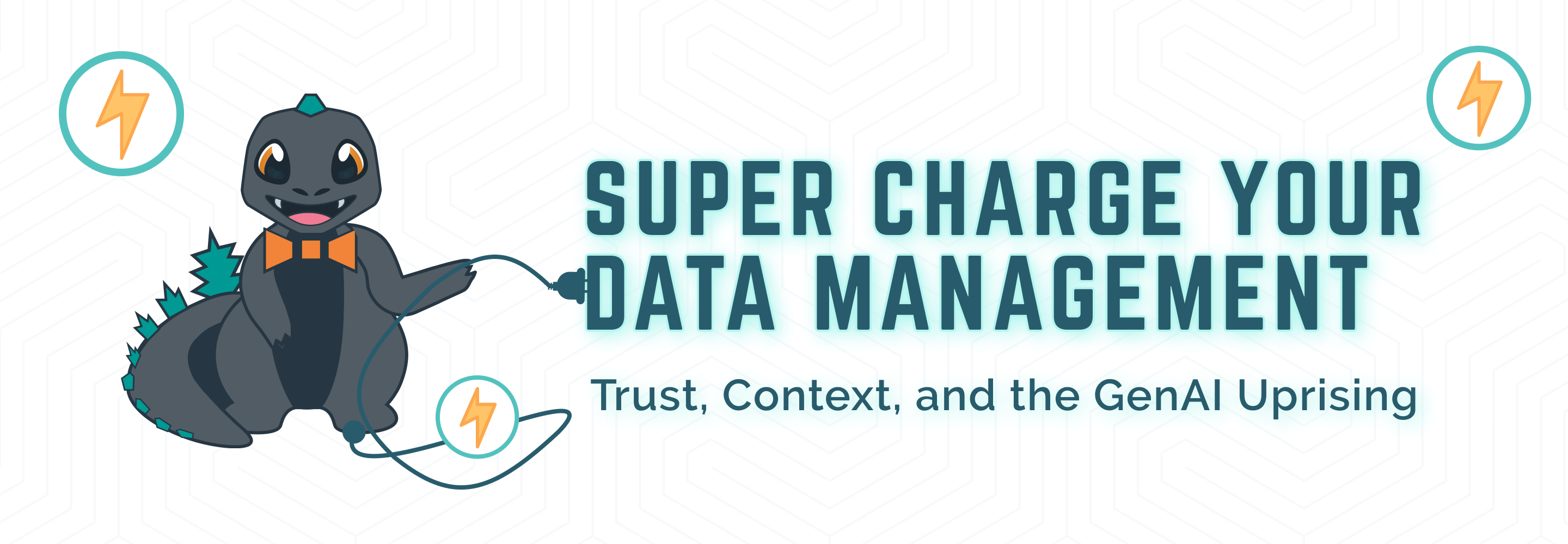
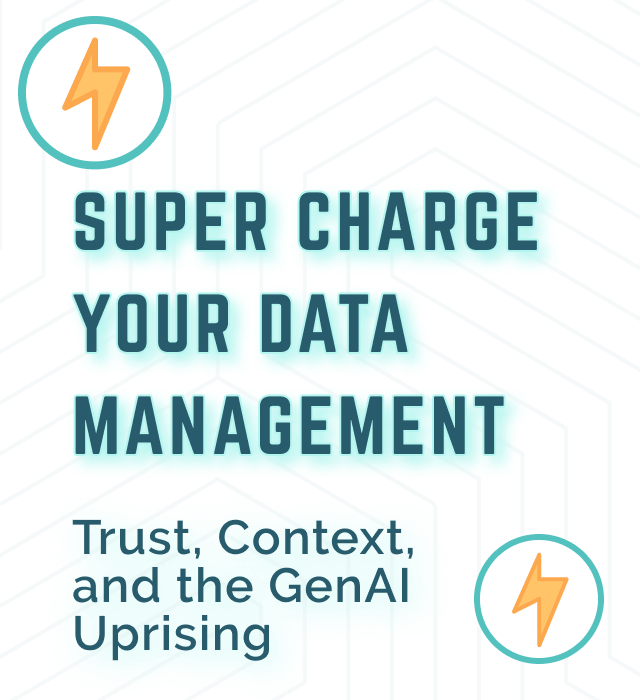
– Frank Ricotta, CEO & Founder, BurstIQ
– Frank Ricotta, CEO & Founder, BurstIQ
– Frank Ricotta, CEO & Founder, BurstIQ
The rapid evolution of large language models, particularly in Generative Artificial Intelligence (GenAI), has sparked a race to execute solutions in all industries. As organizations explore how to incorporate GenAI into their operations, crucial aspects such as trust, context, and protecting proprietary intellectual property emerge as pivotal factors for effective implementation.
The rapid evolution of large language models, particularly in Generative Artificial Intelligence (GenAI), has sparked a race to execute solutions in all industries. As organizations explore how to incorporate GenAI into their operations, crucial aspects such as trust, context, and protecting proprietary intellectual property emerge as pivotal factors for effective implementation.
Bridging the Context Gap
Accuracy and context take center stage in the deployment of GenAI models. The core objective is to achieve results that are not just correct in a technical sense but also perfectly aligned with the specific contextual demands of the business or application in question. AI models may deliver syntactically correct outcomes but lack contextual relevance. This gap challenges organizations relying on these results for critical business decisions.
For example, if I ask ChatGPT about Java, it first defaults to talking about the programming language. However, I was interested in the island of Java. I also wanted some advice on good coffee. Funny note: in real life, I can’t tell you how many resumes we received from baristas when we were looking for senior Java engineers.
Navigating Data Governance and Stewardship
The effective management of GenAI demands robust data governance frameworks that ensure data quality, privacy, and security. You must establish clear protocols for who can access data, how it’s managed, and ensure that its lineage—its origin and how it’s been altered over time—is transparent and traceable. Effective data governance is pivotal in building trust in GenAI applications, ensuring they adhere to regulatory standards and ethical guidelines, safeguarding sensitive information, and maintaining user privacy.
Data Quality and Curation
The axiom “garbage in, garbage out” is particularly pertinent in the realm of GenAI, where the quality of input data directly influences the utility and reliability of generated outcomes. High-quality, well-curated data is the foundation for effective GenAI models. This involves meticulous data preparation, including cleaning, cataloging, and ensuring the data reflects the diversity and complexity of the real world. Large volumes of high-quality data empower GenAI models to generate accurate, reliable, and applicable insights and solutions for the problems they are meant to solve.
Bias
Bias encompasses algorithmic and data biases, which can significantly influence the efficacy of AI systems. Algorithmic bias occurs when AI systems’ underlying algorithms process data to reflect the implicit values of their creators or the societal context in which they developed them. Data bias, on the other hand, arises from the data used to train AI models. Tackling bias requires a concerted effort to scrutinize data sources, data sets, and algorithms.
Amidst the recent scrutiny of Google’s Gemini, bias is no longer a theoretical or subtle aspect; it has become a tangible issue. We encountered several instances of this phenomenon during the initial release of ChatGPT. It’s crucial to recognize that the nature of AI systems is not the result of algorithmic errors or flawed data, which can be swiftly corrected. Instead, it is shaped by the intentions of those who built the systems and trained the models.
The real question is whether it is possible to remove bias, given that people inherently operate from their biased worldview.
Data Privacy and Compliance
Navigating the realm of GenAI models involves technical considerations and a complex web of privacy and legal implications. Training these models with personally identifiable information (PII) runs the risk of violating privacy laws, such as the California Consumer Privacy Act (CCPA) and Section 5 of the Federal Trade Commission Act. The Federal Trade Commission (FTC) has taken a firm stance, mandating companies to delete PII-containing data and the models themselves if privacy commitments are breached.
Intellectual Property Protection
Protecting intellectual property (IP) in AI development is challenging for various reasons, including open-source environments, data privacy concerns, algorithmic transparency needs, the rapid pace of technological advancements, reverse engineering threats, cross-border collaboration complexities, and difficulties in obtaining patents. Organizations must adopt comprehensive strategies to safeguard proprietary AI technologies, combining legal measures such as patents and licensing with technical safeguards like encryption. It is crucial to maintain awareness regarding IP laws and foster compliance to navigate these challenges.
The Power of Knowledge Graphs
Knowledge graphs play a pivotal role in ensuring GenAI’s effectiveness and trustworthiness. Knowledge graphs make LLMs more accurate, transparent, and explainable. Knowledge graphs enable GenAI models to understand and leverage complex, interconnected data by representing information in a graph structure that captures relationships and context. This contextual understanding is crucial for generating accurate and relevant results and aligning the output with the intended business context.
Knowledge graphs also facilitate effective data governance by providing a framework for metadata management, lineage tracking, and access control. In the realm of GenAI, where accurate contextualization is paramount, knowledge graphs serve as a foundation for enhancing the reliability and interpretability of the generated outcomes. Organizations leveraging knowledge graphs with GenAI can bridge the gap between syntactic correctness and contextual relevance, ultimately fostering trust in decision-making and promoting responsible AI practices.
Looking Ahead
We are dealing with GenAI’s rapidly evolving landscape. Organizations must address critical considerations in their data management strategies to manage the changes effectively. Accurate, contextual results, robust governance frameworks, transparency, and high-quality, curated data are essential for successful GenAI integration.
As organizations navigate this landscape, organizations must take a holistic approach that considers the technical aspects and addresses the challenges associated with adoption. By fostering an understanding of the trust and context required for effective GenAI, organizations can make informed decisions in leveraging these advanced technologies to drive innovation and productivity.
About BurstIQ:
LifeGraph® by BurstIQ redefines the potential of organizational data. This next-generation data platform integrates advanced data management, privacy-enhancing technology, and knowledge graphs, transforming data into your organization’s ultimate superpower. Eliminate silos with a single, secure source of truth. LifeGraph reveals hidden connections within complex data sets, aligning with human and machine thinking for easier and more insightful analysis and powerful collaboration.
Organizations use LifeGraph to elevate legacy data lakes and warehouses into dynamic, secure, and person-centric data ecosystems that deliver value to everyone involved. With LifeGraph you can quickly address today’s problems and business initiatives, and ignite the spark of innovation to help your organization not only keep pace but set the tempo for the future.
To learn more about how LifeGraph can help you make data your superpower, please contact us here.
Enhance Your Learning
Check out a couple of our other newest blog posts by clicking the images below.