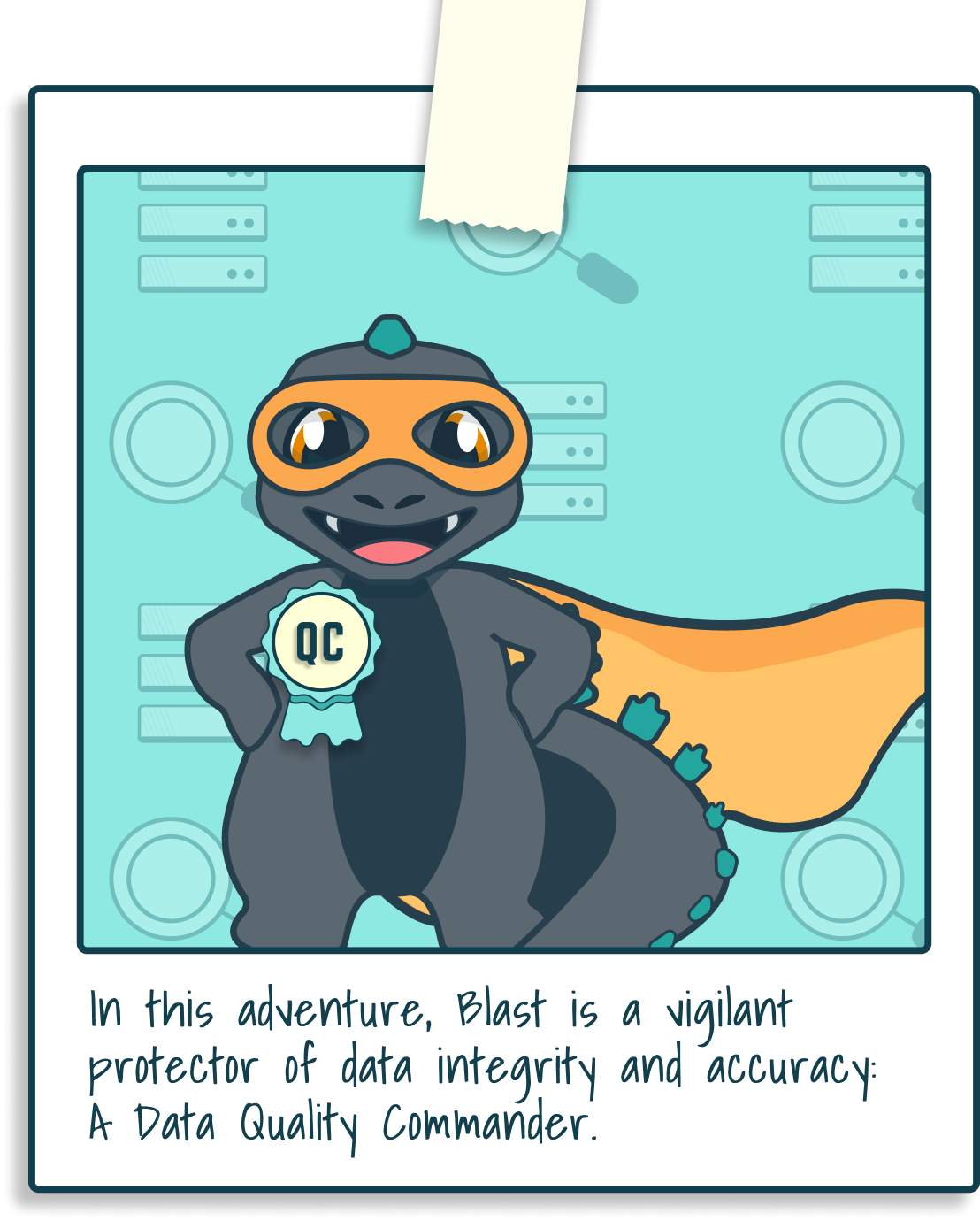
Passionate about making sure that every piece of data collected is spot-on and reliable, Blast knows the implications of poor quality on downstream operations—especially AI. With a keen eye for detail, Blast strives to root out errors and maintain high standards, creating a culture where teams can trust their data to make smart, informed decisions.
The Pursuit of AI-Ready Data
The first step is understanding what AI-ready data really means. AI-ready data isn’t just about being clean or structured; it’s about being representative, comprehensive, and aligned with specific AI use cases. Data should encapsulate all relevant patterns, errors, and outliers that an AI model might encounter in real-world applications.
AI-ready data is optimized for use in AI models and applications, defined by its cleanliness, organization, and relevance. The quality of data input into AI systems is directly linked to the accuracy and reliability of the results produced. As AI technology evolves, implementing strong data strategies becomes essential. AI-ready data requires greater variability and alignment with the AI’s learning objectives and often necessitates real-time or near-real-time quality.
The pursuit of AI-ready data must transcend the notion of mere data value. Data has to transform into a secure and reliable asset enriched with context (origin, lineage, changes over time, etc.) and trust (ownership, privacy, and security). This involves integrating active metadata rather than relying solely on static metadata, encompassing essential features such as longitudinal data and chain-of-custody attributes.
By taking these steps, organizations can develop AI-ready data and address fundamental data quality issues, such as incompleteness, duplication, inconsistent formats, and poor labeling, that have long been challenges in data management.
how LifeGraph helps:
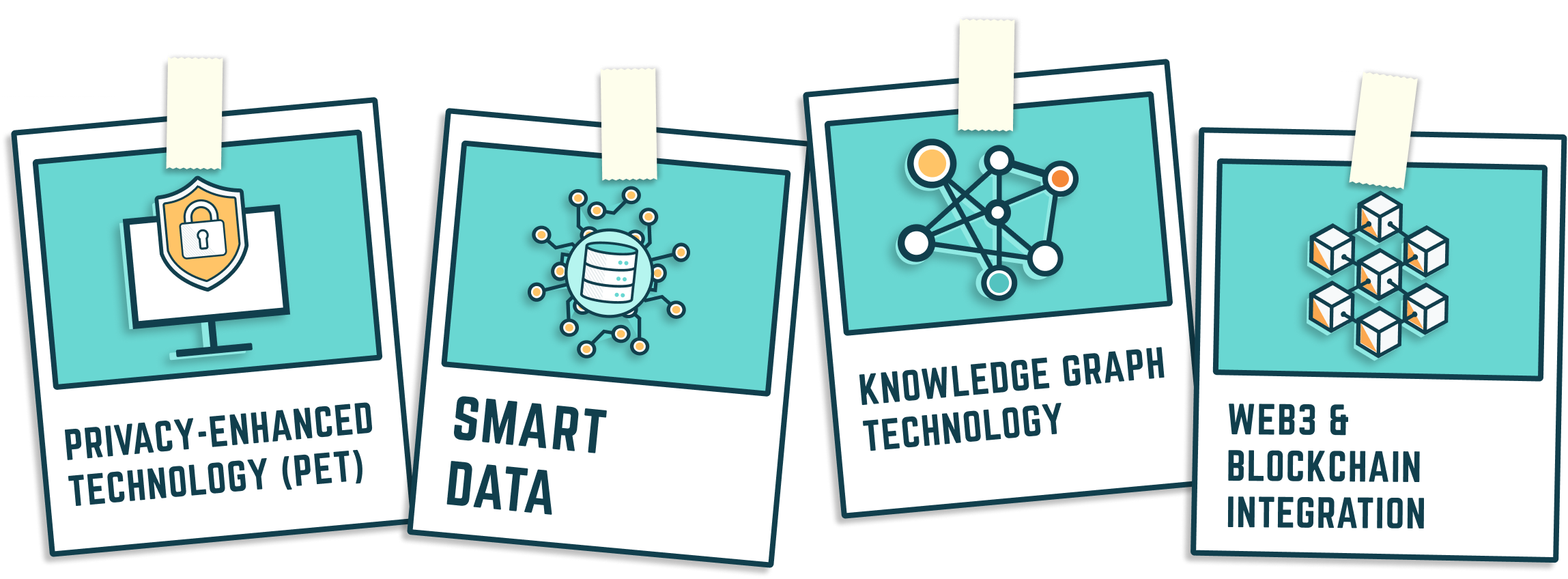
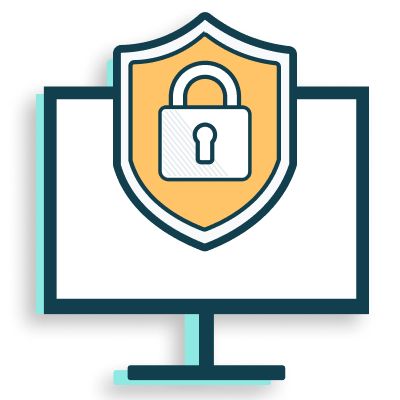
Privacy-Enhanced Technology (PET):
LifeGraph’s privacy-enhancing technology protects personal data in untrusted environments. This essential capability addresses evolving privacy laws and growing consumer concerns. By using various privacy protection techniques, LifeGraph enables valuable data insights while ensuring compliance with regulations.
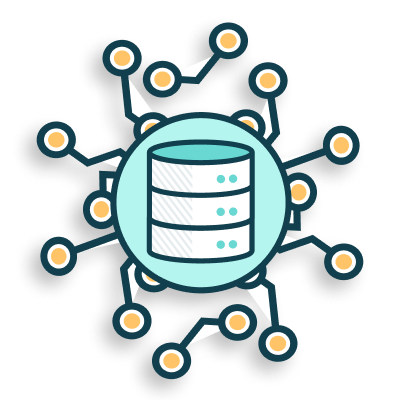
Smart Data:
LifeGraph connects data and transforms it into Smart Data. Smart Data is privacy-enhanced data enriched with trust (privacy, security, and ownership) and context (origin, lineage, changes over time, etc.), making data inherently more valuable for AI applications.
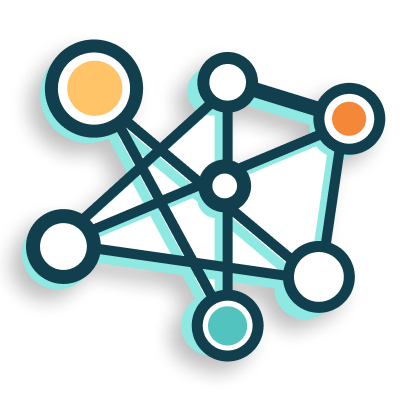
Knowledge graph technology:
LifeGraph uses knowledge graphs to store data and the relationships between data points, enhancing data quality by ensuring relevance and accuracy. This relational understanding is pivotal for AI, especially LLMs, which thrive on interconnected data for better contextual understanding.
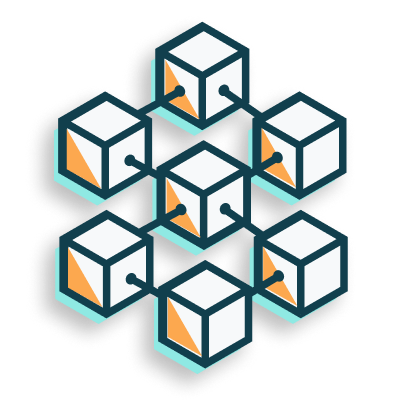
WEB3 & BLOCKCHAIN INTEGRATION:
By leveraging blockchain, LifeGraph ensures data provenance, security, and granular ownership controls which is foundational for trust in data. This integration also supports privacy-enhancing techniques crucial for sensitive data like healthcare or personal information. You can learn more in our previous Knowledge Burst: How a Web3 Data Fabric Can Help You Leapfrog the Market.
Data Governance
Data governance is critical to maintaining data quality. The framework must include policies for data quality, stewardship roles, intellectual property protection, and compliance with regulations. This framework should ensure that data quality is managed throughout its lifecycle and the entire data ecosystem. The only approach to managing this aspect of data quality is to implement a distributed governance mechanism that can be enforced in real-time or near real-time. That means governance rules must be embedded with the data transactional and access mechanisms.
Data governance must go beyond an administration function and extend to data lineage and provenance. The data ecosystem must be able to implement systems for tracking data lineage to understand where data comes from, how it’s transformed, and its quality throughout its journey. This is crucial for AI models, as understanding data origins can affect model reliability.
how LifeGraph helps:
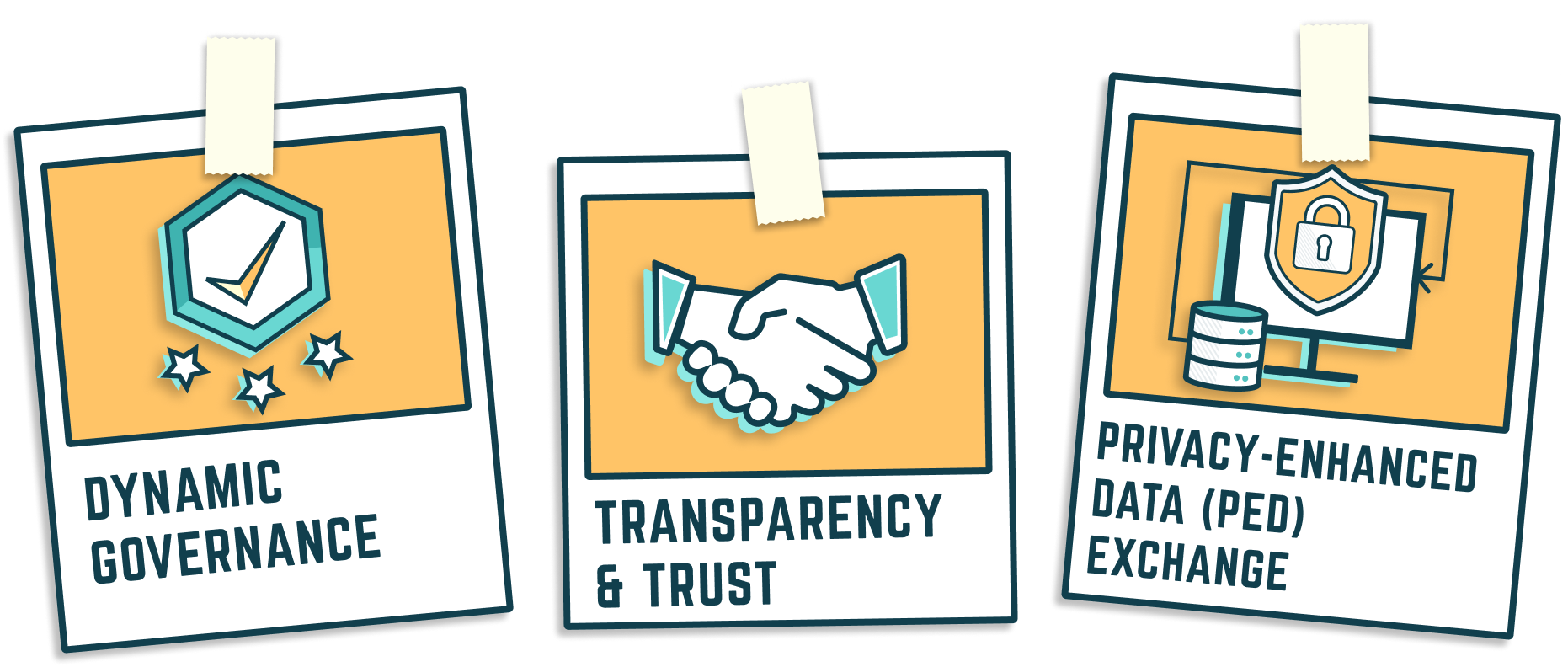
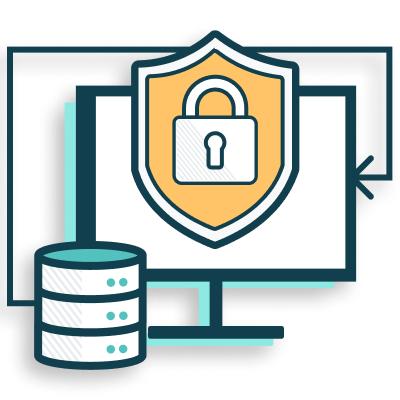
privacy-enhanced data (ped) exchange:
LifeGraph’s privacy-preserving infrastructure leverages blockchain services to empower organizations to securely share data across partners while maintaining control over who accesses what information. Ownership is assigned to each data asset using consent contracts, ensuring data is only shared if the owner consents.

dynamic governance:
LifeGraph employs blockchain technology to orchestrate how data moves throughout your organization. Consent Contracts ensure data can only be shared if the owner consents. Smart Contracts are self-executing agreements with the terms directly written into code and recorded on the blockchain. This automation ensures that data governance policies are enforced consistently without the need for intermediaries. For instance, they can automatically execute actions based on predefined conditions, ensuring compliance with regulations and organizational policies.
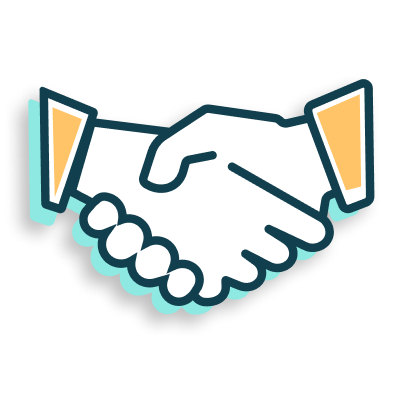
transparency & trust:
LifeGraph uses a decentralized ledger system to record all data transactions transparently and immutably, creating a reliable audit trail that stakeholders can trust. Moreover, Knowledge Graphs allow for visualizing data relationships, enhancing transparency and fostering better data governance. This solid foundation is crucial for ensuring high data quality in AI applications.
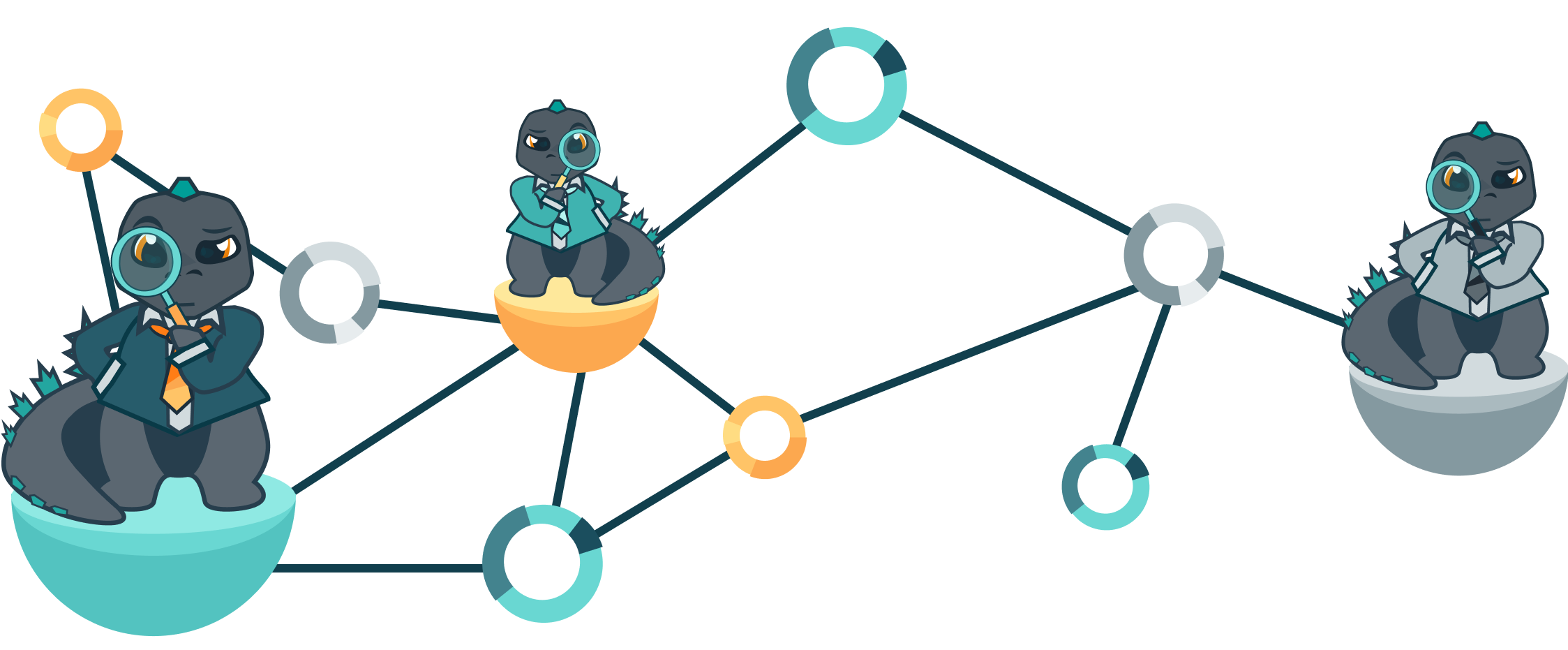
Continuous Data Quality Monitoring
Given AI’s need for up-to-date data, the Quality Commander must strive to implement continuous monitoring systems that alert when data quality drops below acceptable thresholds, affecting AI model performance. These mechanisms must be implemented at least at two critical points within the broader data ecosystem.
The first is at the ingestion point. At this point, organizations can establish a set of rules and parameters based on the targeted quality level.
It is at this point where AI-capable data pipelines and APIs are very advantageous, and given the monotonous detail required to perform this task, it is a perfect place for automated AI agents.
The second would be at the point of data consumption, where organizations can establish mechanisms to capture AI models’ performance feedback to inform data quality processes, creating a loop where data quality improvements directly enhance AI outcomes.
how LifeGraph helps:
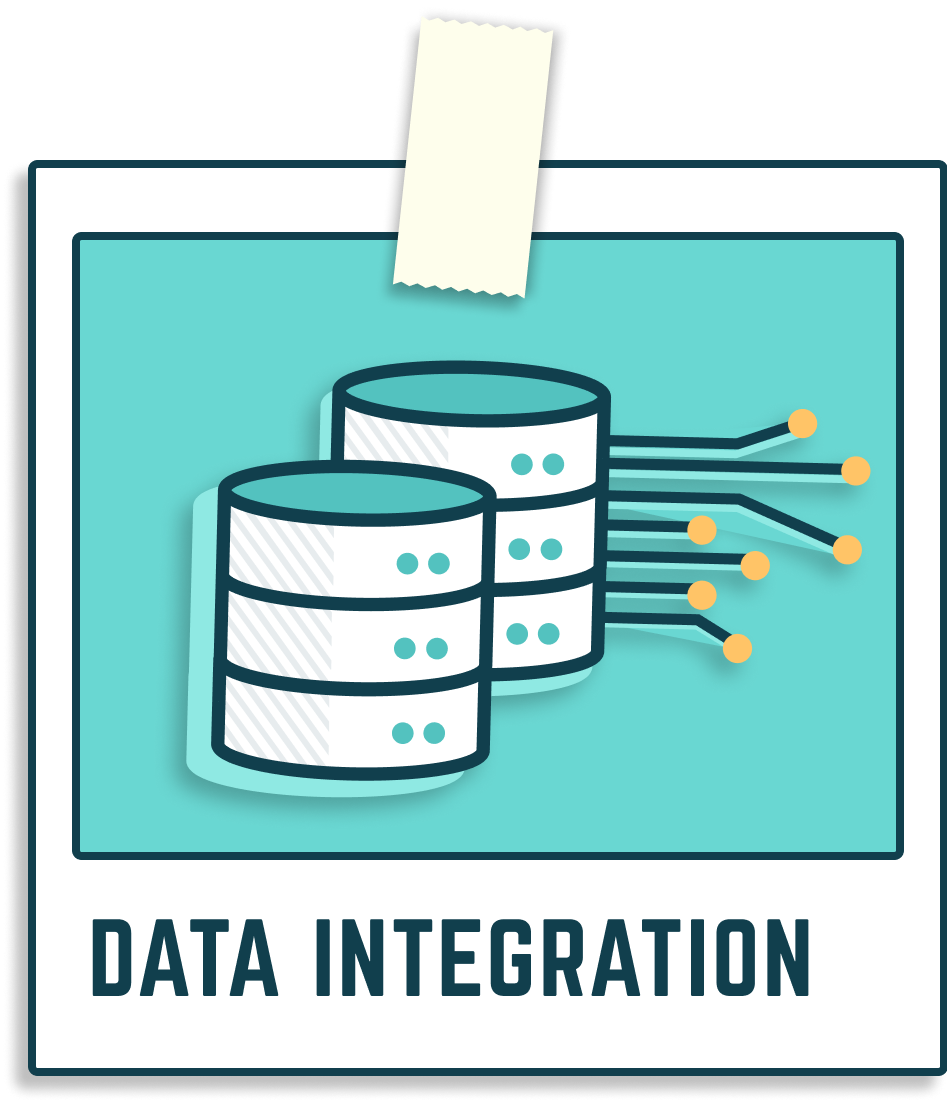
DATA integration:
LifeGraph connects all your systems and data sources through REST APIs and ingests data through adaptive data pipelines that include built-in data validation and cleansing steps, which ensures that only high-quality data is ingested and processed.
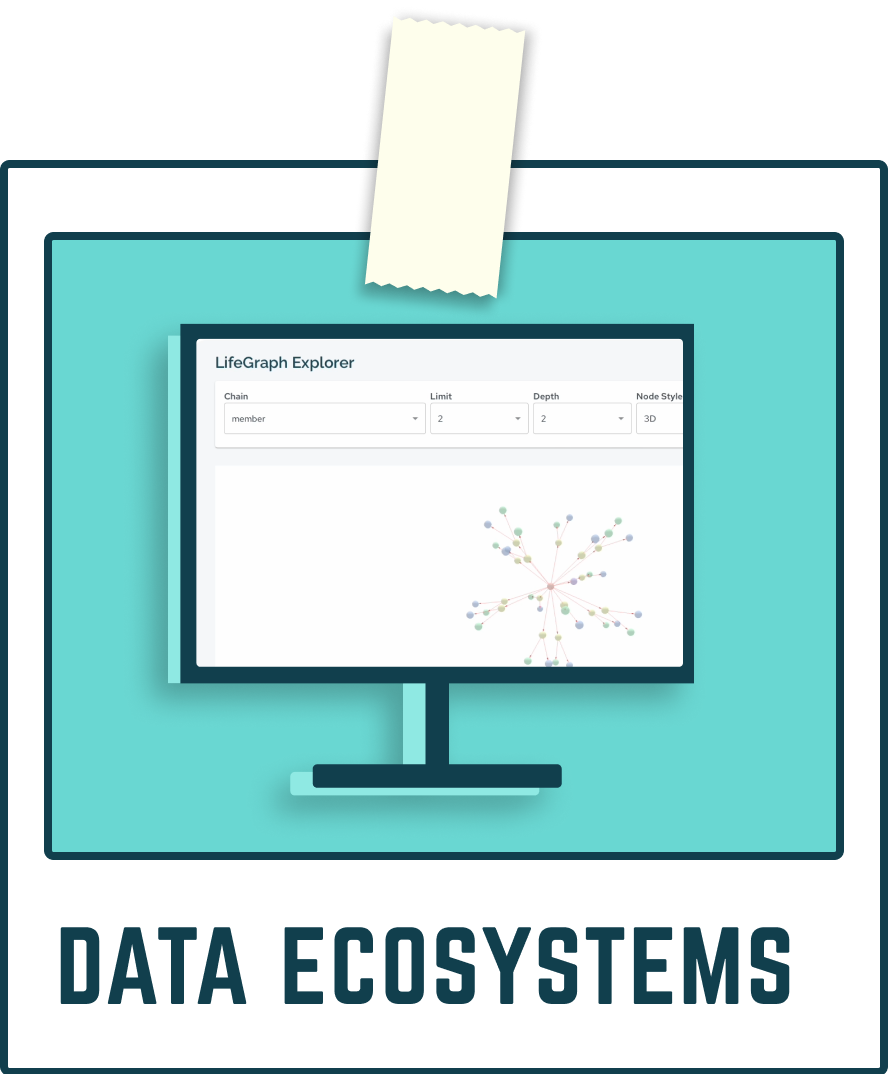
DATA ECOSYSTEMS:
With LifeGraph, data quality isn’t static. As new data from across your business ecosystem is integrated, the graph evolves, automatically updating relationships and ensuring ongoing data quality.
Data Quality as Part of AI Model Development
A Quality Commander knows quality needs to be an integral part of AI model development. The risks associated with poor-quality data in AI models are substantial, affecting everything from accuracy and bias to costs and overall effectiveness. Ensuring high-quality data is crucial for the successful deployment of AI technologies.
It is important for data teams to incorporate data quality checks as part of the training pipeline. This could mean filtering out low-quality data or weighting it based on quality. Additionally, when assessing the AI model’s effectiveness, teams need to understand the sensitivity of the AI model to data quality issues. Some models might perform poorly with slight data degradation, requiring higher data quality standards. This includes when data is looped back on itself or when the model is only trained on synthetic data with no mechanism to adjust when exposed to real-world data assets.
how LifeGraph helps:
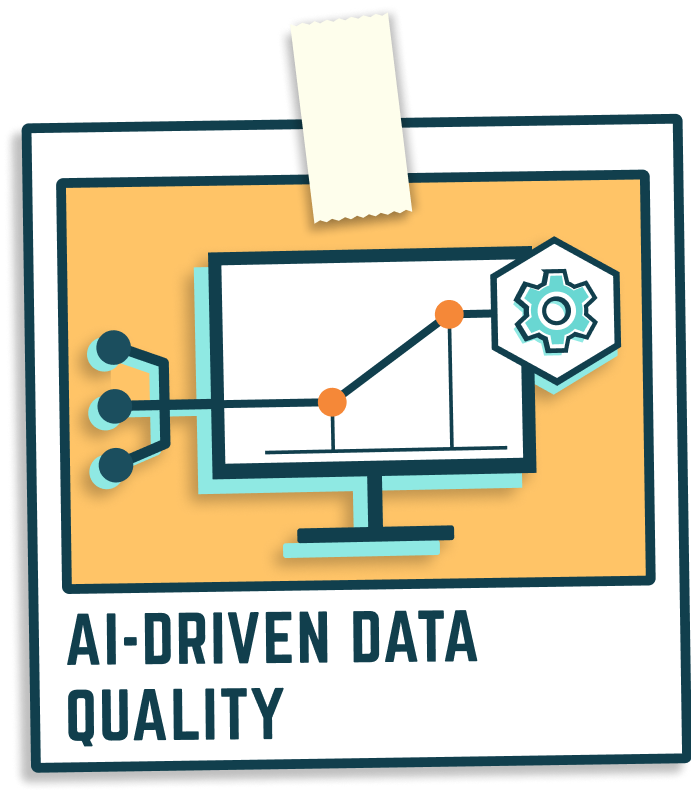
ai-driven data quality:
LifeGraph enables AI models to understand and infer from data in ways that traditional databases cannot, leading to more nuanced data quality checks based on AI’s understanding of data patterns and anomalies.
Cultural Shift & Training
Leadership is vital to a long-term sustainable data quality initiative. They must promote a culture of data literacy where all employees understand the importance of data quality for AI. There needs to be training on handling data, reporting quality issues, and understanding the impact of their data contributions.
They must also encourage a bottom-up approach where data users at all levels can contribute to data quality improvements through reporting mechanisms or direct data curation.
Data quality governance needs to be pushed to the edge and function more as a distributed autonomous organization vs a centralized bureaucracy.
how LifeGraph helps:
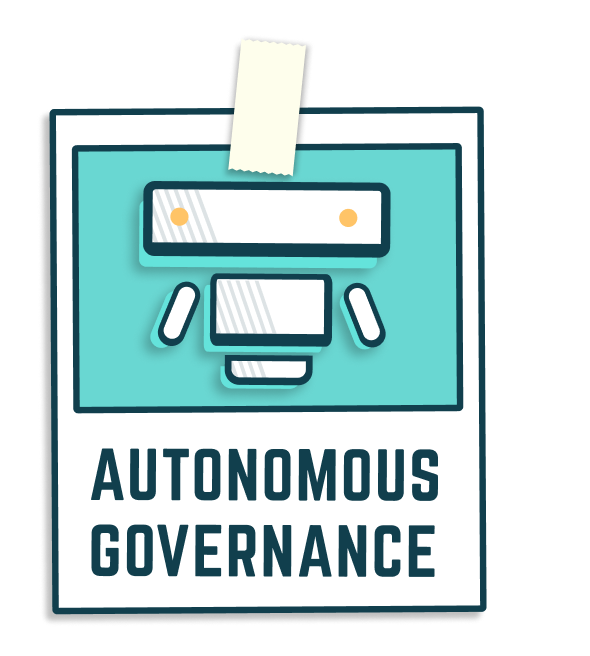
AUTONOMOUS GOVERNANCE:
LifeGraph leverages Consent Contracts to establish governance rules, significantly minimizing manual oversight. This ensures seamless and consistent governance maintenance.
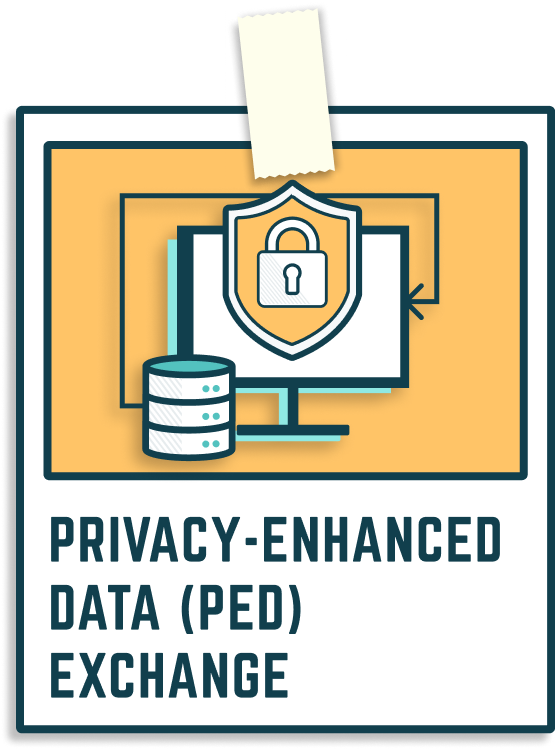
privacy-enhanced data (ped) exchange:
LifeGraph’s privacy-preserving infrastructure leverages blockchain services to empower organizations to securely share data across partners while maintaining control over who accesses what information. Ownership is assigned to each data asset using consent contracts, ensuring data is only shared if the owner consents.
Scalability & Adaptability
Data quality initiatives must scale with the enterprise’s data growth. That is why data quality metrics, standards, and enforcement must be part of the data flow and not implemented as an external solution.
As AI technologies evolve, so will the requirements for data. The initiative should be adaptable and ready to incorporate new data quality metrics, data structures, or standards that future AI models might demand. The only way to do this is by creating data assets vs data stores. We recently wrote about how to make this happen in this Knowledge Burst: The Next Wave: Data Stores to Data Ecosystems & Data Flows.
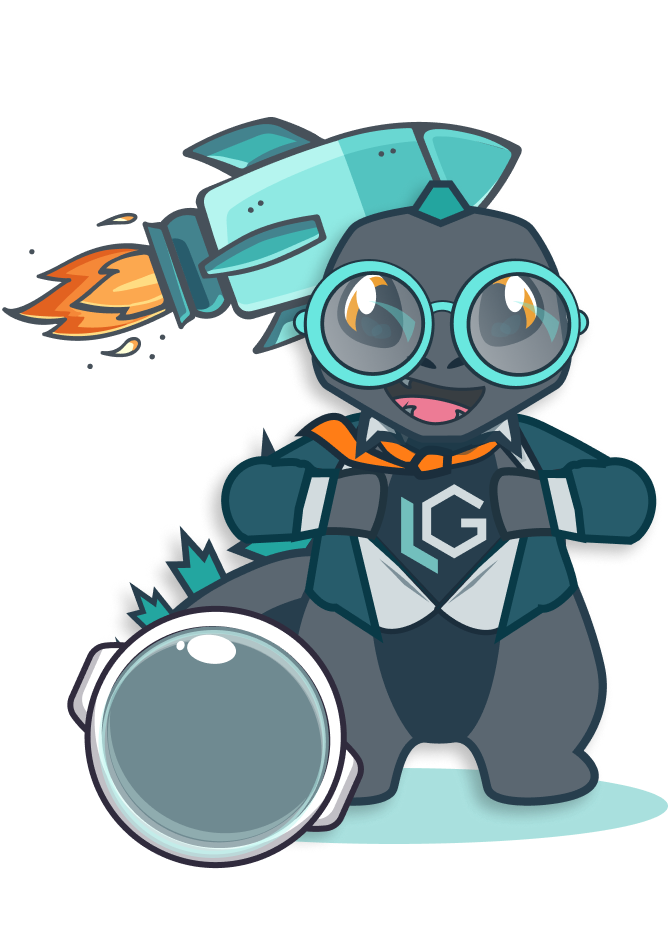
how LifeGraph helps:
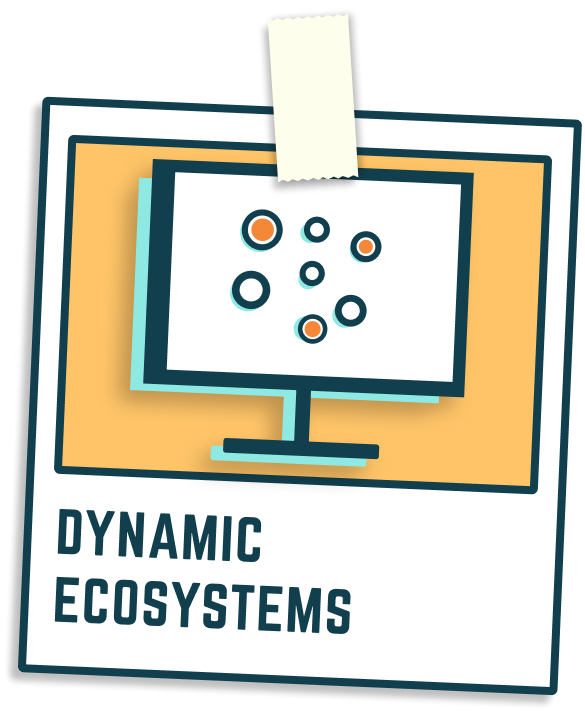
dynamic ecosystems:
LifeGraph empowers dynamic ecosystems capable of incorporating new data sources and evolving as organizational needs change. No need to write new data dictionaries and redeploy.
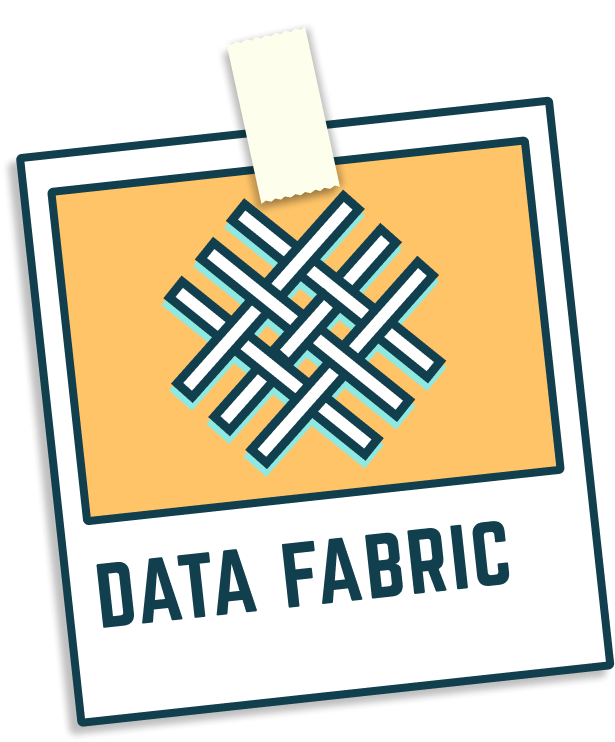
data fabric:
LifeGraph delivers a data fabric, providing a unified view of data across an organization’s ecosystem and ensuring uniform data quality management.
A Successful Quality Adventure
The evolution of data quality management, driven by adaptive APIs and platforms like BurstIQ’s LifeGraph, sets the stage for a new era of data-driven AI. By leveraging Smart Data, LifeGraph enhances data quality and builds trust and context into data assets, making them indispensable for organizations aiming to thrive in the AI-driven future. As we progress, data quality assurance will be a competitive advantage and a necessity for staying relevant in an increasingly data-centric world.
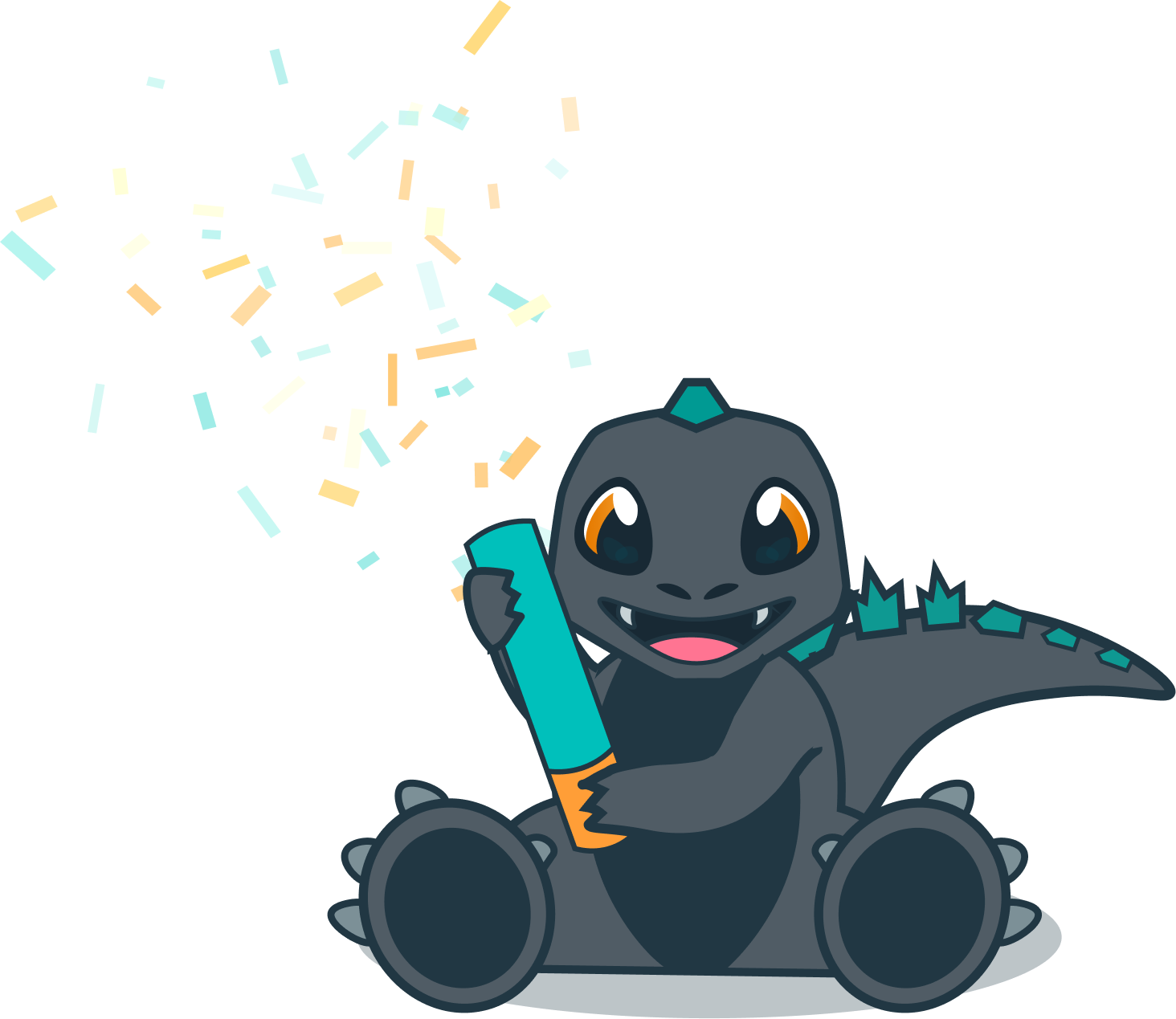
Knowledge Burst Series:
Conquering Data Chaos to Win in an AI World
In a world increasingly driven by data, the landscape can often feel chaotic and overwhelming. The urgency to implement AI both successfully and ethically has never been greater. Join us in this Knowledge Graph Series as we embark on an exhilarating adventure with our hero, Blast. Equipped with his powerful tool, LifeGraph®—the next generation of data management—Blast tackles the chaotic landscape of data. Prepare to explore the intricacies of data-driven innovation and discover how to harness its true potential!
At BurstIQ, we make data your superpower.
We’ve found our customers identify with the following data personas:
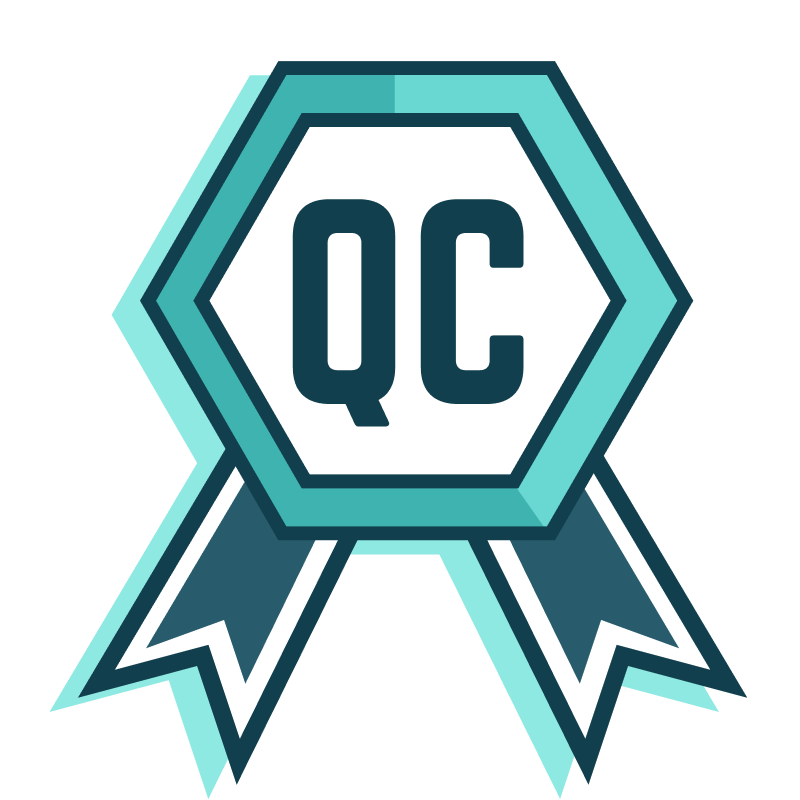
Quality Commander:
The relentless champion of data integrity, tirelessly working to ensure that information is not just abundant but also accurate and actionable.
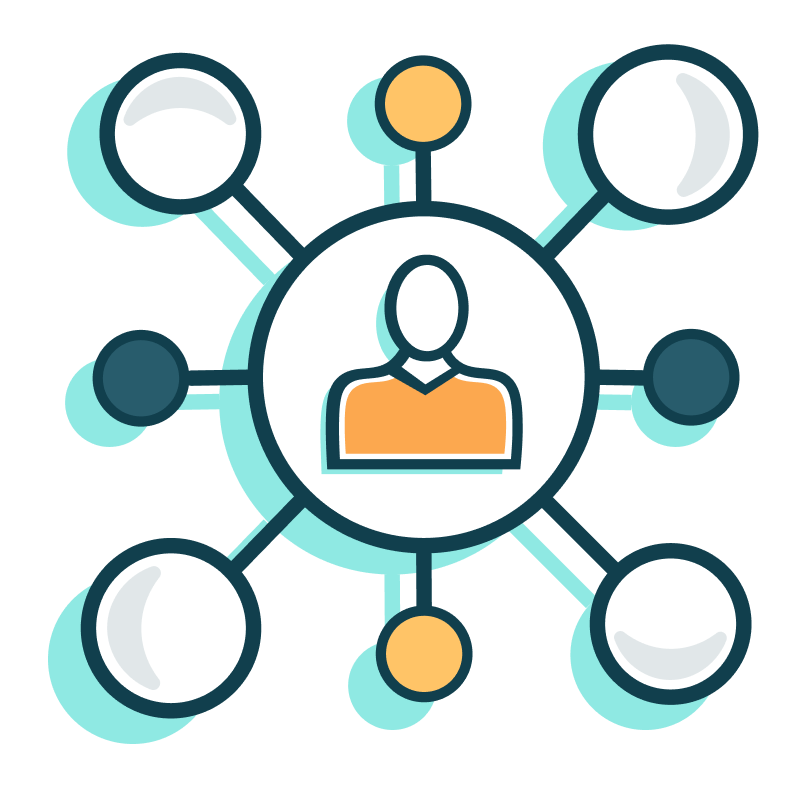
THE GREAT UNIFIER:
The collaborative force that bridges gaps between silos, systems, departments, and disciplines, fostering a culture of data-sharing and teamwork.
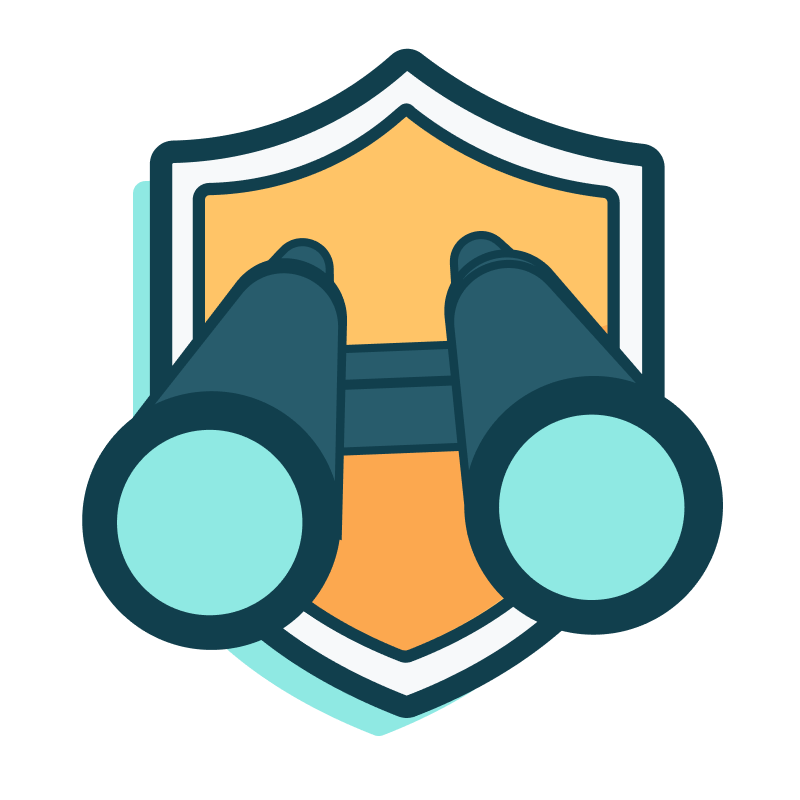
GUARDIAN OF PRIVACY:
The vigilant protector of personal information, committed to safeguarding privacy while navigating the complexities of data collection and usage.
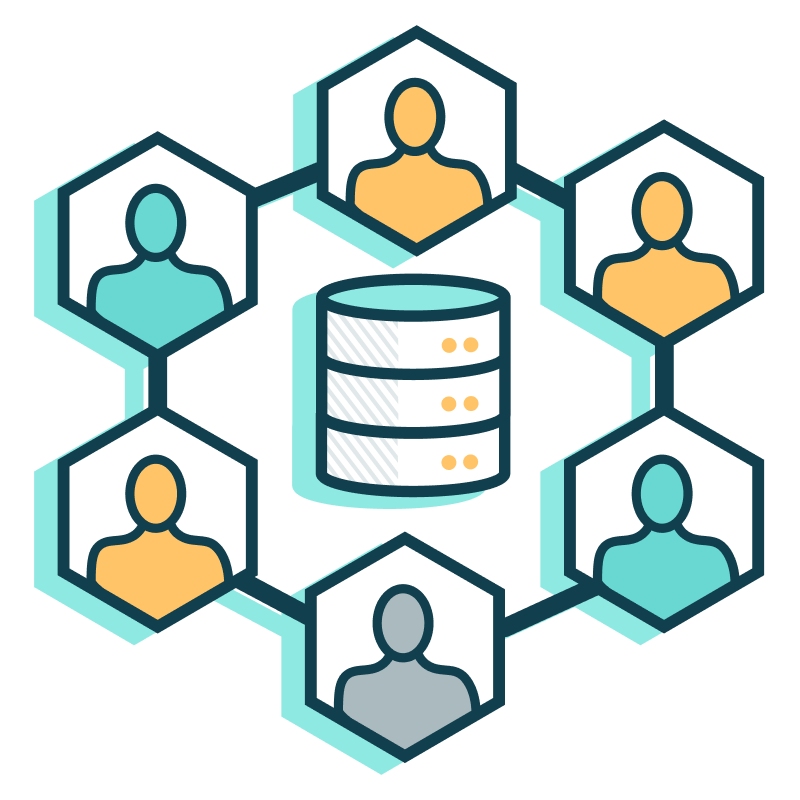
DATA DEMOCRATIZER:
The passionate advocate for accessibility, striving to empower everyone in their business ecosystem with the knowledge and tools to leverage data for their benefit.
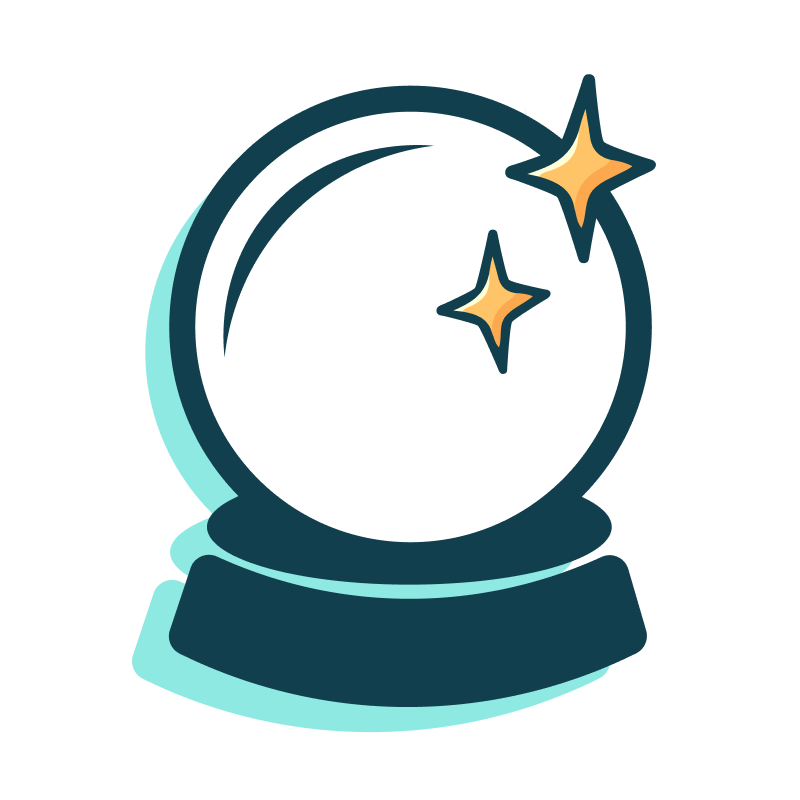
AI Activator:
The visionary architect of intelligent systems, harnessing the power of artificial intelligence, machine learning, and generative AI as a force multiplier to enhance team productivity, personalize engagements, and accelerate the pace of innovation.
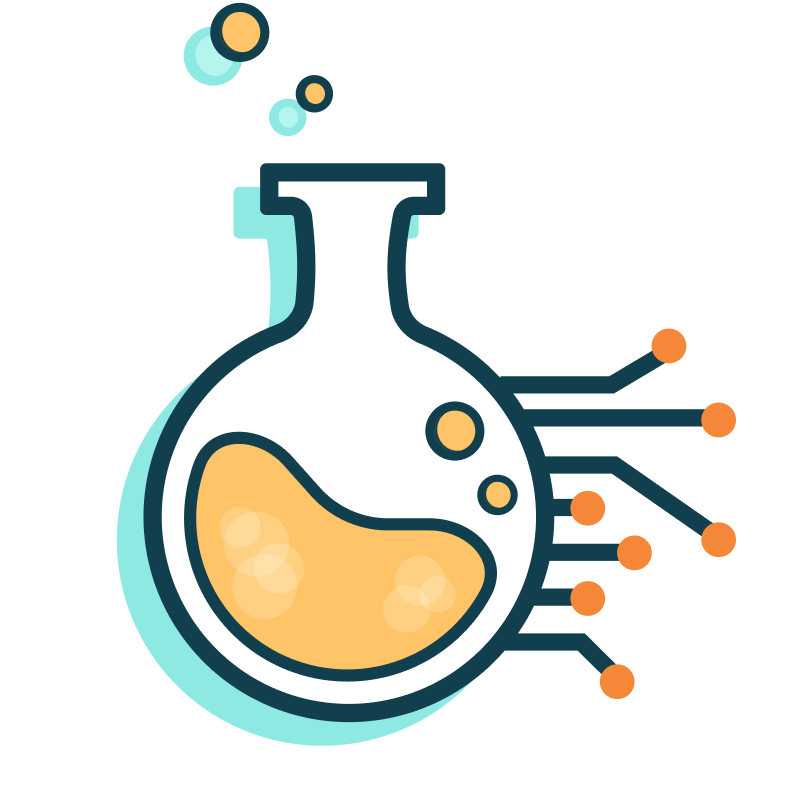
AI ALCHEMIST:
A master of transforming raw data into golden opportunities, using AI to create innovative solutions for market differentiation and business growth.
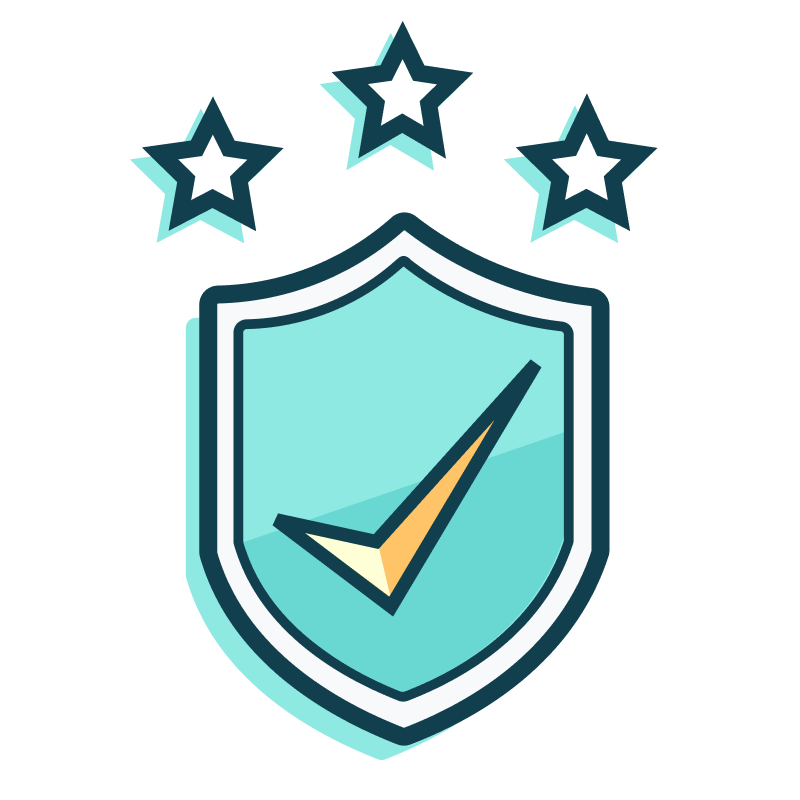
COMPLIANCE CRUSADER:
The unwavering enforcer of regulations and ethical standards, ensuring that data practices align with laws and best practices to protect both organizations and consumers.
Throughout this Knowledge Graph Series, we will dive deep into each persona, celebrating their unique missions and the challenges they face in the ever-evolving data landscape. You’ll learn how LifeGraph helps you tackle some of the biggest obstacles in data management.
Chapter 1:
Quality Commander
blast’s quality commander saga
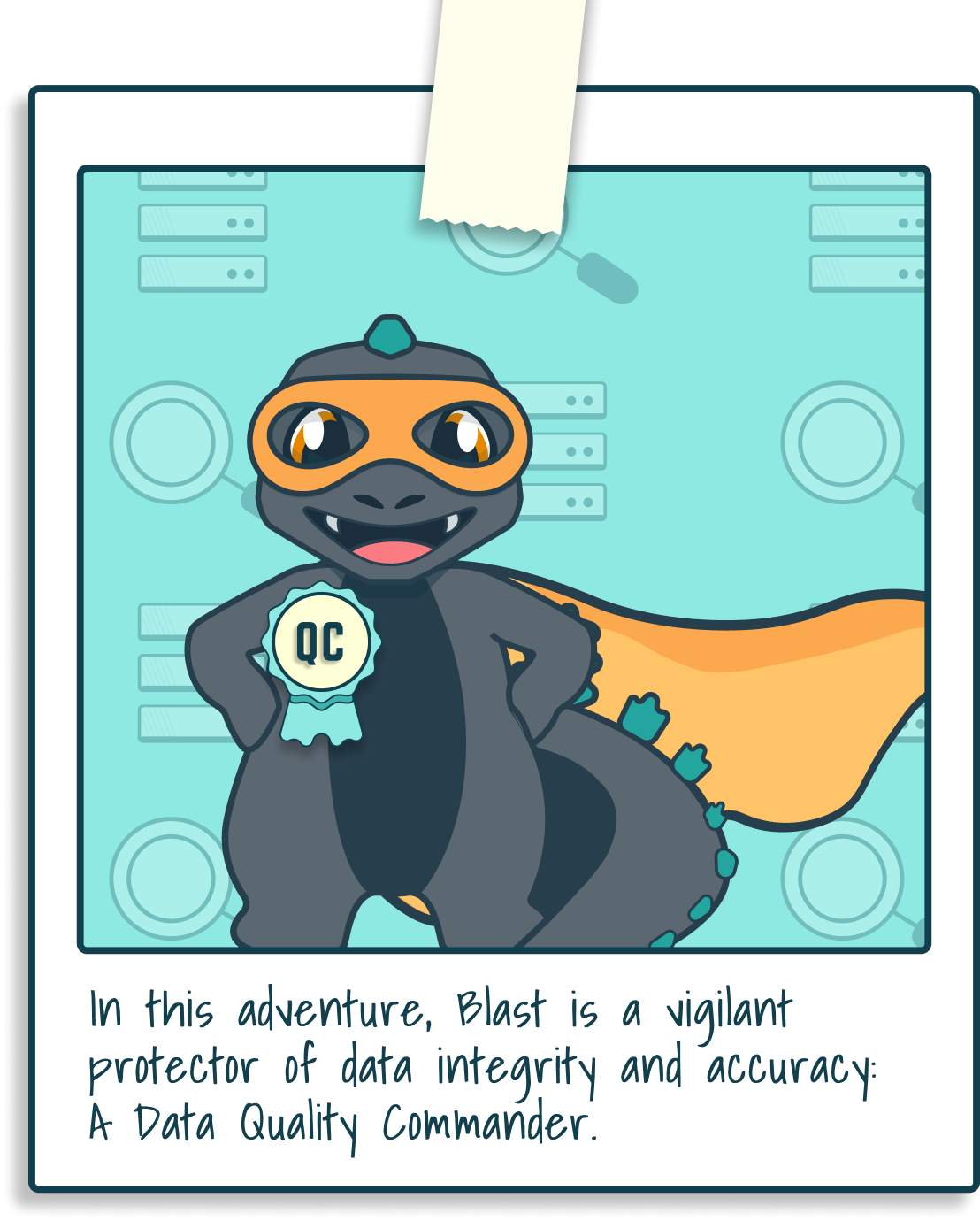
Passionate about making sure that every piece of data collected is spot-on and reliable, Blast knows the implications of poor quality on downstream operations—especially AI. With a keen eye for detail, Blast strives to root out errors and maintain high standards, creating a culture where teams can trust their data to make smart, informed decisions.
The Pursuit of AI-Ready Data
The first step is understanding what AI-ready data really means. AI-ready data isn’t just about being clean or structured; it’s about being representative, comprehensive, and aligned with specific AI use cases. Data should encapsulate all relevant patterns, errors, and outliers that an AI model might encounter in real-world applications.
AI-ready data is optimized for use in AI models and applications, defined by its cleanliness, organization, and relevance. The quality of data input into AI systems is directly linked to the accuracy and reliability of the results produced. As AI technology evolves, implementing strong data strategies becomes essential. AI-ready data requires greater variability and alignment with the AI’s learning objectives and often necessitates real-time or near-real-time quality.
The pursuit of AI-ready data must transcend the notion of mere data value. Data has to transform into a secure and reliable asset enriched with context (origin, lineage, changes over time, etc.) and trust (ownership, privacy, and security). This involves integrating active metadata rather than relying solely on static metadata, encompassing essential features such as longitudinal data and chain-of-custody attributes.
By taking these steps, organizations can develop AI-ready data and address fundamental data quality issues, such as incompleteness, duplication, inconsistent formats, and poor labeling, that have long been challenges in data management.
how LifeGraph helps:
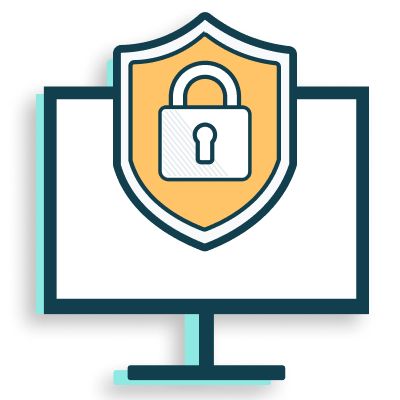
Privacy-Enhanced Technology (PET):
LifeGraph’s privacy-enhancing technology protects personal data in untrusted environments. This essential capability addresses evolving privacy laws and growing consumer concerns. By using various privacy protection techniques, LifeGraph enables valuable data insights while ensuring compliance with regulations.
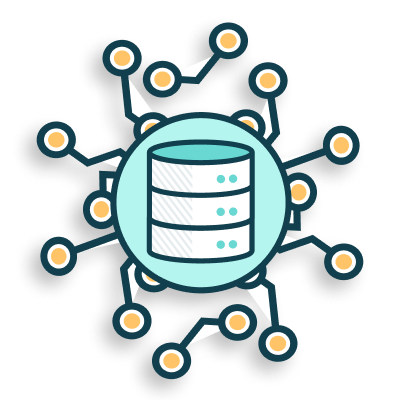
Smart Data:
LifeGraph connects data and transforms it into Smart Data. Smart Data is privacy-enhanced data enriched with trust (privacy, security, and ownership) and context (origin, lineage, changes over time, etc.), making data inherently more valuable for AI applications.
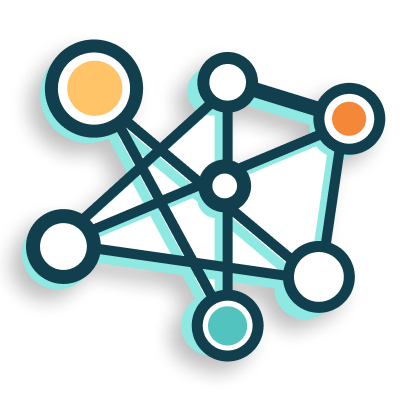
Knowledge graph technology:
LifeGraph uses knowledge graphs to store data and the relationships between data points, enhancing data quality by ensuring relevance and accuracy. This relational understanding is pivotal for AI, especially LLMs, which thrive on interconnected data for better contextual understanding.
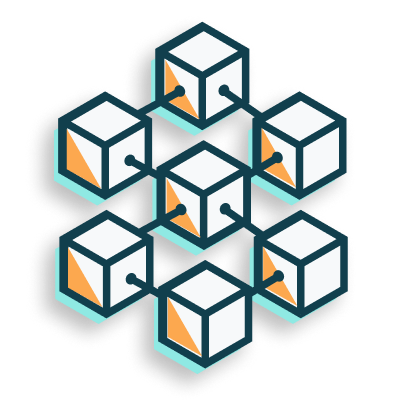
WEB3 & BLOCKCHAIN INTEGRATION:
By leveraging blockchain, LifeGraph ensures data provenance, security, and granular ownership controls which is foundational for trust in data. This integration also supports privacy-enhancing techniques crucial for sensitive data like healthcare or personal information. You can learn more in our previous Knowledge Burst: How a Web3 Data Fabric Can Help You Leapfrog the Market.
Data Governance
Data governance is critical to maintaining data quality. The framework must include policies for data quality, stewardship roles, intellectual property protection, and compliance with regulations. This framework should ensure that data quality is managed throughout its lifecycle and the entire data ecosystem. The only approach to managing this aspect of data quality is to implement a distributed governance mechanism that can be enforced in real-time or near real-time. That means governance rules must be embedded with the data transactional and access mechanisms.
Data governance must go beyond an administration function and extend to data lineage and provenance. The data ecosystem must be able to implement systems for tracking data lineage to understand where data comes from, how it’s transformed, and its quality throughout its journey. This is crucial for AI models, as understanding data origins can affect model reliability.
how LifeGraph helps:
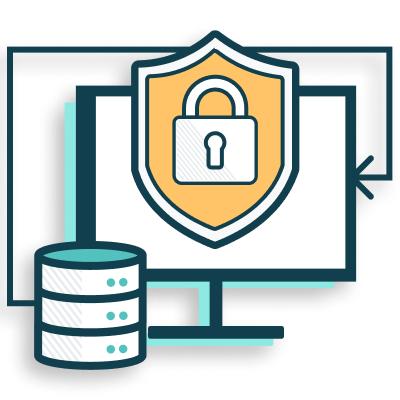
privacy-enhanced data (ped) exchange:
LifeGraph’s privacy-preserving infrastructure leverages blockchain services to empower organizations to securely share data across partners while maintaining control over who accesses what information. Ownership is assigned to each data asset using consent contracts, ensuring data is only shared if the owner consents.

dynamic governance:
LifeGraph employs blockchain technology to orchestrate how data moves throughout your organization. Consent Contracts ensure data can only be shared if the owner consents. Smart Contracts are self-executing agreements with the terms directly written into code and recorded on the blockchain. This automation ensures that data governance policies are enforced consistently without the need for intermediaries. For instance, they can automatically execute actions based on predefined conditions, ensuring compliance with regulations and organizational policies.
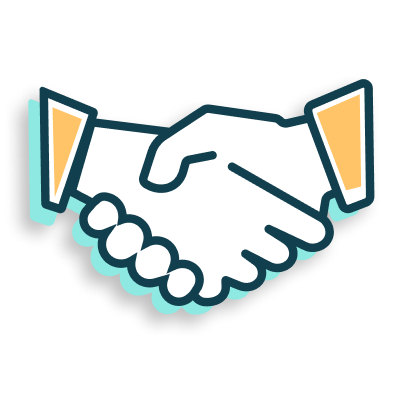
transparency & trust:
LifeGraph uses a decentralized ledger system to record all data transactions transparently and immutably, creating a reliable audit trail that stakeholders can trust. Moreover, Knowledge Graphs allow for visualizing data relationships, enhancing transparency and fostering better data governance. This solid foundation is crucial for ensuring high data quality in AI applications.
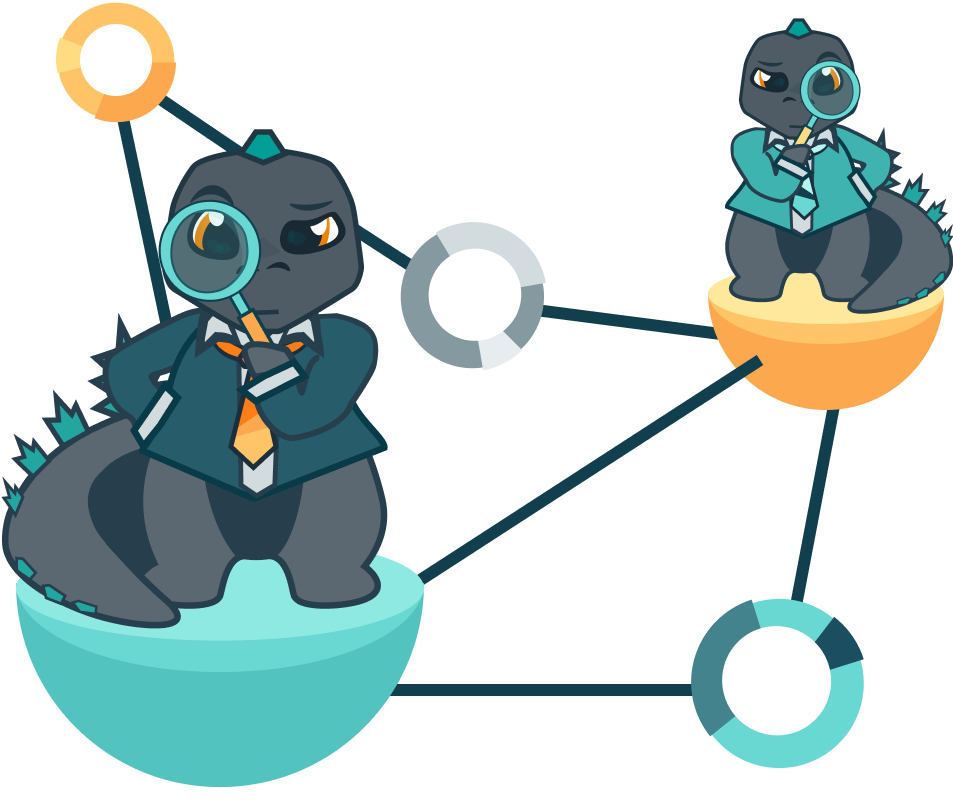
Continuous Data Quality Monitoring
Given AI’s need for up-to-date data, the Quality Commander must strive to implement continuous monitoring systems that alert when data quality drops below acceptable thresholds, affecting AI model performance. These mechanisms must be implemented at least at two critical points within the broader data ecosystem.
The first is at the ingestion point. At this point, organizations can establish a set of rules and parameters based on the targeted quality level.
It is at this point where AI-capable data pipelines and APIs are very advantageous, and given the monotonous detail required to perform this task, it is a perfect place for automated AI agents.
The second would be at the point of data consumption, where organizations can establish mechanisms to capture AI models’ performance feedback to inform data quality processes, creating a loop where data quality improvements directly enhance AI outcomes.
how LifeGraph helps:
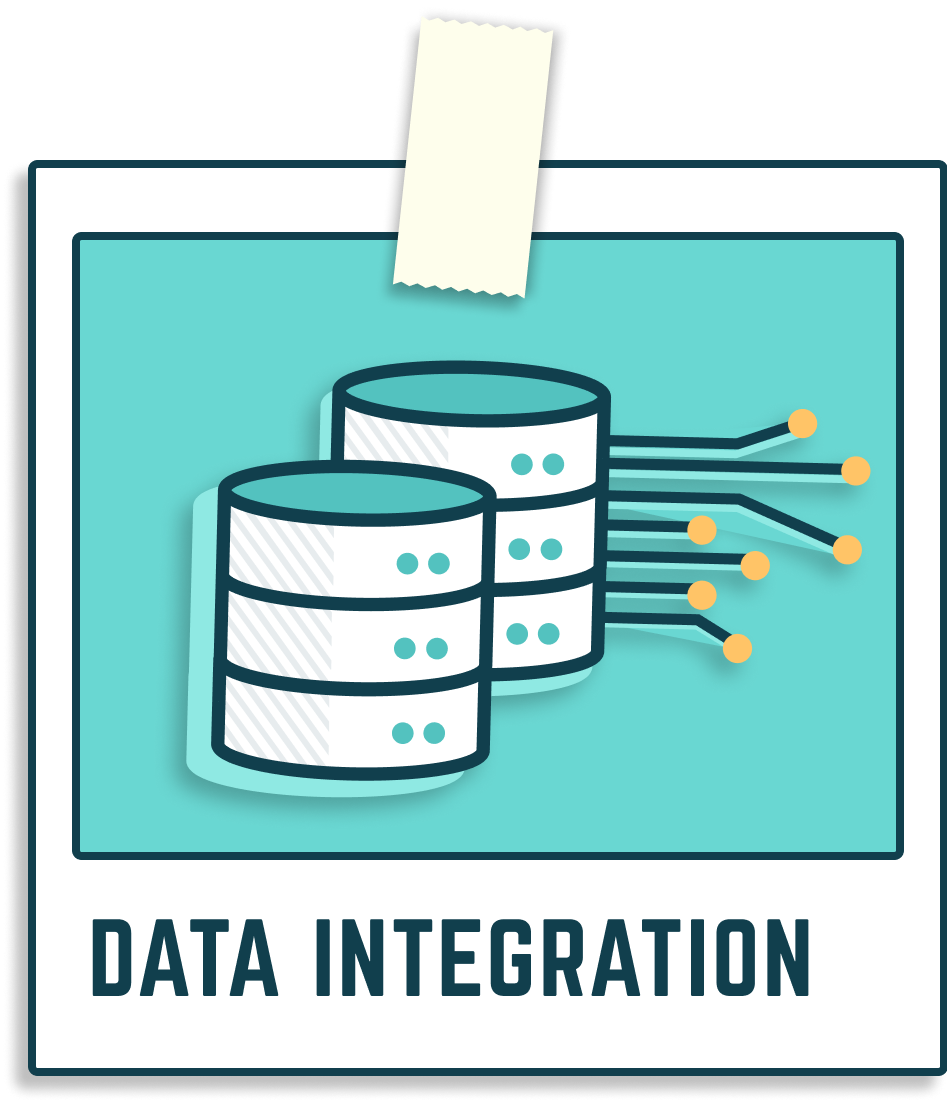
DATA integration:
LifeGraph connects all your systems and data sources through REST APIs and ingests data through adaptive data pipelines that include built-in data validation and cleansing steps, which ensures that only high-quality data is ingested and processed.
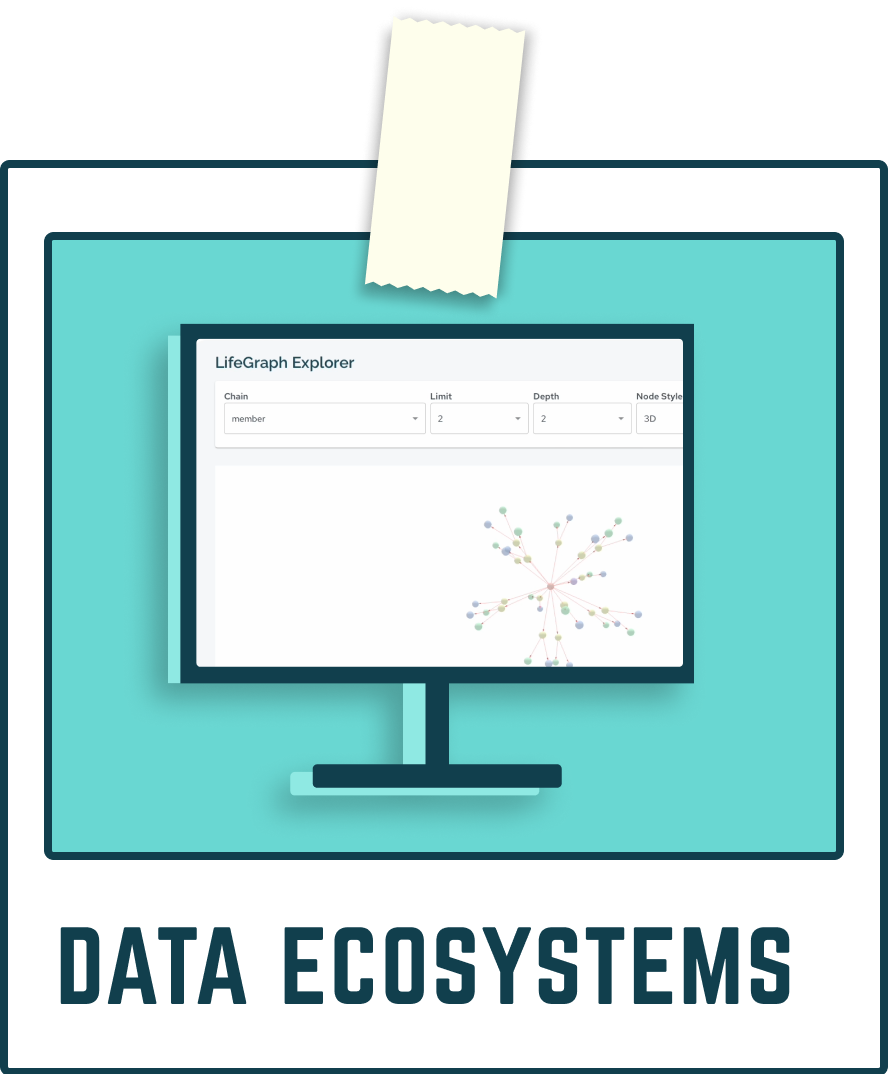
DATA ECOSYSTEMS:
With LifeGraph, data quality isn’t static. As new data from across your business ecosystem is integrated, the graph evolves, automatically updating relationships and ensuring ongoing data quality.
Data Quality as Part of AI Model Development
A Quality Commander knows quality needs to be an integral part of AI model development. The risks associated with poor-quality data in AI models are substantial, affecting everything from accuracy and bias to costs and overall effectiveness. Ensuring high-quality data is crucial for the successful deployment of AI technologies.
It is important for data teams to incorporate data quality checks as part of the training pipeline. This could mean filtering out low-quality data or weighting it based on quality. Additionally, when assessing the AI model’s effectiveness, teams need to understand the sensitivity of the AI model to data quality issues. Some models might perform poorly with slight data degradation, requiring higher data quality standards. This includes when data is looped back on itself or when the model is only trained on synthetic data with no mechanism to adjust when exposed to real-world data assets.
how LifeGraph helps:
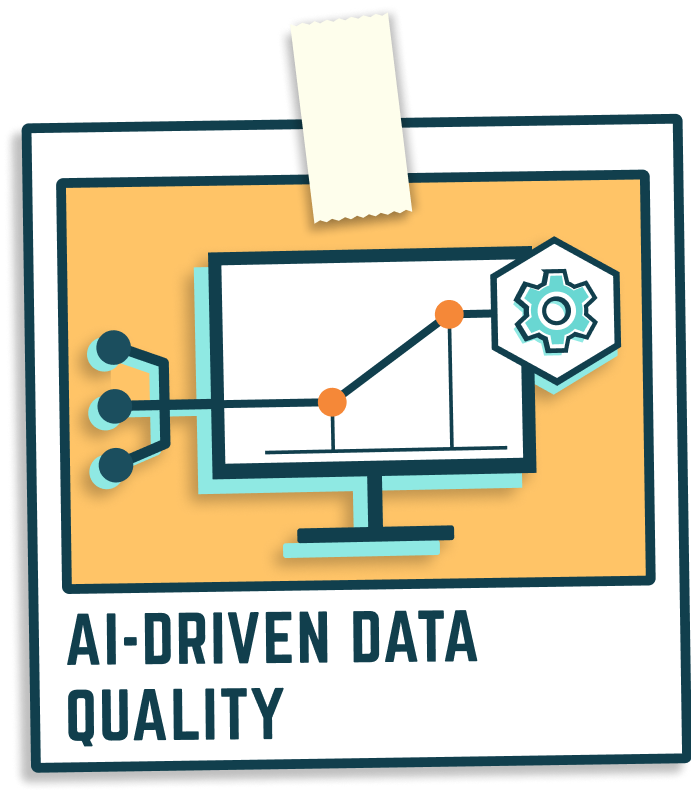
ai-driven data quality:
LifeGraph enables AI models to understand and infer from data in ways that traditional databases cannot, leading to more nuanced data quality checks based on AI’s understanding of data patterns and anomalies.
Cultural Shift & Training
Leadership is vital to a long-term sustainable data quality initiative. They must promote a culture of data literacy where all employees understand the importance of data quality for AI. There needs to be training on handling data, reporting quality issues, and understanding the impact of their data contributions.
They must also encourage a bottom-up approach where data users at all levels can contribute to data quality improvements through reporting mechanisms or direct data curation.
Data quality governance needs to be pushed to the edge and function more as a distributed autonomous organization vs a centralized bureaucracy.
how LifeGraph helps:
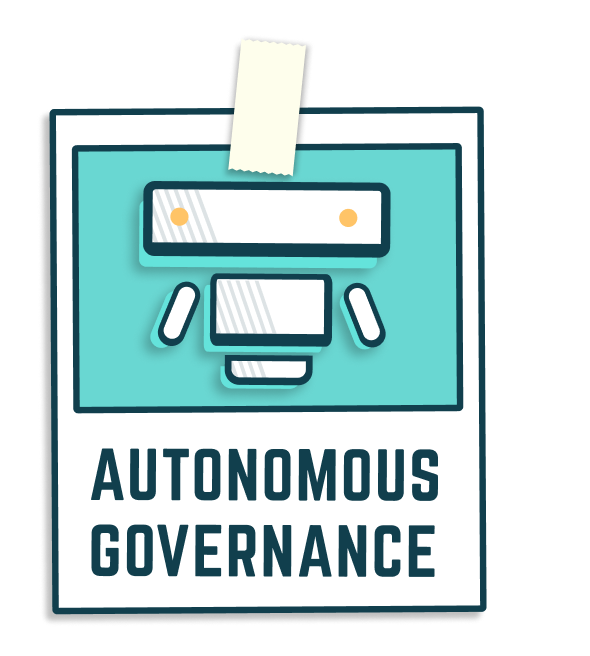
AUTONOMOUS GOVERNANCE:
LifeGraph leverages Consent Contracts to establish governance rules, significantly minimizing manual oversight. This ensures seamless and consistent governance maintenance.
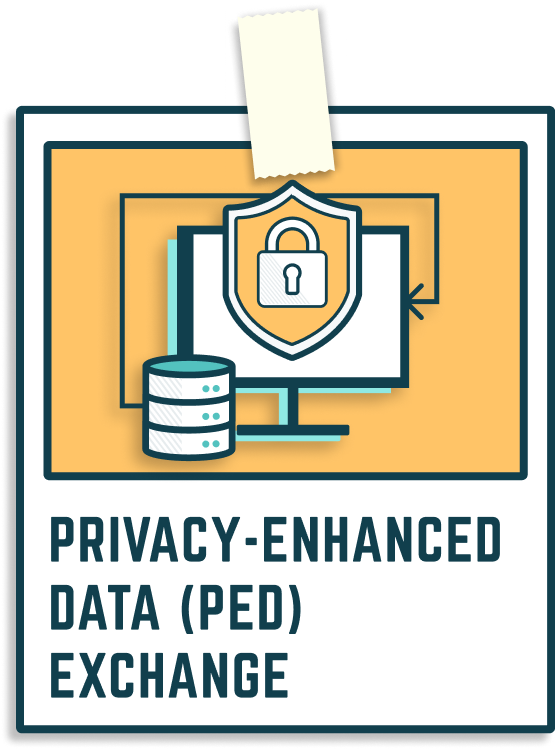
privacy-enhanced data (ped) exchange:
LifeGraph’s privacy-preserving infrastructure leverages blockchain services to empower organizations to securely share data across partners while maintaining control over who accesses what information. Ownership is assigned to each data asset using consent contracts, ensuring data is only shared if the owner consents.
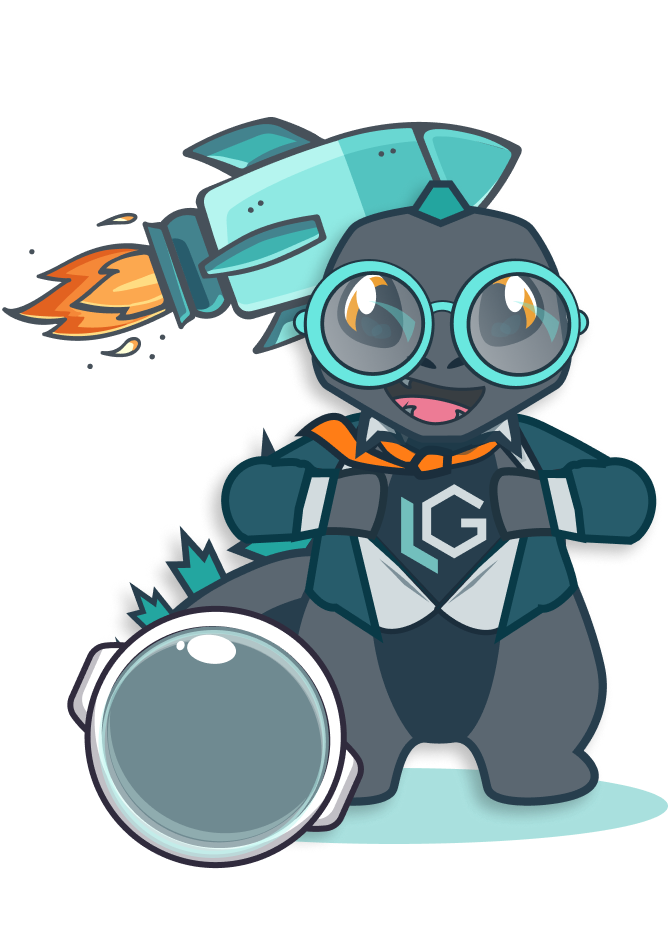
Scalability & Adaptability
Data quality initiatives must scale with the enterprise’s data growth. That is why data quality metrics, standards, and enforcement must be part of the data flow and not implemented as an external solution.
As AI technologies evolve, so will the requirements for data. The initiative should be adaptable and ready to incorporate new data quality metrics, data structures, or standards that future AI models might demand. The only way to do this is by creating data assets vs data stores. We recently wrote about how to make this happen in this Knowledge Burst: The Next Wave: Data Stores to Data Ecosystems & Data Flows.
how LifeGraph helps:
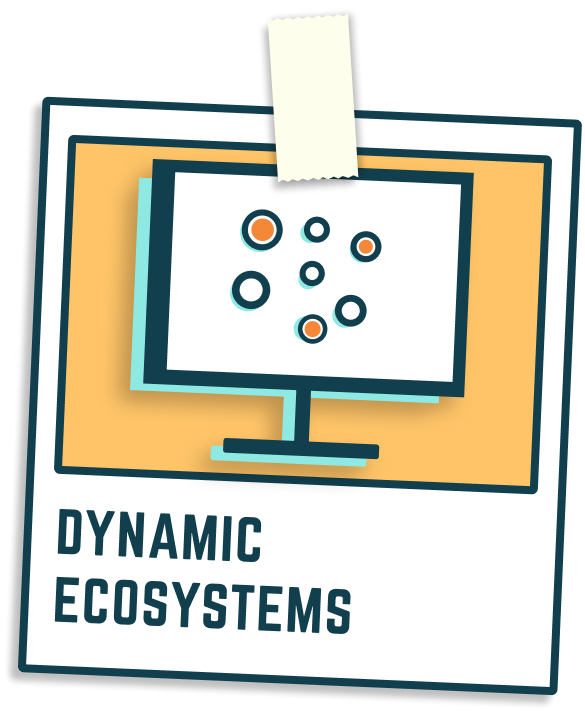
dynamic ecosystems:
LifeGraph empowers dynamic ecosystems capable of incorporating new data sources and evolving as organizational needs change. No need to write new data dictionaries and redeploy.
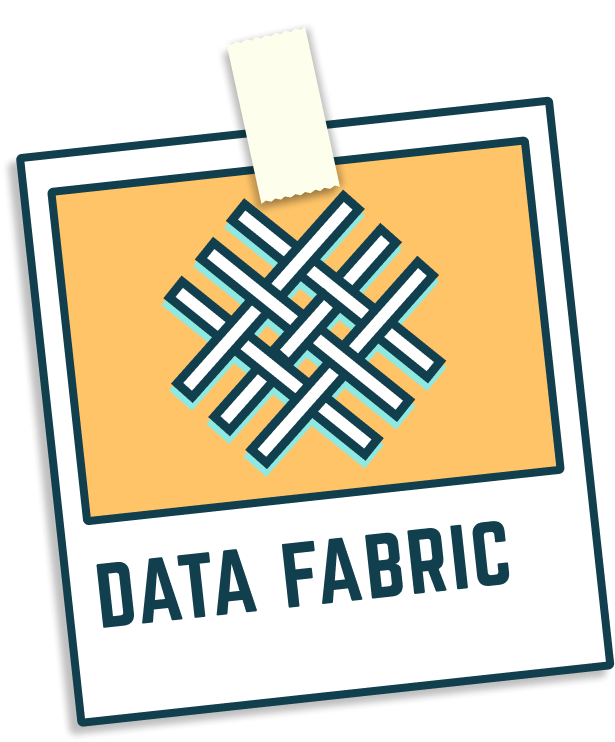
data fabric:
LifeGraph delivers a data fabric, providing a unified view of data across an organization’s ecosystem and ensuring uniform data quality management.
A Successful Quality Adventure
The evolution of data quality management, driven by adaptive APIs and platforms like BurstIQ’s LifeGraph, sets the stage for a new era of data-driven AI. By leveraging Smart Data, LifeGraph enhances data quality and builds trust and context into data assets, making them indispensable for organizations aiming to thrive in the AI-driven future. As we progress, data quality assurance will be a competitive advantage and a necessity for staying relevant in an increasingly data-centric world.
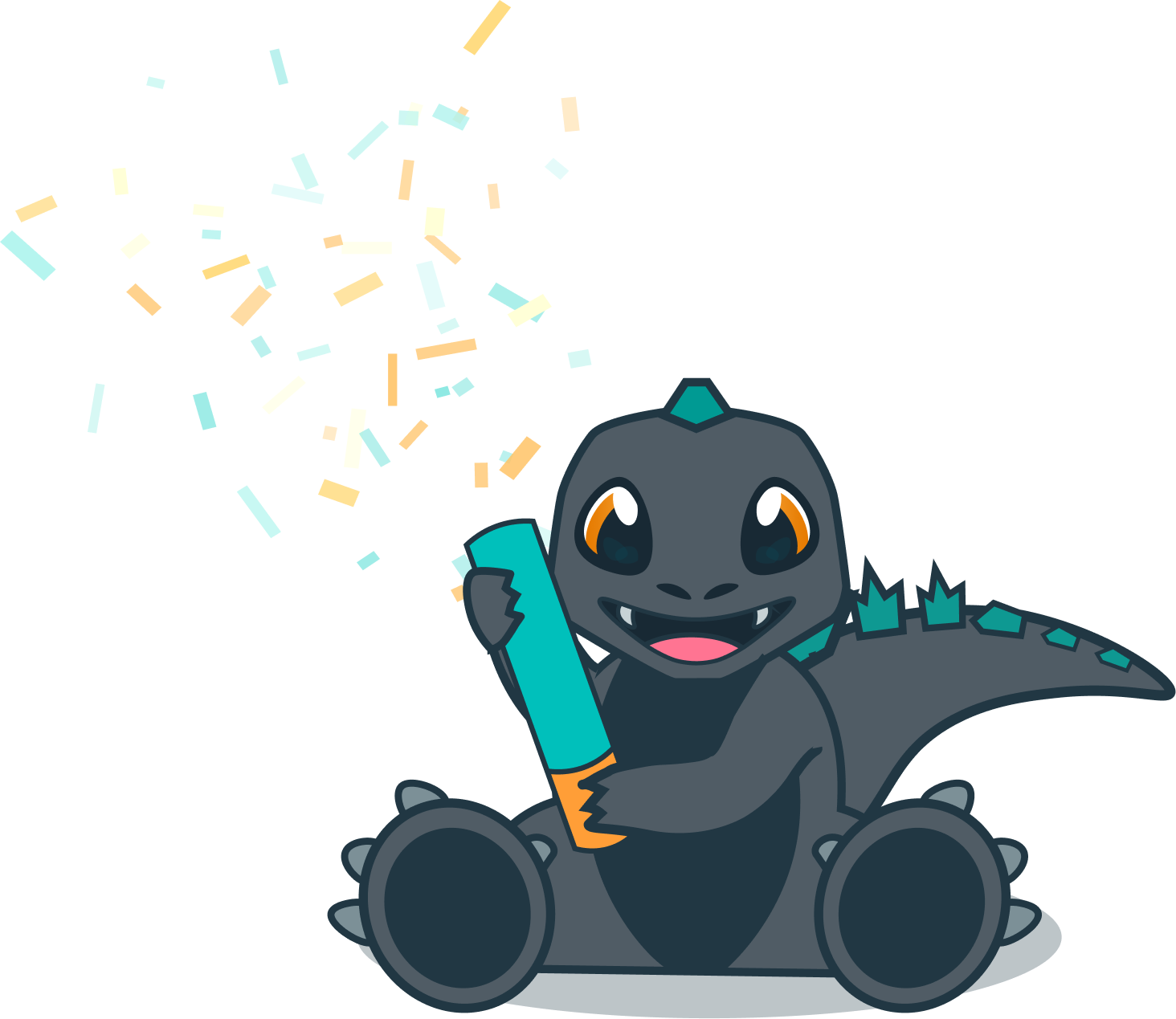